Combining max-pooling and wavelet pooling strategies for semantic image segmentation
Expert Systems with Applications(2021)
摘要
This paper presents a novel multi-pooling architecture generated by combining the advantages of wavelet and max-pooling operations in convolutional neural networks (CNNs), focusing on semantic segmentation tasks. CNNs often use pooling to reduce the number of parameters, improve invariance to certain distortions, and enlarge the receptive field. However, pooling can cause information loss and thus is detrimental to further operations such as feature extraction and analysis. This problem is particularly critical for semantic segmentation, where each pixel of an image is assigned to a specific class to divide the image into disjoint regions of interest. To address this problem, pooling strategies based on wavelets-operations have been proposed with the promise to achieve a better trade-off between receptive field size and computational efficiency. Previous works have confirmed the superiority of wavelet pooling over the traditional one in semantic segmentation tasks. However, we have observed in our computational experiments that the expressive gains reported from the use of wavelet pooling in other segmentation tasks were not observed in the scope of aerial imagery due to imprecision in the segmentation of image details. The combination of wavelet pooling and max-pooling, a solution not yet reported in the literature, can address that issue. Such gap observed in the pooling area motivated the two proposals that are the main contributions of this paper: (a) A new multi-pooling strategy combining wavelet and traditional pooling in a new network structure suitable for aerial image segmentation tasks; (b) Two-stream architectures using the traditional max-pooling and wavelet pooling as streams. These proposals were implemented using the Segnet, a known architecture for semantic segmentation. The computational experiments, based on the IRRG images from the Potsdam and Vaihingen data sets, demonstrated that the proposed architectures surpassed the original Segnet architecture’s performance with results comparable to state-of-the-art approaches.
更多查看译文
关键词
Convolutional neural networks,Semantic segmentation,Max pooling,Wavelet pooling,IRRG images
AI 理解论文
溯源树
样例
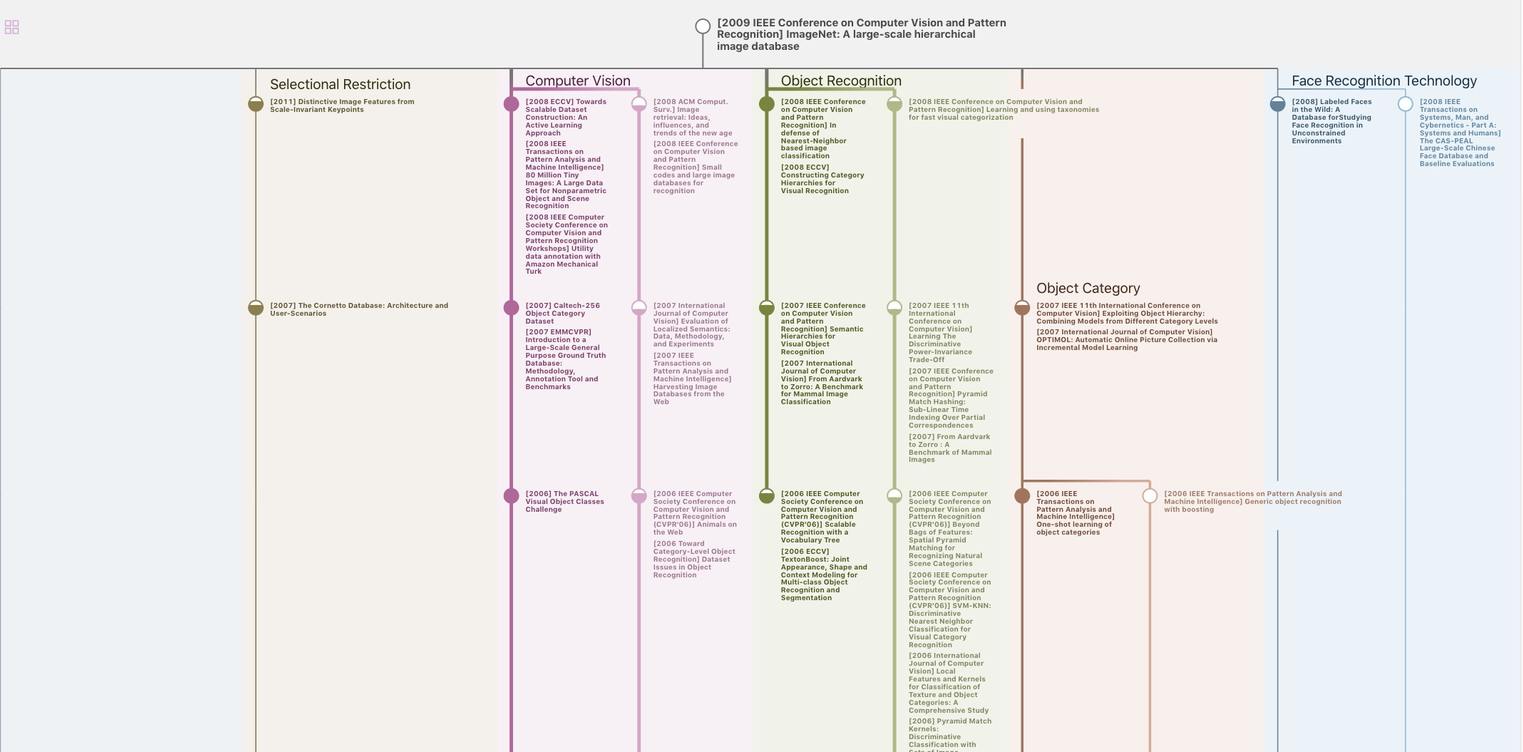
生成溯源树,研究论文发展脉络
Chat Paper
正在生成论文摘要