Variational Encoding and Decoding for Hybrid Supervision of Registration Network
MLMI@MICCAI(2021)
Abstract
Various progresses have been made in improving the accuracy of deep learning-based registration. However, there are still some limitations with current methods because of 1) difficulty of acquiring supervised data, 2) challenge of optimizing image similarity and enforcing deformation regularization, and 3) small number of training samples in such an ill-posed problem. It is believed that prior knowledge about the variability of a population could be incorporated to guide the network training to overcome these limitations. In this paper, we propose a group variational decoding-based training strategy to incorporate statistical priors of deformations for network supervision. Specifically, a variational auto-encoder is employed to learn the manifold for reconstructing deformations from a group of valid samples by projecting deformations into a low dimension latent space. Valid transformations can be simulated to serve as the ground-truth for supervised learning of registration. By working alternatively with the conventional unsupervised training, our registration network can better adapt to shape variability and yield accurate and consistent deformations. Experiments on 3D brain magnetic resonance (MR) images show that our proposed method performs better in terms of registration accuracy, consistency, and topological correctness.
MoreTranslated text
Key words
Medical image registration,Population-based regularization,Deep learning,Data modeling,Deformation consistency
AI Read Science
Must-Reading Tree
Example
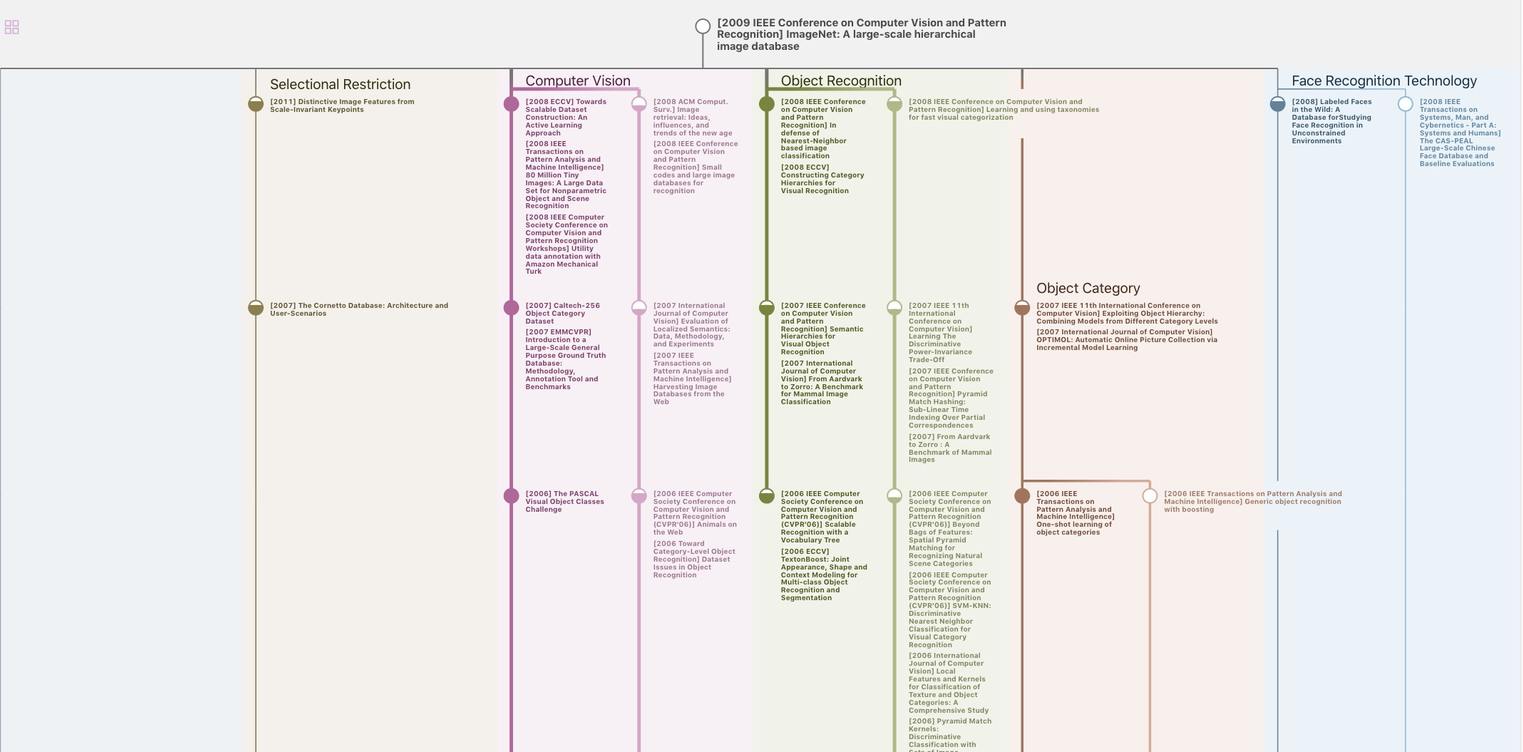
Generate MRT to find the research sequence of this paper
Chat Paper
Summary is being generated by the instructions you defined