DRAN: Detailed Region-Adaptive Normalization for Conditional Image Synthesis
IEEE TRANSACTIONS ON MULTIMEDIA(2024)
摘要
In recent years, conditional image synthesis has attracted growing attention due to its controllability in the image generation process. Although recent works have achieved realistic results, most of them have difficulty handling fine-grained styles with subtle details. To address this problem, a novel normalization module, named Detailed Region-Adaptive Normalization (DRAN), is proposed. It adaptively learns both fine-grained and coarse-grained style representations. Specifically, we first introduce a multi-level structure, Spatiality-aware Pyramid Pooling, to guide the model to learn coarse-to-fine features. Then, to adaptively fuse different levels of styles, we propose Dynamic Gating, making it possible to adaptively fuse different levels of styles according to different spatial regions. Finally, we collect a new makeup dataset (Makeup-Complex dataset) that contains a wide range of complex makeup styles with diverse poses and expressions. To evaluate the effectiveness and show the general use of our method, we conduct a set of experiments on makeup transfer and semantic image synthesis. Quantitative and qualitative experiments show that equipped with DRAN, simple baseline models are able to achieve promising improvements in complex style transfer and detailed texture synthesis.
更多查看译文
关键词
Conditional image synthesis,makeup transfer,semantic image synthesis,generative adversarial network
AI 理解论文
溯源树
样例
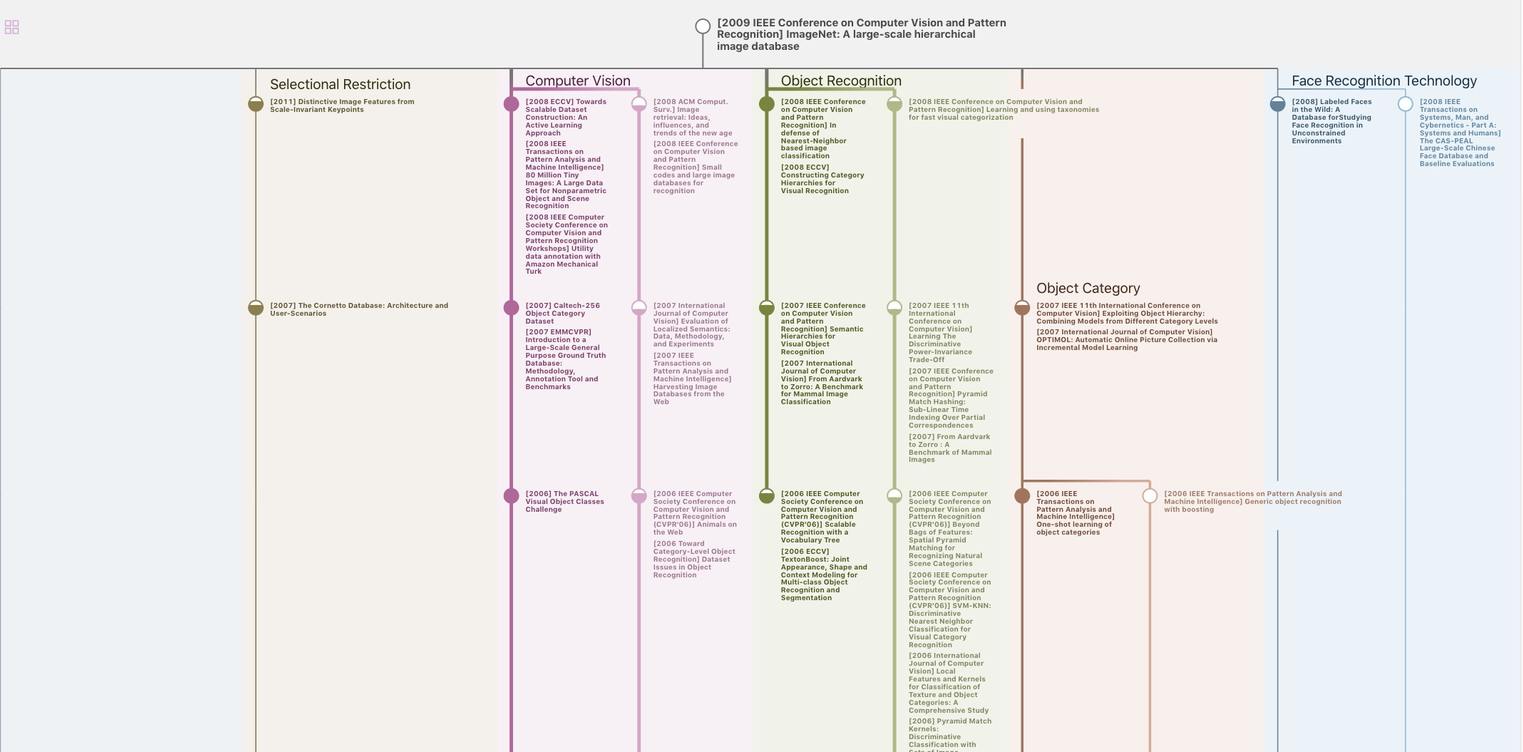
生成溯源树,研究论文发展脉络
Chat Paper
正在生成论文摘要