A Multi-attribute Controllable Generative Model for Histopathology Image Synthesis
MEDICAL IMAGE COMPUTING AND COMPUTER ASSISTED INTERVENTION - MICCAI 2021, PT VIII(2021)
摘要
Generative models have been applied in the medical imaging domain for various image recognition and synthesis tasks. However, a more controllable and interpretable image synthesis model is still lacking yet necessary for important applications such as assisting in medical training. In this work, we leverage the efficient self-attention and contrastive learning modules and build upon state-of-the-art generative adversarial networks (GANs) to achieve an attribute-aware image synthesis model, termed AttributeGAN, which can generate high-quality histopathology images based on multi-attribute inputs. In comparison to existing single-attribute conditional generative models, our proposed model better reflects input attributes and enables smoother interpolation among attribute values. We conduct experiments on a histopathology dataset containing stained H&E images of urothelial carcinoma and demonstrate the effectiveness of our proposed model via comprehensive quantitative and qualitative comparisons with state-of-the-art models as well as different variants of our model.
更多查看译文
关键词
Histopathology image synthesis, Attribute-aware conditional generative model, Conditional contrastive learning
AI 理解论文
溯源树
样例
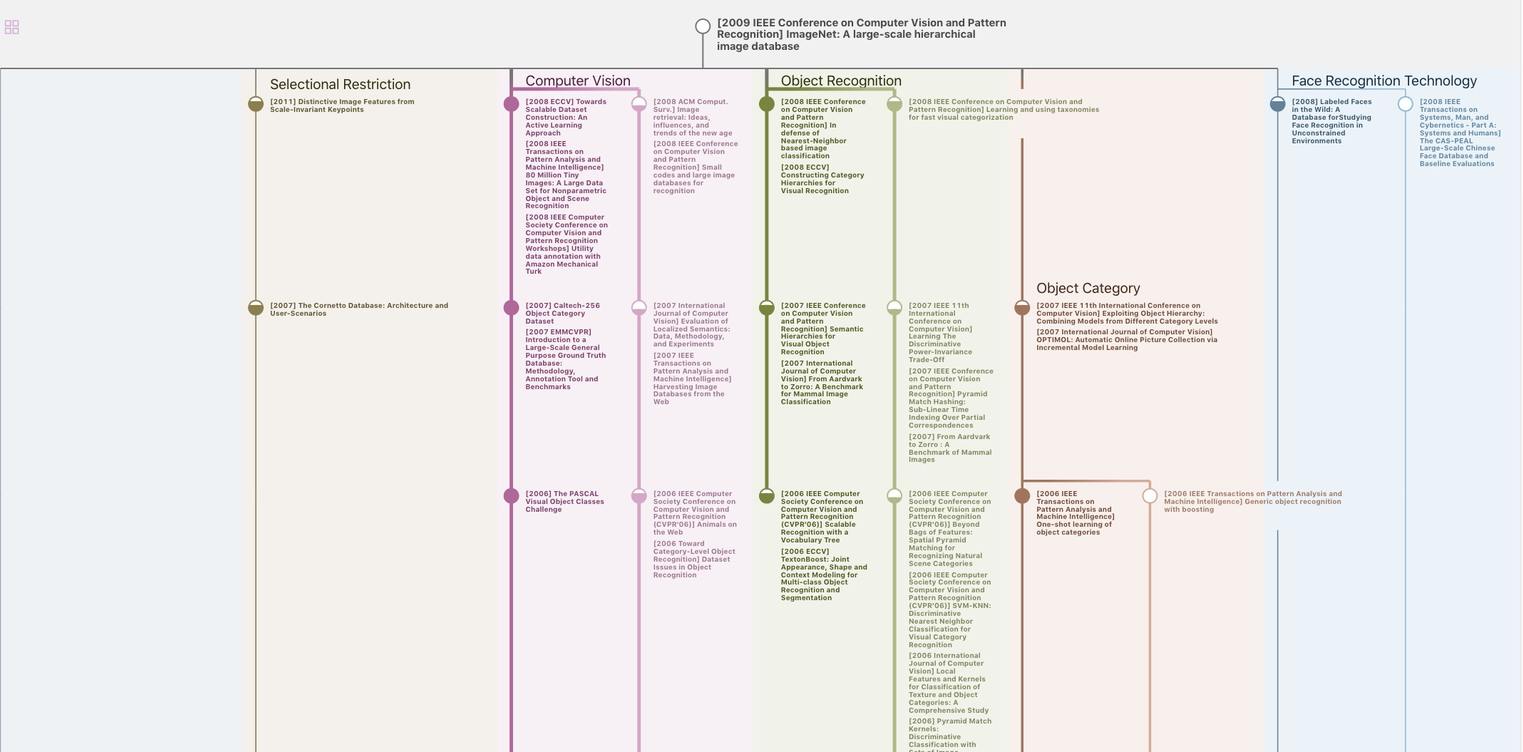
生成溯源树,研究论文发展脉络
Chat Paper
正在生成论文摘要