Forming a sparse representation for visual place recognition using a neurorobotic approach.
ITSC(2021)
摘要
This paper introduces a novel unsupervised neural network model for visual information encoding which aims to address the problem of large-scale visual localization. Inspired by the structure of the visual cortex, the model (namely HSD) alternates layers of topologic sparse coding and pooling to build a more compact code of visual information. Intended for visual place recognition (VPR) systems that use local descriptors, the impact of its integration in a bio-inpired model for self-localization (LPMP) is evaluated. Our experimental results on the KITTI dataset show that HSD improves the runtime speed of LPMP by a factor of at least 2 and its localization accuracy by 10%. A comparison with CoHog, a state-of-the-art VPR approach, showed that our method achieves slightly better results.
更多查看译文
关键词
visual place recognition,place recognition,sparse representation,neurorobotic approach
AI 理解论文
溯源树
样例
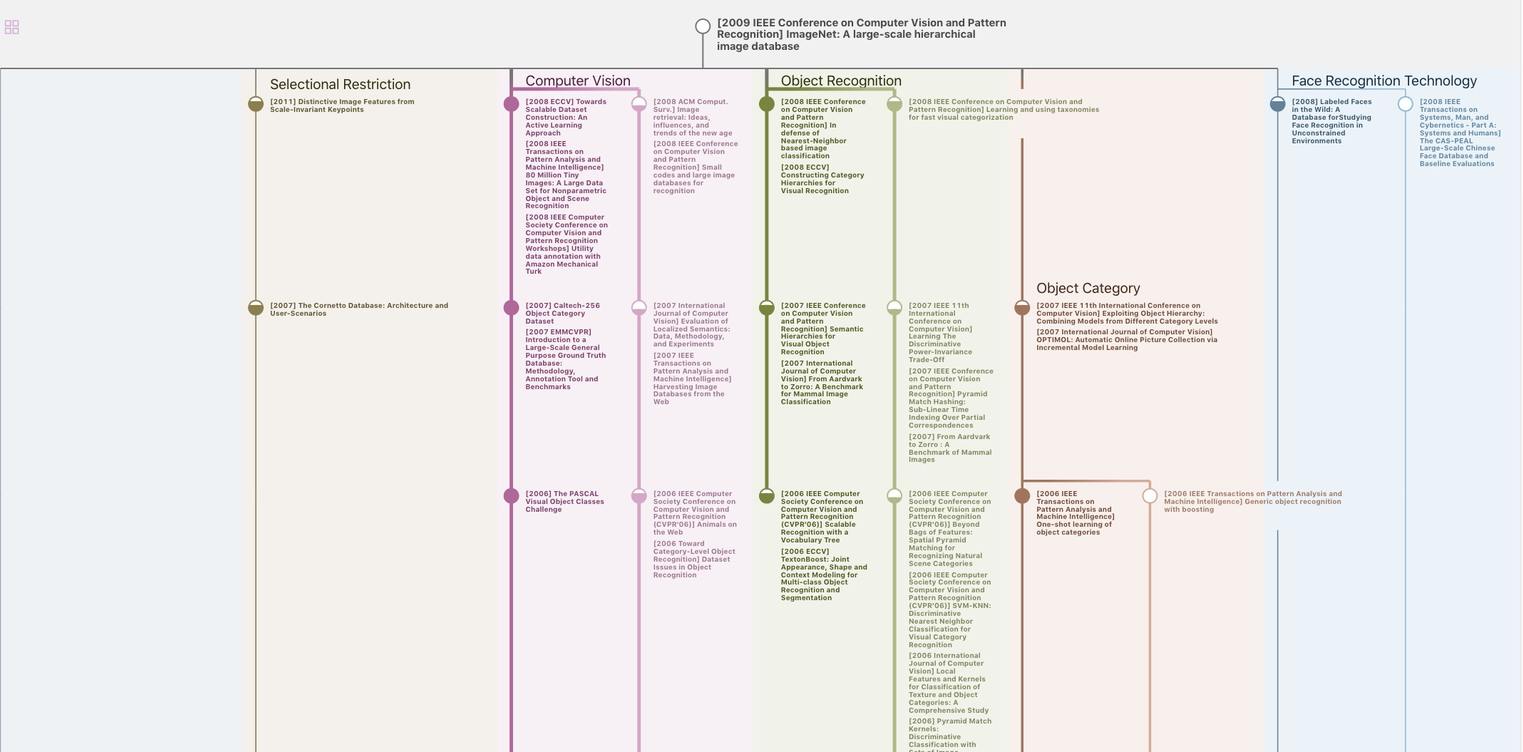
生成溯源树,研究论文发展脉络
Chat Paper
正在生成论文摘要