A Unified Framework for Improving Misclassifications in Modern Deep Neural Networks for Sentiment Analysis
2021 INTERNATIONAL JOINT CONFERENCE ON NEURAL NETWORKS (IJCNN)(2021)
摘要
Deep Neural Networks (DNNs) have achieved high accuracy in multiple Natural Language Processing (NLP) applications. The great success lies in the test data is drawn from the same distribution of the training samples. However, researches have found that the current models classify out-of-distribution, adversarial, and erroneous samples incorrectly with high confidence. Researchers also find the problem comes from the softmax layer of DNN. In this paper, we address this issue and propose a method that ignores the softmax layer in the DNN architecture. Specifically, we estimate the training samples' parameters of the output of the pre-softmax layer of DNN using the Dirichlet Process Gaussian Mixture Model (DPGMM). Then, we compute the distance between a test sample and the distribution of the training samples using Mahalanobis distance to get the classification results. We evaluate our method on a classic NLP task, sentiment analysis, by conducting extensive experiments on different models across several real-world datasets. The results demonstrate that our method assigns correct labels to the samples that are misclassified by current DNNs with softmax layer. Our method can be generalized to any pre-trained DNN without the need to re-train the models and it also does not need supervision learning.
更多查看译文
关键词
Deep Neural Network,Misclassification samples,Softmax,Sentiment Analysis
AI 理解论文
溯源树
样例
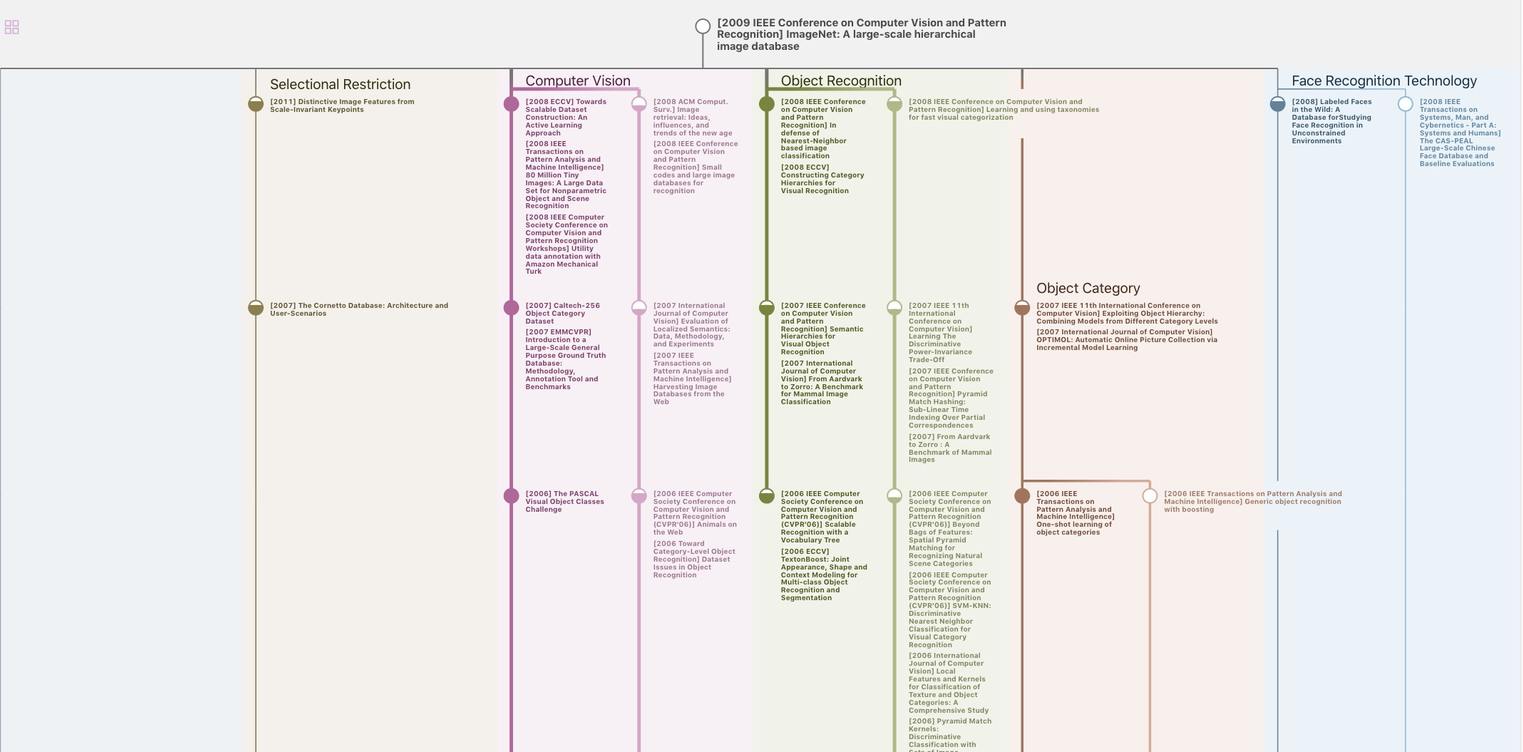
生成溯源树,研究论文发展脉络
Chat Paper
正在生成论文摘要