OXnet: Deep Omni-Supervised Thoracic Disease Detection from Chest X-Rays
MEDICAL IMAGE COMPUTING AND COMPUTER ASSISTED INTERVENTION - MICCAI 2021, PT II(2021)
摘要
Chest X-ray (CXR) is the most typical diagnostic X-ray examination for screening various thoracic diseases. Automatically localizing lesions from CXR is promising for alleviating radiologists' reading burden. However, CXR datasets are often with massive image-level annotations and scarce lesion-level annotations, and more often, without annotations. Thus far, unifying different supervision granularities to develop thoracic disease detection algorithms has not been comprehensively addressed. In this paper, we present OXnet, the first deep omni-supervised thoracic disease detection network to our best knowledge that uses as much available supervision as possible for CXR diagnosis. We first introduce supervised learning via a one-stage detection model. Then, we inject a global classification head to the detection model and propose dual attention alignment to guide the global gradient to the local detection branch, which enables learning lesion detection from image-level annotations. We also impose intra-class compactness and inter-class separability with global prototype alignment to further enhance the global information learning. Moreover, we leverage a soft focal loss to distill the soft pseudo-labels of unlabeled data generated by a teacher model. Extensive experiments on a large-scale chest X-ray dataset show the proposed OXnet outperforms competitive methods with significant margins. Further, we investigate omni-supervision under various annotation granularities and corroborate OXnet is a promising choice to mitigate the plight of annotation shortage for medical image diagnosis (Code is available at https://github.com/LLYXC/OXnet.).
更多查看译文
关键词
Omni-supervised learning, Chest X-ray, Disease localization, Attention
AI 理解论文
溯源树
样例
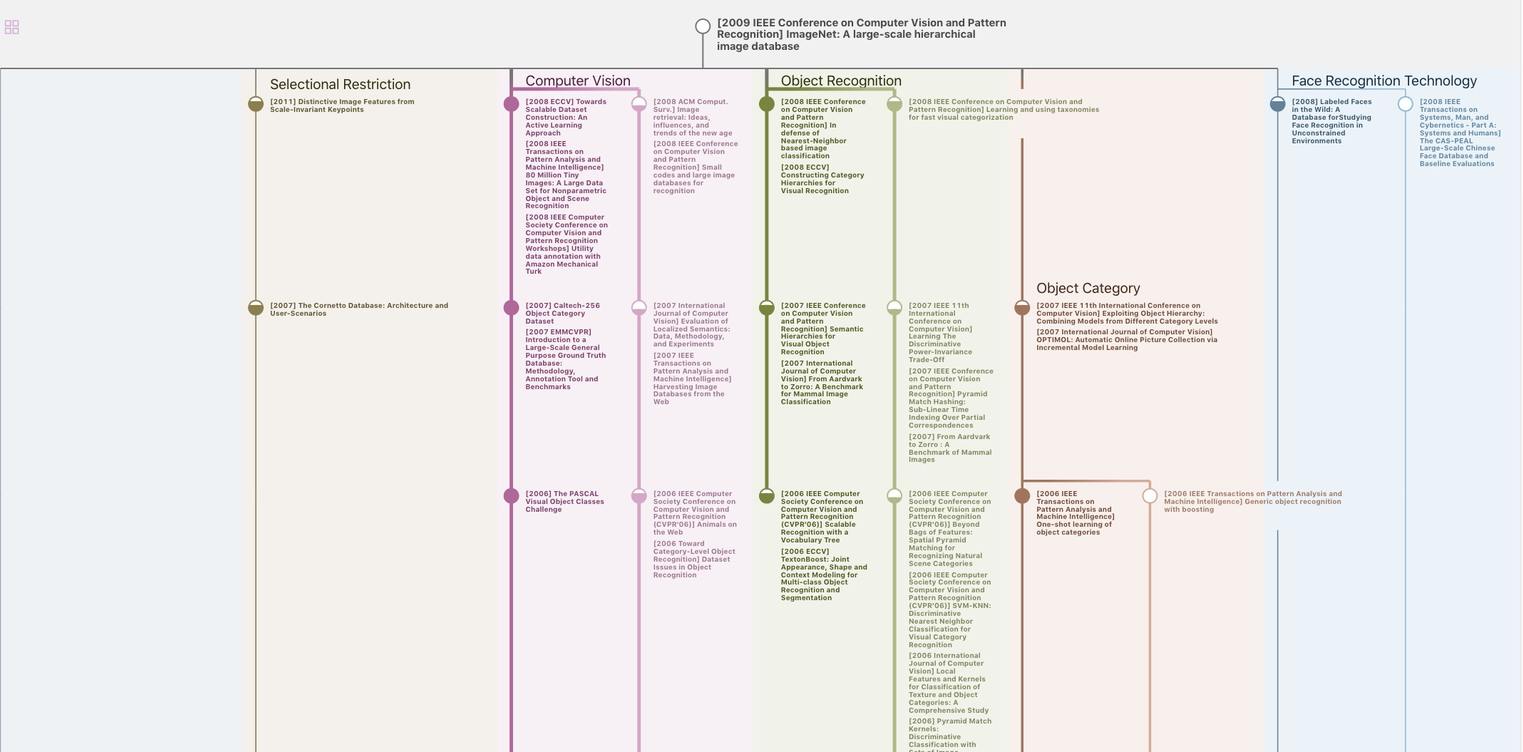
生成溯源树,研究论文发展脉络
Chat Paper
正在生成论文摘要