A Multisystem-Compatible Deep Learning-Based Algorithm for Detection and Characterization of Angiectasias in Small-Bowel Capsule Endoscopy. A Proof-of-concept Study.
Digestive and Liver Disease/Digestive and liver disease(2021)
摘要
Background and aims: Current artificial intelligence (AI)-based solutions for capsule endoscopy (CE) interpretation are proprietary. We aimed to evaluate an AI solution trained on a specific CE system (Pillcam (R), Medtronic) for the detection of angiectasias on images captured by a different proprietary system (MiroCam (R), Intromedic). Material and methods: An advanced AI solution (Axaro (R), Augmented Endoscopy), previously trained on Pillcam (R) small bowell images, was evaluated on independent datasets with more than 1200 Pillcam (R) and MiroCam (R) still frames (equally distributed, with or without angiectasias). Images were reviewed by experts before and after AI interpretation. Results: Sensitivity for the diagnosis of angiectasia was 97.4% with Pillcam (R) images and 96.1% with Mirocam (R) images, with specificity of 98.8% and 97.8%, respectively. Performances regarding the delineation of regions of interest and the characterization of angiectasias were similar in both groups (all above 95%). Processing time was significantly shorter with Mirocam (R) (20.7 ms) than with Pillcam (R) images (24.6 ms, p < 0.0 0 01), possibly related to technical differences between systems. Conclusion: This proof-of-concept study on still images paves the way for the development of resource sparing, "universal" CE databases and AI solutions for CE interpretation. (c) 2021 Editrice Gastroenterologica Italiana S.r.l. Published by Elsevier Ltd. All rights reserved.
更多查看译文
关键词
Artificial intelligence,Capsule endoscopy,Deep learning,Small bowel
AI 理解论文
溯源树
样例
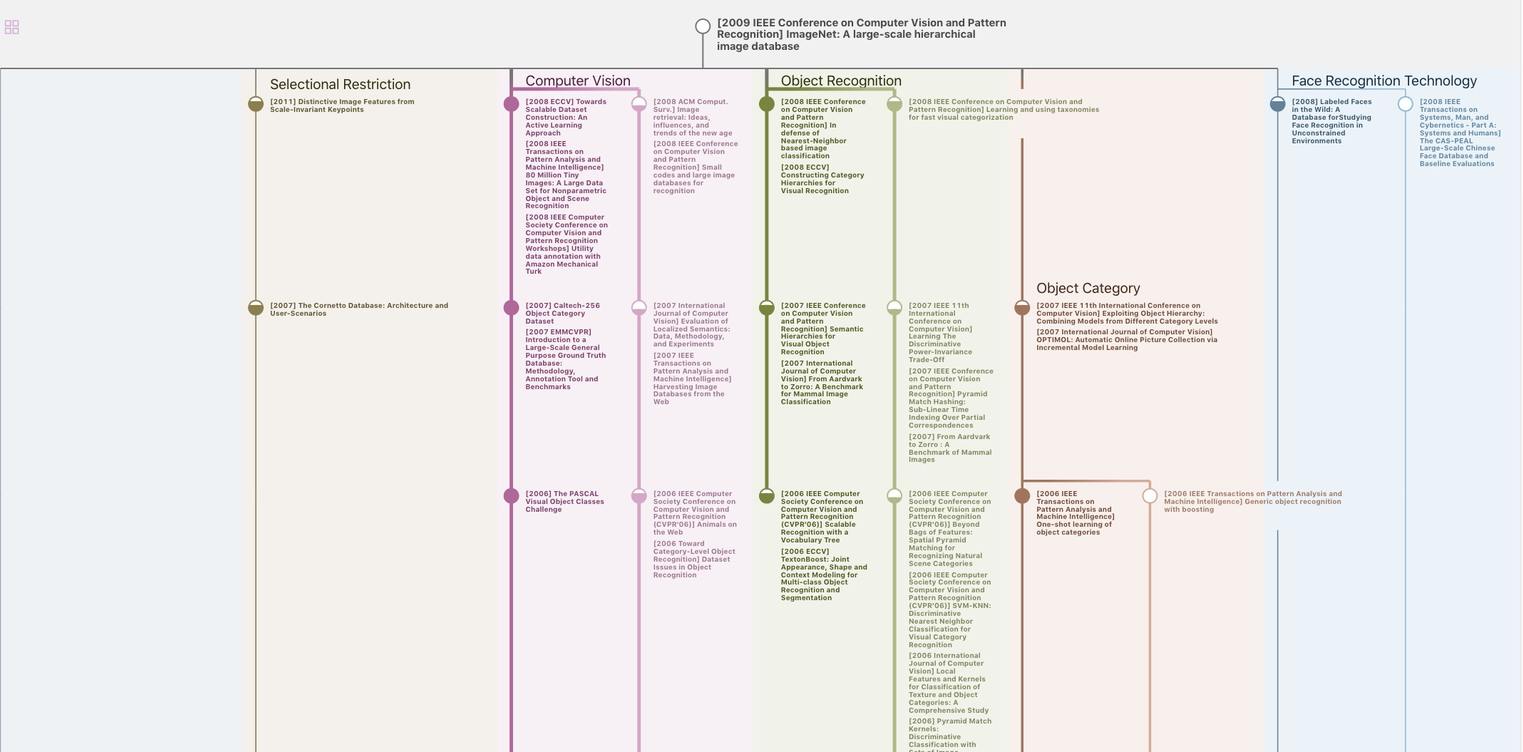
生成溯源树,研究论文发展脉络
Chat Paper
正在生成论文摘要