On fast convergence rates for generalized conditional gradient methods with backtracking stepsize
NUMERICAL ALGEBRA CONTROL AND OPTIMIZATION(2024)
摘要
A generalized conditional gradient method for minimizing the sum of two convex functions, one of them differentiable, is presented. This iterative method relies on two main ingredients: First, the minimization of a partially linearized objective functional to compute a descent direction and, second, a stepsize choice based on an Armijo-like condition to ensure sufficient descent in every iteration. We provide several convergence results. Under mild assumptions, the method generates sequences of iterates which converge, on subsequences, towards minimizers. Moreover, a sublinear rate of convergence for the objective functional values is derived. Second, we show that the method enjoys improved rates of convergence if the partially linearized problem fulfills certain growth estimates. Most notably these results do not require strong convexity of the objective functional. Numerical tests on a variety of challenging PDE-constrained optimization problems confirm the practical efficiency of the proposed algorithm.
更多查看译文
关键词
Optimization methods,generalized conditional gradient,nonsmooth optimization
AI 理解论文
溯源树
样例
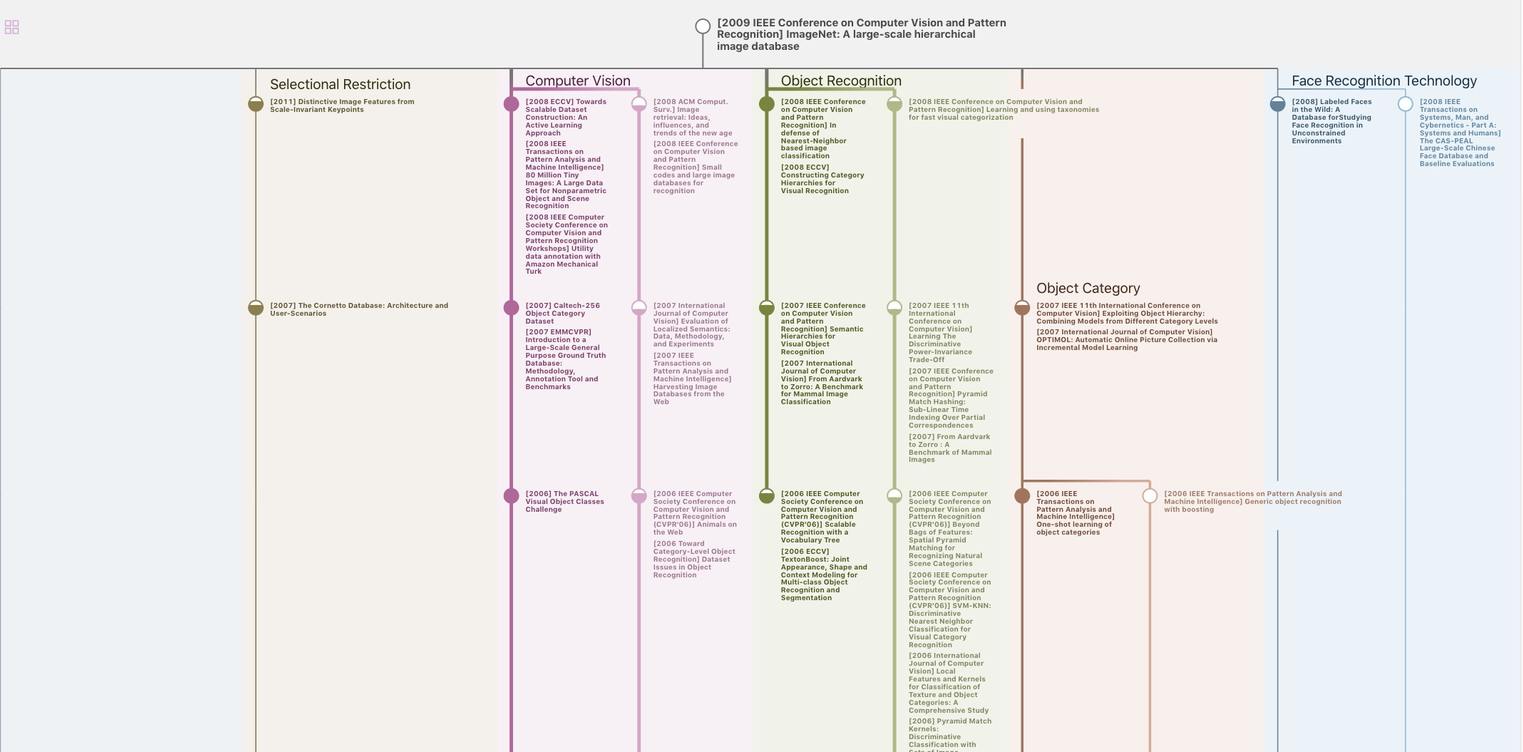
生成溯源树,研究论文发展脉络
Chat Paper
正在生成论文摘要