Generalised Hierarchical Bayesian Microstructure Modelling for Diffusion MRI
COMPUTATIONAL DIFFUSION MRI, CDMRI 2021(2021)
摘要
Microstructure imaging combines tailored diffusion MRI acquisition protocols with a mathematical model to give insights into subvoxel tissue features. The model is typically fit voxel-by-voxel to the MRI image with least squares minimisation to give voxelwise maps of parameters relating to microstructural features, such as diffusivities and tissue compartment fractions. However, this fitting approach is susceptible to voxelwise noise, which can lead to erroneous values in parameter maps. Data-driven Bayesian hierarchical modelling defines prior distributions on parameters and learns them from the data, and can hence reduce such noise effects. Bayesian hierarchical modelling has been demonstrated for microstructure imaging with diffusion MRI, but only for a few, relatively simple, models. In this paper, we generalise hierarchical Bayesian modelling to a wide range of multi-compartment microstructural models, and fit the models with a Markov chain Monte Carlo (MCMC) algorithm. We implement our method by utilising Dmipy, a microstructure modelling software package for diffusion MRI data. Our code is available at github.com/PaddySlator/dmipy-bayesian.
更多查看译文
关键词
Bayesian statistics, Bayesian hierarchical model, Microstructure modelling, Diffusion MRI
AI 理解论文
溯源树
样例
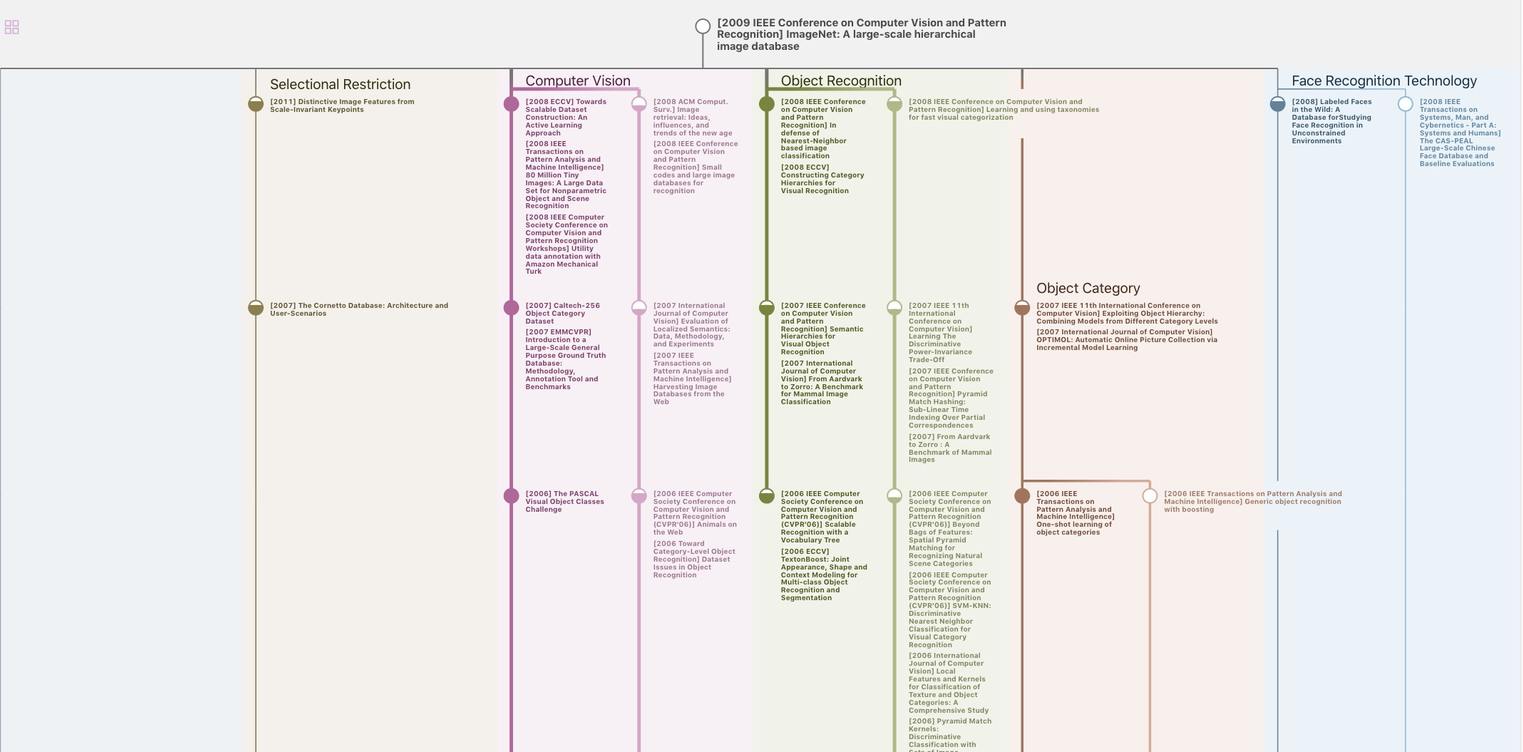
生成溯源树,研究论文发展脉络
Chat Paper
正在生成论文摘要