Modeling Edge Features with Deep Bayesian Graph Networks
2021 INTERNATIONAL JOINT CONFERENCE ON NEURAL NETWORKS (IJCNN)(2021)
Abstract
We propose an extension of the Contextual Graph Markov Model, a deep and probabilistic machine learning model for graphs, to model the distribution of edge features. Our approach is architectural, as we introduce an additional Bayesian network mapping edge features into discrete states to be used by the original model. In doing so, we are also able to build richer graph representations even in the absence of edge features, which is confirmed by the performance improvements on standard graph classification benchmarks. Moreover, we successfully test our proposal in a graph regression scenario where edge features are of fundamental importance, and we show that the learned edge representation provides substantial performance improvements against the original model on three link prediction tasks. By keeping the computational complexity linear in the number of edges, the proposed model is amenable to large-scale graph processing.
MoreTranslated text
Key words
deep Bayesian Graph networks,deep machine learning model,probabilistic machine learning model,standard graph classification benchmarks,graph regression scenario,learned edge representation,large-scale graph processing,edge feature modeling,contextual graph Markov model,Bayesian network mapping edge features,graph representations,computational complexity
AI Read Science
Must-Reading Tree
Example
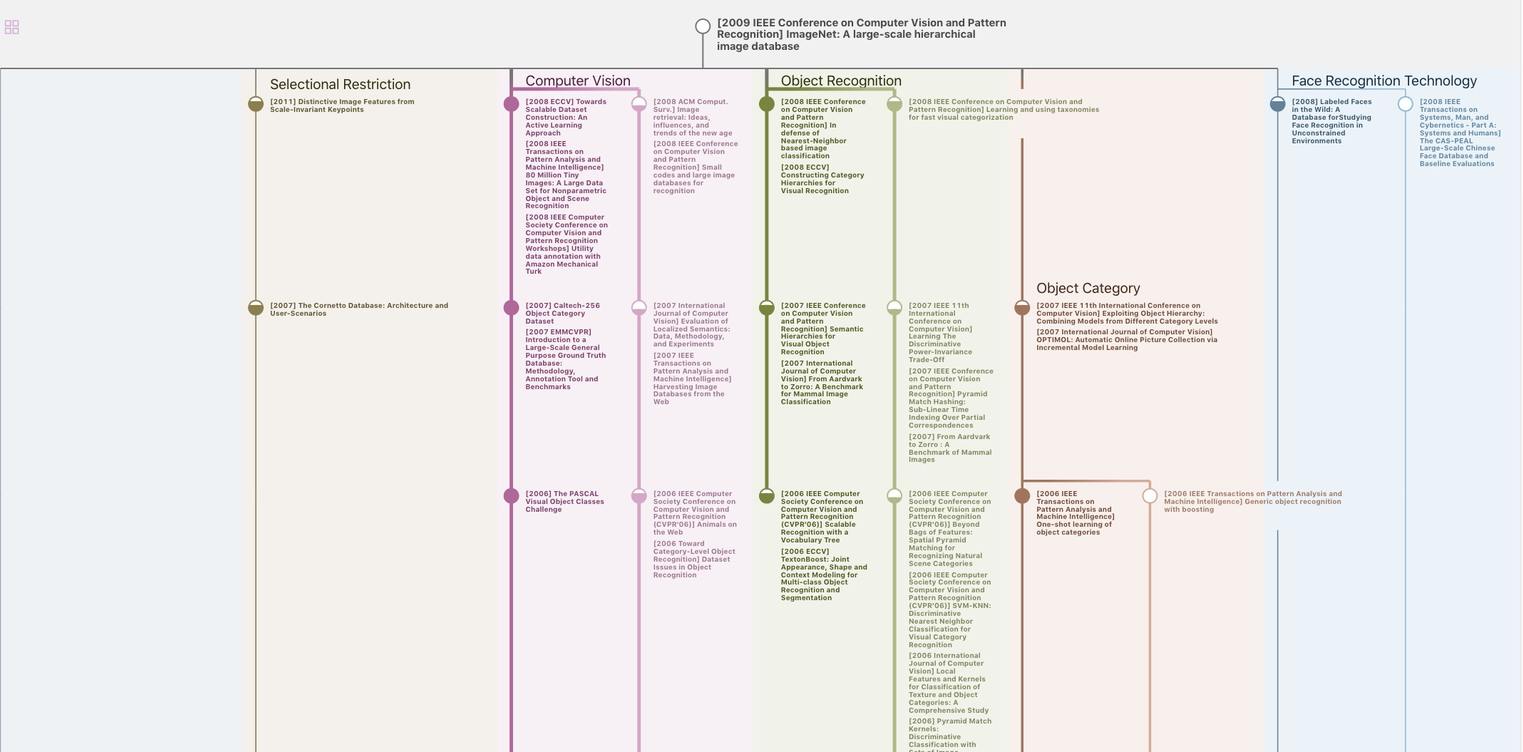
Generate MRT to find the research sequence of this paper
Chat Paper
Summary is being generated by the instructions you defined