Uncertainty-aware Mean Teacher for Source-free Unsupervised Domain Adaptive 3D Object Detection
arxiv(2021)
摘要
Pseudo-label based self training approaches are a popular method for source-free unsupervised domain adaptation. However, their efficacy depends on the quality of the labels generated by the source trained model. These labels may be incorrect with high confidence, rendering thresholding methods ineffective. In order to avoid reinforcing errors caused by label noise, we propose an uncertainty-aware mean teacher framework which implicitly filters incorrect pseudo-labels during training. Leveraging model uncertainty allows the mean teacher network to perform implicit filtering by down-weighing losses corresponding uncertain pseudo-labels. Effectively, we perform automatic soft-sampling of pseudo-labeled data while aligning predictions from the student and teacher networks. We demonstrate our method on several domain adaptation scenarios, from cross-dataset to cross-weather conditions, and achieve state-of-the-art performance in these cases, on the KITTI lidar target dataset.
更多查看译文
关键词
detection,3d,teacher,uncertainty-aware,source-free
AI 理解论文
溯源树
样例
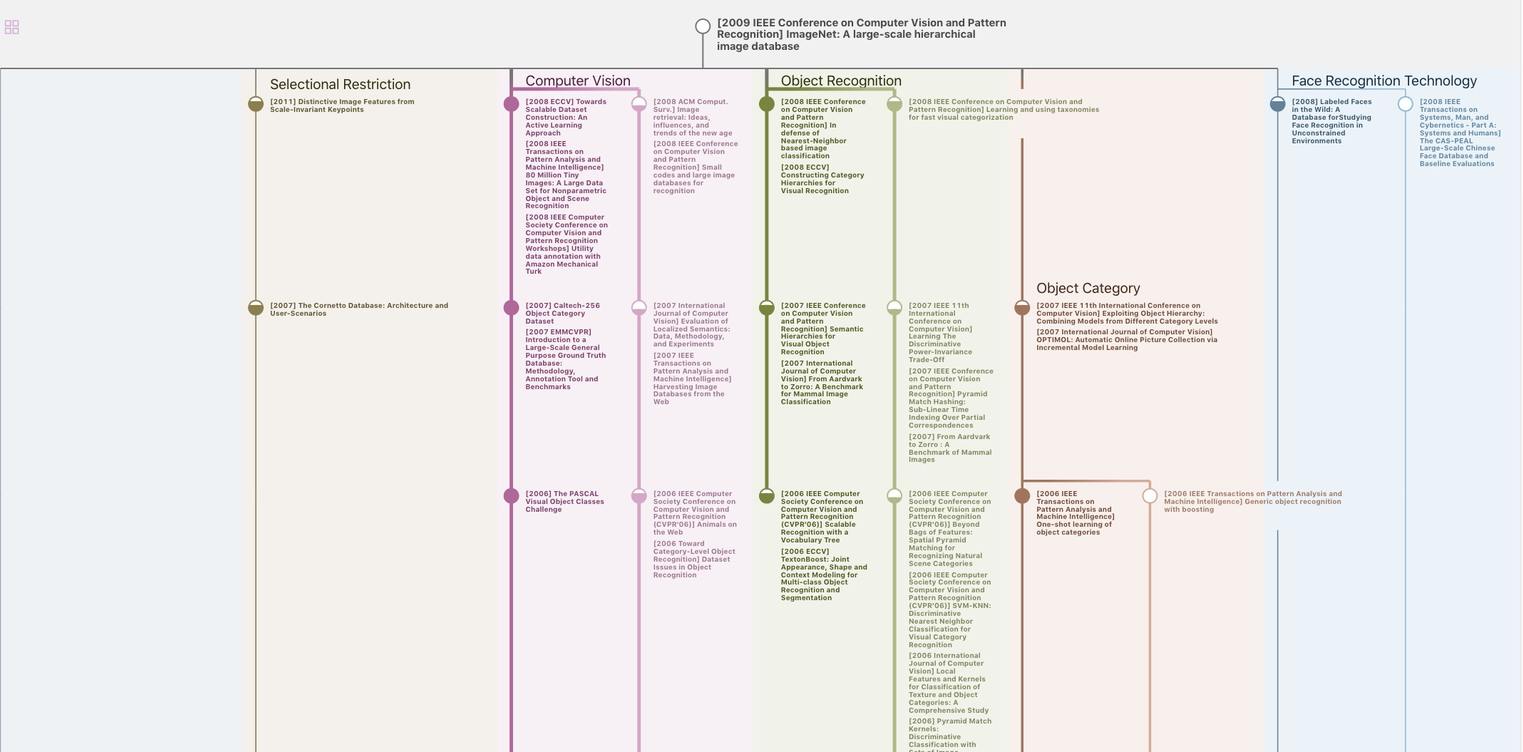
生成溯源树,研究论文发展脉络
Chat Paper
正在生成论文摘要