Deep Learning for Improving Non-Destructive Grain Mapping in 3D
IUCrJ(2021)
摘要
Laboratory X-ray diffraction contrast tomography (LabDCT) is a novel imaging technique for non-destructive 3D characterization of grain structures. An accurate grain reconstruction critically relies on precise segmentation of diffraction spots in the LabDCT images. The conventional method utilizing various filters generally satisfies segmentation of sharp spots in the images, thereby serving as a standard routine, but it also very often leads to over or under segmentation of spots, especially those with low signal-to-noise ratios and/or small sizes. The standard routine also requires a fine tuning of the filtering parameters. To overcome these challenges, a deep learning neural network is presented to efficiently and accurately clean the background noise, thereby easing the spot segmentation. The deep learning network is first trained with input images, synthesized using a forward simulation model for LabDCT in combination with a generic approach to extract features of experimental backgrounds. Then, the network is applied to remove the background noise from experimental images measured under different geometrical conditions for different samples. Comparisons of both processed images and grain reconstructions show that the deep learning method outperforms the standard routine, demonstrating significantly better grain mapping.
更多查看译文
关键词
grain mapping,X-ray diffraction,tomography,deep learning,computer vision,background noise,spot segmentation,LabDCT
AI 理解论文
溯源树
样例
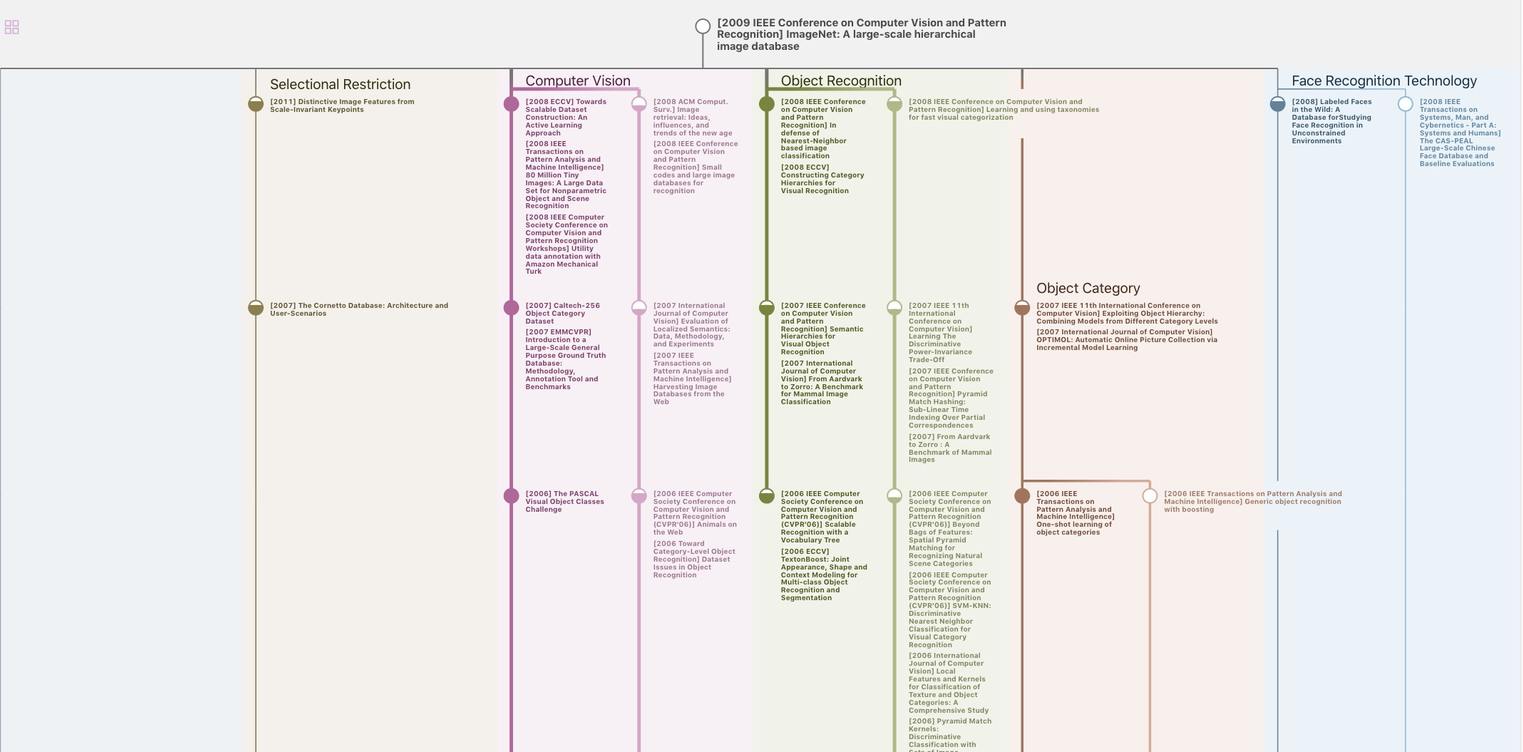
生成溯源树,研究论文发展脉络
Chat Paper
正在生成论文摘要