Synergising Stoichiometric Modelling With Artificial Neural Networks To Predict Antibody Glycosylation Patterns In Chinese Hamster Ovary Cells
COMPUTERS & CHEMICAL ENGINEERING(2021)
摘要
In-process quality control of biotherapeutics, such as monoclonal antibodies, requires computationally ef-ficient process models that use readily measured process variables to compute product quality. Existing kinetic cell culture models can effectively describe the underlying mechanisms but require considerable development and parameterisation effort. Stoichiometric models, on the other hand, provide a generic, parameter-free means for describing metabolic behaviour but do not extend to product quality predic-tion. We have overcome this limitation by integrating a stoichiometric model of Chinese hamster ovary (CHO) cell metabolism with an artificial neural network that uses the fluxes of nucleotide sugar donor synthesis to compute the profile of Fc N-glycosylation, a critical quality attribute of antibody therapeu-tics. We demonstrate that this hybrid framework accurately computes glycan distribution profiles using a set of easy-to-obtain experimental data, thus providing a platform for process control applications. (c) 2021 Elsevier Ltd. All rights reserved.
更多查看译文
关键词
Constraint-based modelling, Flux balance analysis, Artificial neural network, Monoclonal antibody, Protein glycosylation, Hybrid modelling
AI 理解论文
溯源树
样例
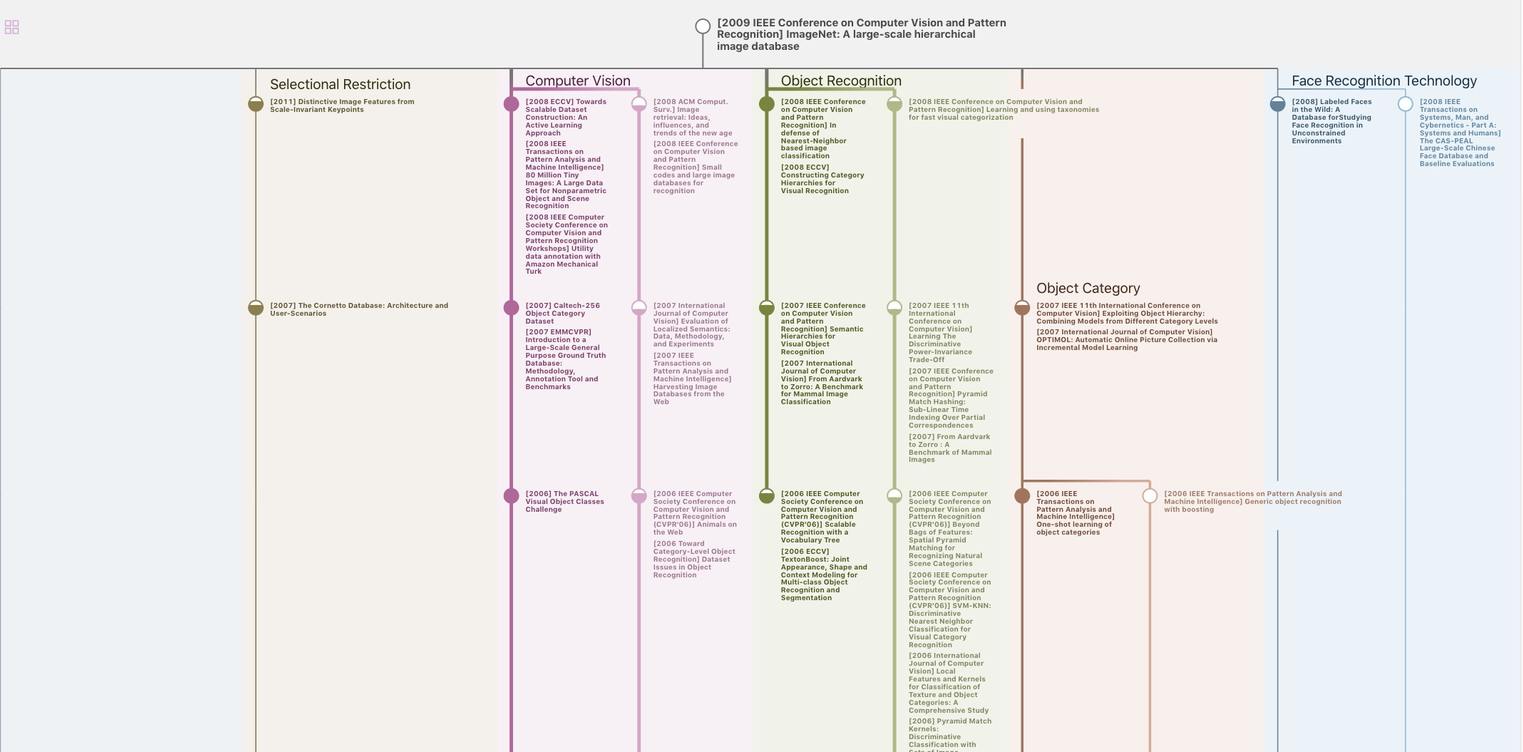
生成溯源树,研究论文发展脉络
Chat Paper
正在生成论文摘要