Interactive Smoothing Parameter Optimization in DBT Reconstruction Using Deep Learning
MEDICAL IMAGE COMPUTING AND COMPUTER ASSISTED INTERVENTION - MICCAI 2021, PT VII(2021)
摘要
Medical image reconstruction algorithms such as Penalized Weighted Least Squares (PWLS) typically rely on a good choice of tuning parameters such as the number of iterations, the strength of regularizar, etc. However, obtaining a good estimate of such parameters is often done using trial and error methods. This process is very time consuming and laborious especially for high resolution images. To solve this problem we propose an interactive framework. We focus on the regularization parameter and train a CNN to imitate its impact on image for varying values. The trained CNN can be used by a human practitioner to tune the regularization strength on-the-fly as per the requirements. Taking the example of Digital Breast Tomosynthesis reconstruction, we demonstrate the feasibility of our approach and also discuss the future applications of this interactive reconstruction approach. We also test the proposed methodology on public Walnut and Lodopab CT reconstruction datasets to show it can be generalized to CT reconstruction as well.
更多查看译文
关键词
CNN, Breast Tomosynthesis reconstruction, Deep Learning
AI 理解论文
溯源树
样例
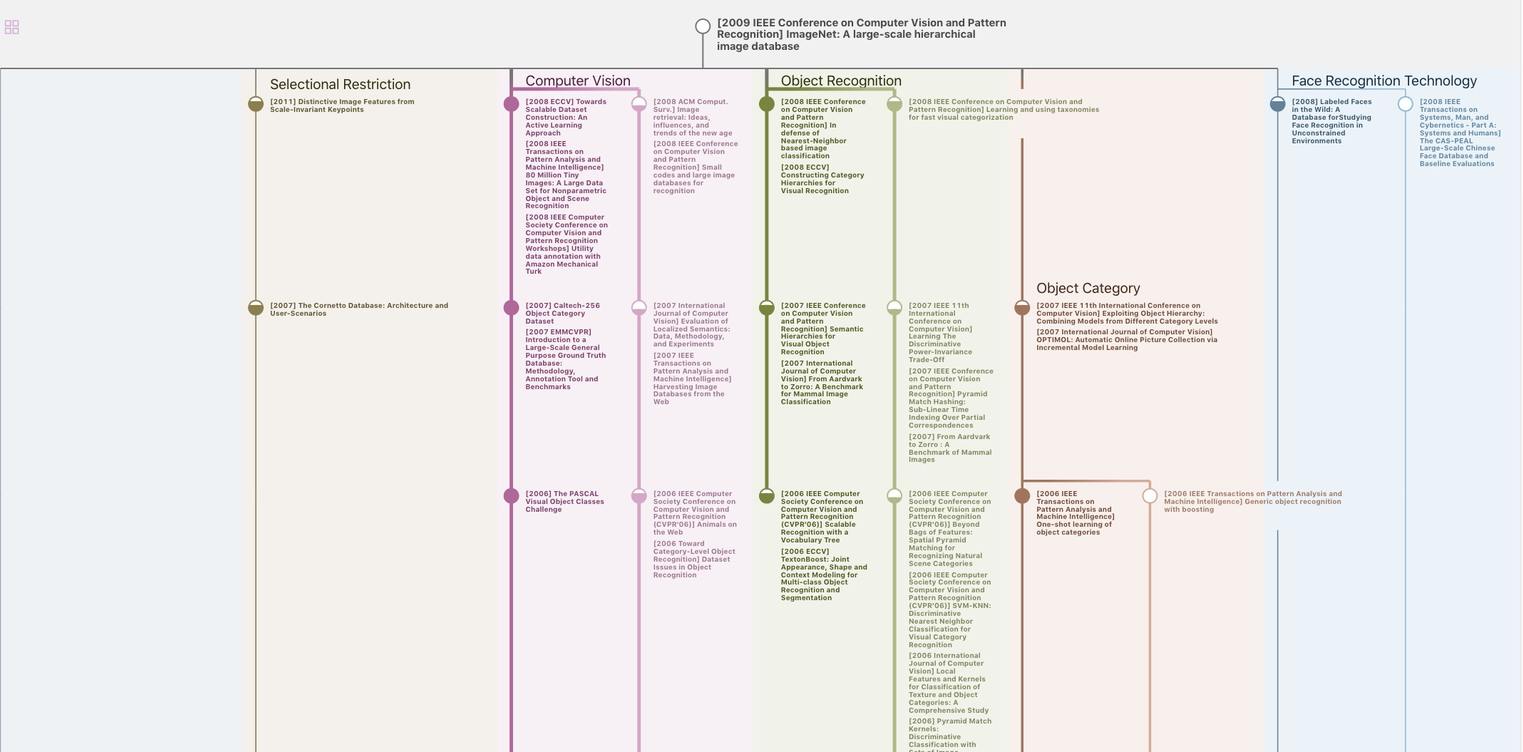
生成溯源树,研究论文发展脉络
Chat Paper
正在生成论文摘要