Future Network Traffic Matrix Synthesis and Estimation Based on Deep Generative Models
2021 International Conference on Computer Communications and Networks (ICCCN)(2021)
Abstract
Traffic matrices (TMs) contain information that is essential for network management, traffic engineering, and anomaly detection. However, constructing a TM through direct traffic measurements has a high administrative and computational cost. A more feasible approach is to estimate the TM from the easily obtainable link load measurements. In this paper, we address the issue of traffic matrix estimation (TME) from link loads using a deep generative model - namely, a variational autoencoder (VAE) - to solve the respective ill-posed inverse problem. In particular, we train the VAE with historical data (previously observed TMs) and we leverage the trained decoder to transform TME into a minimization problem in the latent space, which in turn can be solved by employing a gradient-based optimizer. Furthermore, the trained decoder can be used for traffic matrix synthesis, i.e., for generating synthetic TM examples that have "similar" properties to the samples of the training set. Finally, we explore the incremental optimization of the sequence of objectives constructed from the sequence of decoders that we obtain at different stages of the VAE training. The performance of the proposed methods is evaluated using a publicly available dataset of actual traffic matrices recorded in a real backbone network.
MoreTranslated text
Key words
Network monitoring,network tomography,traffic matrix estimation,deep learning,variational autoencoder
AI Read Science
Must-Reading Tree
Example
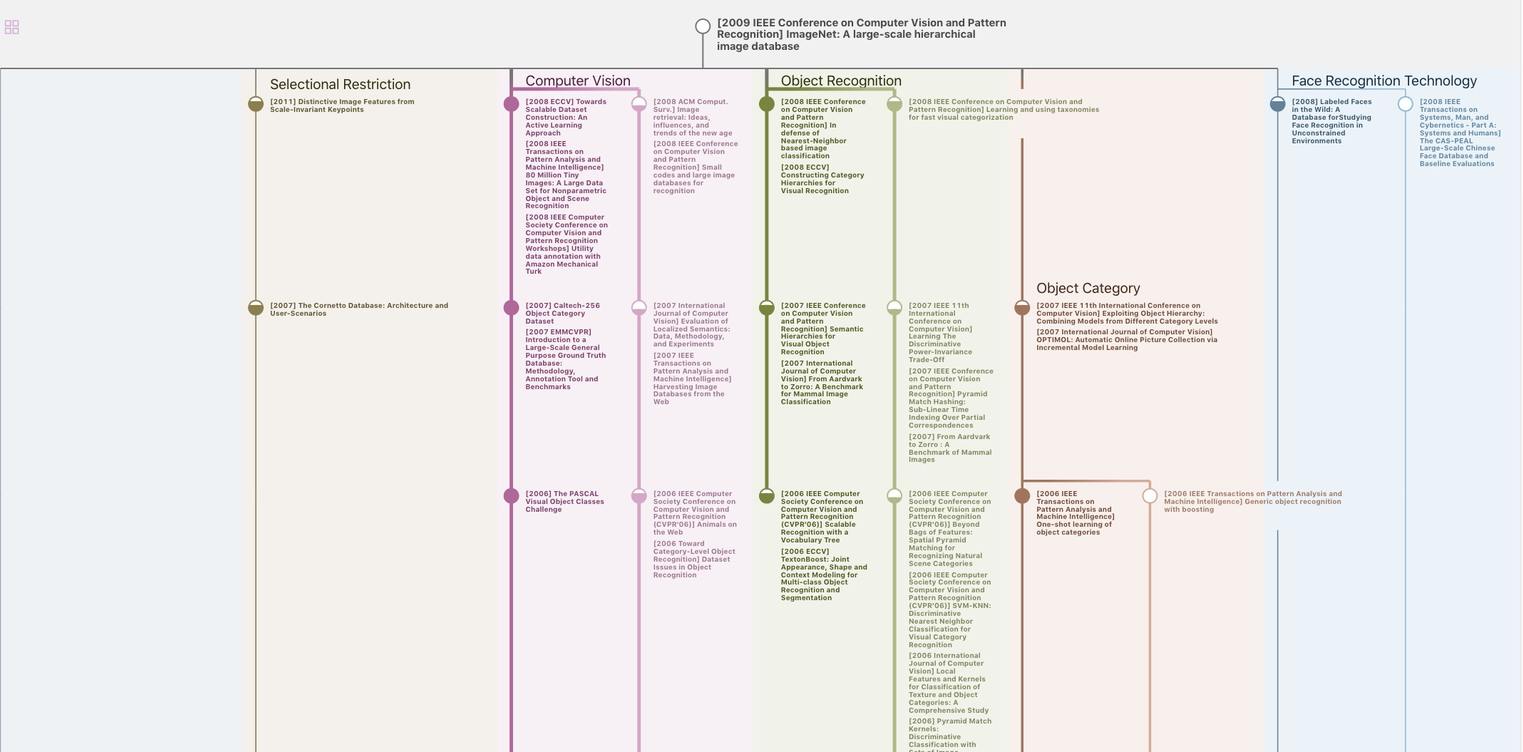
Generate MRT to find the research sequence of this paper
Chat Paper
Summary is being generated by the instructions you defined