Phase-Independent Latent Representation for Cardiac Shape Analysis
MEDICAL IMAGE COMPUTING AND COMPUTER ASSISTED INTERVENTION - MICCAI 2021, PT VI(2021)
摘要
Atrial fibrillation (AF) is a complex cardiac disease impacting an ever-growing population and increases 6-fold the risk of thrombus formation. However, image based bio-markers to predict thrombosis in presence of AF are not well known. This lack of knowledge comes from the difficulty to analyse and compare the shape of the Left Atrium (LA) as well as the insufficiency of data that limits the complexity of models we can use. Conducting data analysis in cardiology exacerbates the small dataset problem because the heart cycle renders impossible to compare images taken at systole and diastole time. To address these issues, we first propose a graph representation of the LA, to focus on the impact of pulmonary veins (PV) and LA Appendage (LAA) positions, giving a simple object easy to analyse. Secondly, we propose a meta-learning framework for heterogeneous datasets based on the consistent representation of each dataset in a common latent space. We show that such a model is analogous to a meta-classifier, where each dataset is characterised by specific projection in a common latent space, while sharing the same separating boundary. We apply this model to the graph representation of the LA and interpret the model to give novel time-dependant bio-markers related to PV and LAA configurations for the prediction of thrombosis.
更多查看译文
关键词
Shape analysis, Atrial fibrillation, Thrombosis, Graph representation, Latent space model, Multi-task learning, Meta-learning
AI 理解论文
溯源树
样例
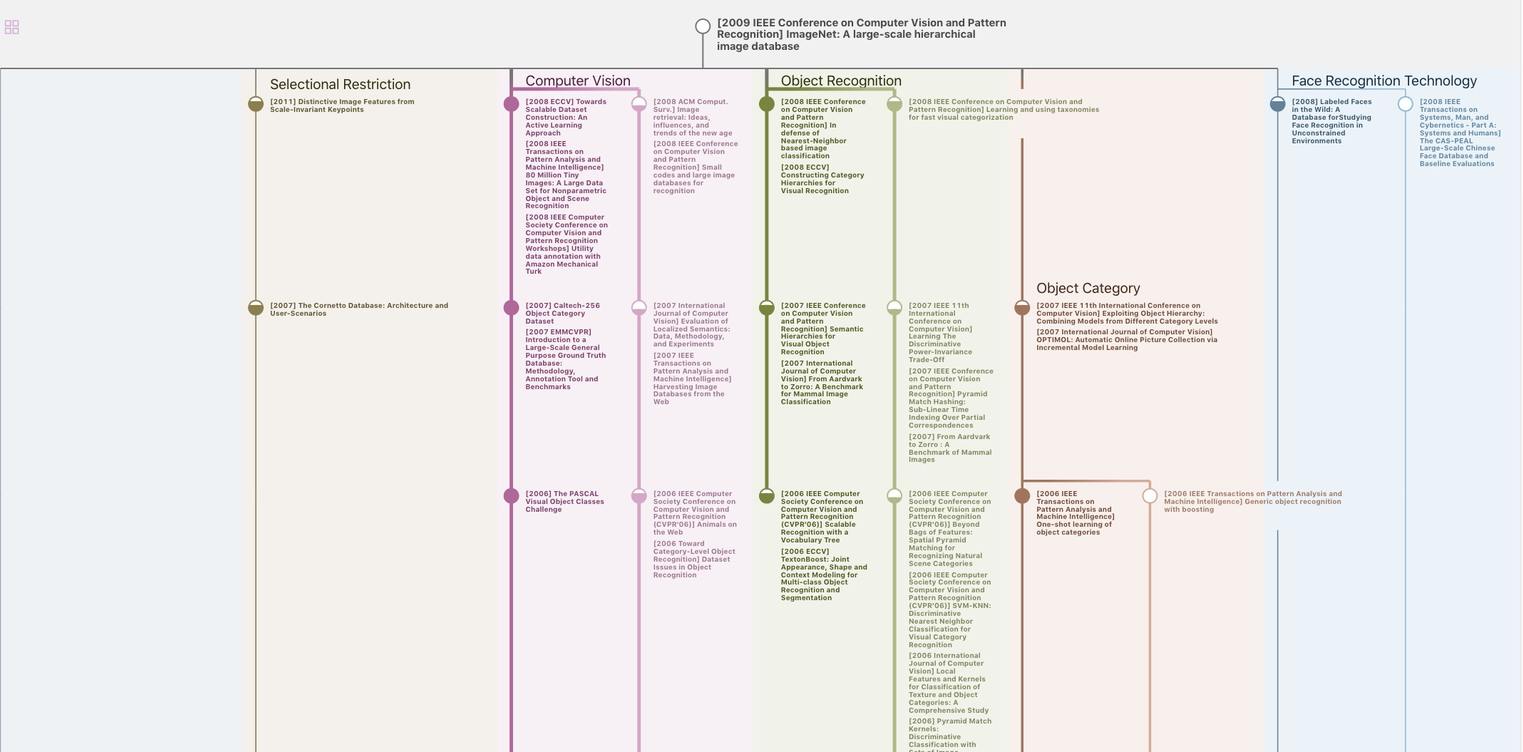
生成溯源树,研究论文发展脉络
Chat Paper
正在生成论文摘要