Using Deep Reinforcement Learning for Routing in IP Networks
30TH INTERNATIONAL CONFERENCE ON COMPUTER COMMUNICATIONS AND NETWORKS (ICCCN 2021)(2021)
摘要
This paper proposes Trailnet, a deep reinforcement learning approach to predict the output port (of a router) for an IP packet based on its destination IP address. Trailnet attempts to replace the forwarding table at an IP router with a computational model. To optimally learn each router's forwarding decisions, we propose to train the Artificial Neural Network (ANN) of Trailnet with value iteration and stochastic gradient descent. Through the value iteration algorithm, Trailnet estimates the cost of IP packet forwarding along different ports of a router and eventually selects a port that optimizes a cost function. We evaluate the generalization capability of the ANN on two sufficiently large service provider's network topologies containing millions of IP addresses. Our evaluations show that Trailnet achieves high accuracy and fast inference time for predicting the correct output ports for incoming IP packets. Our results support the claim of replacing forwarding tables and distributed protocols (for computing shortest paths) with a computation model while operating at a high line rate in IP routers.
更多查看译文
关键词
Deep reinforcement learning,value iteration,stochastic gradient descent,artificial neural network,output port prediction
AI 理解论文
溯源树
样例
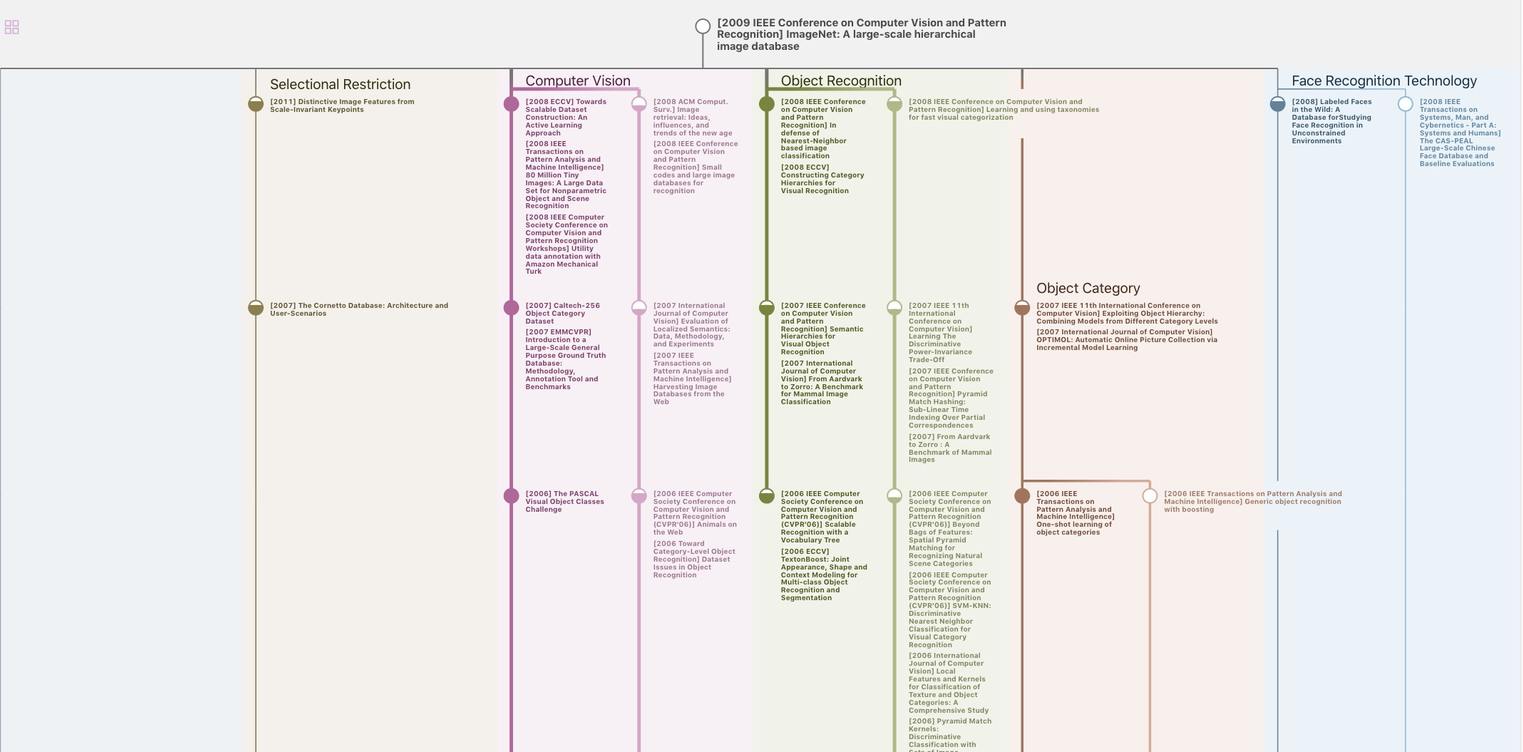
生成溯源树,研究论文发展脉络
Chat Paper
正在生成论文摘要