Attention-based dynamic user preference modeling and nonlinear feature interaction learning for collaborative filtering recommendation
APPLIED SOFT COMPUTING(2021)
摘要
The traditional collaborative filtering (CF) method based on static user preference modeling and linear matching function learning severely limits the recommendation performance. To solve the above problem, in this article, we adopt dynamic user preference modeling and nonlinear matching function learning in the CF recommendation. For dynamic user preference modeling, a two-layer neural attention network is used, which fully considers the predicted item, the recent historical interacted items and their interaction time to estimate the contribution weight of each interacted item in user preferences modeling. For nonlinear matching function learning, we add a single hidden layer neural network on top of the traditional matrix factorization (MF) model, which can significantly improve the feature interaction learning capabilities of the model with only a few additional parameters. Extensive experiments show that our method significantly outperforms the state-of-the-art CF methods and the key technologies we proposed in this research have a positive effect on improving the recommendation performance. (C) 2021 Elsevier B.V. All rights reserved.
更多查看译文
关键词
Collaborative filtering, Neural attention network, Dynamic user preference modeling, Matching function learning
AI 理解论文
溯源树
样例
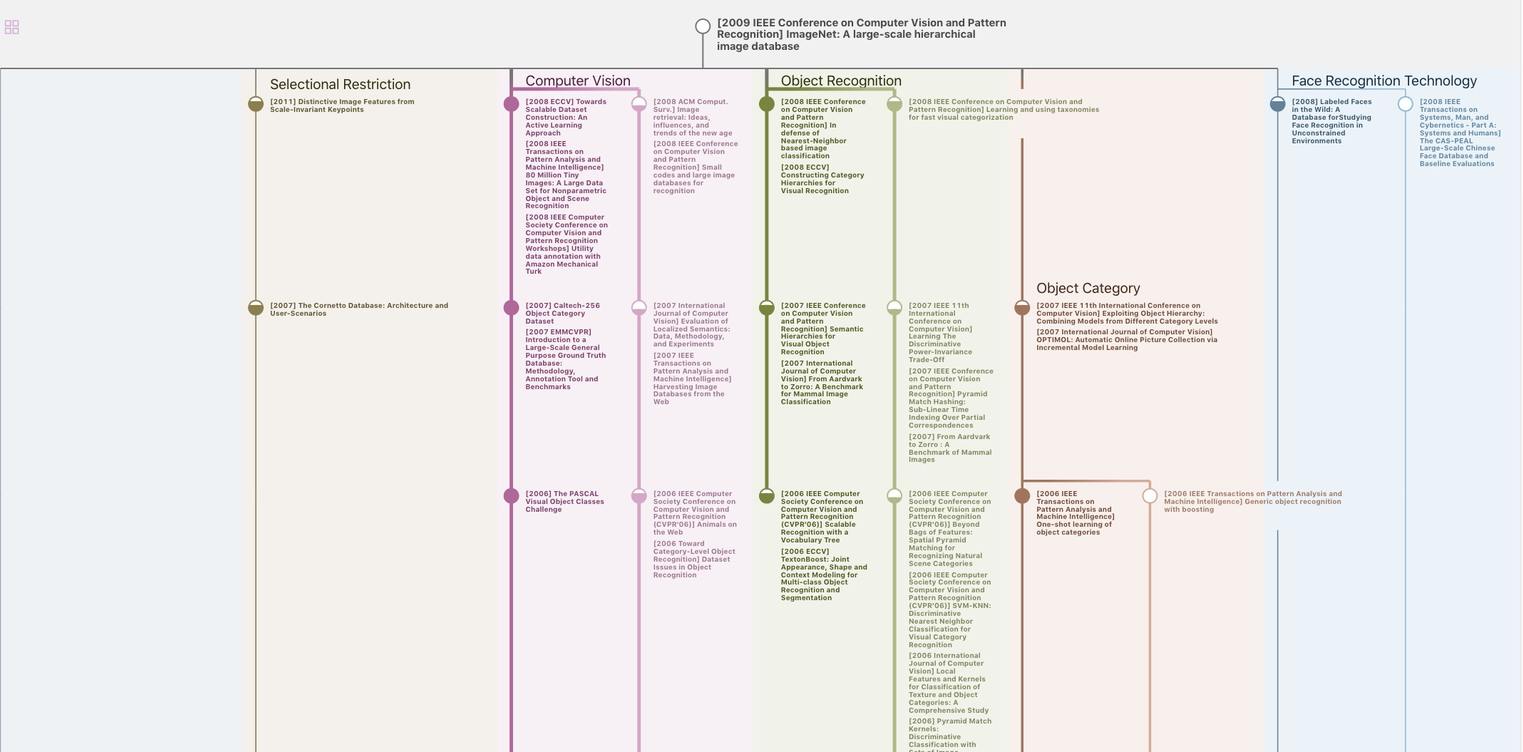
生成溯源树,研究论文发展脉络
Chat Paper
正在生成论文摘要