Obstacle Detection And Recognition In Farmland Based On Fusion Point Cloud Data
COMPUTERS AND ELECTRONICS IN AGRICULTURE(2021)
摘要
Obstacle detection and recognition in the farmland environment is of great significance to unmanned agricultural machinery navigation technology. In this study, a tractor platform based on 3D/2D LiDAR and GNSS/AHRS was built to acquire fusion point cloud data. A complete data processing flow is proposed, including fusion point cloud data acquisition, obstacle detection, and obstacle recognition. The fusing point cloud data acquisition method realized the time synchronization between multiple sensors and the space registration of point cloud at different coordinate systems. The obstacle detection method removed ground, sparse, and ego platform points based on RANSAC and crop-box filtering. Then, a Euclidean clustering method based on the platform width was used for obstacle clustering. The obstacle recognition method established a box model based on PCA for each cluster and extracted its geometric feature information. Next, a MCB classification method is proposed to distinguish false-positive, non-interest, and trackable obstacles (e.g., tractor and pedestrian). To evaluate the results of data collection and processing, a series of experiments was designed and implemented. The average absolute deviation of the fusion point cloud was 0.015 m, and the average rate of lost was 1.24%. The proportion of residual ground points in the ground point cloud was less than 0.012%. The accuracy, precision, recall, and F1score exceeded 80%. Therefore, the proposed method could meet the operation requirements in the farmland environment and provide highly precise and stable detection and recognition results.
更多查看译文
关键词
LiDAR, Fusion point cloud, Obstacle detection, Obstacle recognition, Multi-condition-branch classification
AI 理解论文
溯源树
样例
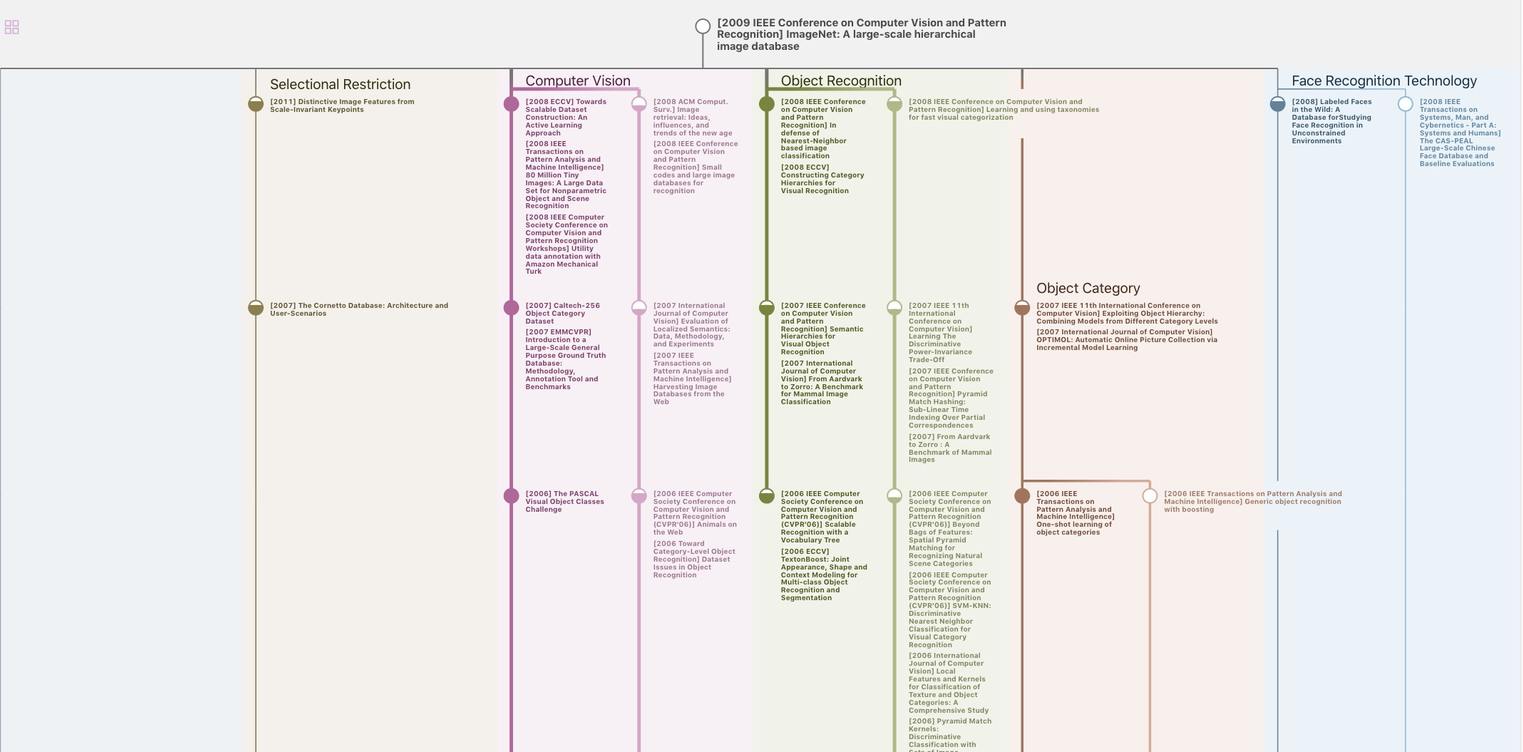
生成溯源树,研究论文发展脉络
Chat Paper
正在生成论文摘要