Tensorial Time Series Prediction via Tensor Neural Ordinary Differential Equations
2021 INTERNATIONAL JOINT CONFERENCE ON NEURAL NETWORKS (IJCNN)(2021)
摘要
In high dimensional tensorial time series prediction, it is highly desired to preserve spatial structural and underlying continuous sequential information in modelling. Existing methods either destroy the spatial structure and require a large number of parameters, such as neural ordinary differential equations (neural ODEs), or cannot capture the temporal information, especially for unevenly spaced time series, such as tensorial neural network families. Hence we propose Tensor Neural Ordinary Differential Equations (TENODEs) to address these issues. The dynamics of data is modelled by a tensorial neural network (TNN) consisting of the Tucker structure. Compared with neural ODEs, it reduces the number of parameters from
$O(I^{2N})$
to
$O(NI^{2})$
. To further ease the computational cost, we also propose a TENODE with further dimensionality reduction which produces a low-dimensional representation of the aforementioned two pieces of information and is projected to the target space by a 1-layer TNN. In each layer of the RHS for TENODE, the weight matrices should maintain Lipschitzness according to the Picard's theorem. Hence TENODEs are consistent and converge. Multiplication of weight matrices together can affect the optimisation stability: when values of some weights are enlarged, values of the other weights are correspondingly decreased, with the loss unchanged. This may create infinite solution spaces. We thus constrain weights to be orthogonal and solve this scaling issue to enhance the optimisation process. In consequence, the proposed TEN-ODEs successfully preserve data spatial and latent continuous sequential information. Experimental results demonstrate the efficacy of our proposed methods over competitive baselines.
更多查看译文
关键词
neural ODE, tensorial time series
AI 理解论文
溯源树
样例
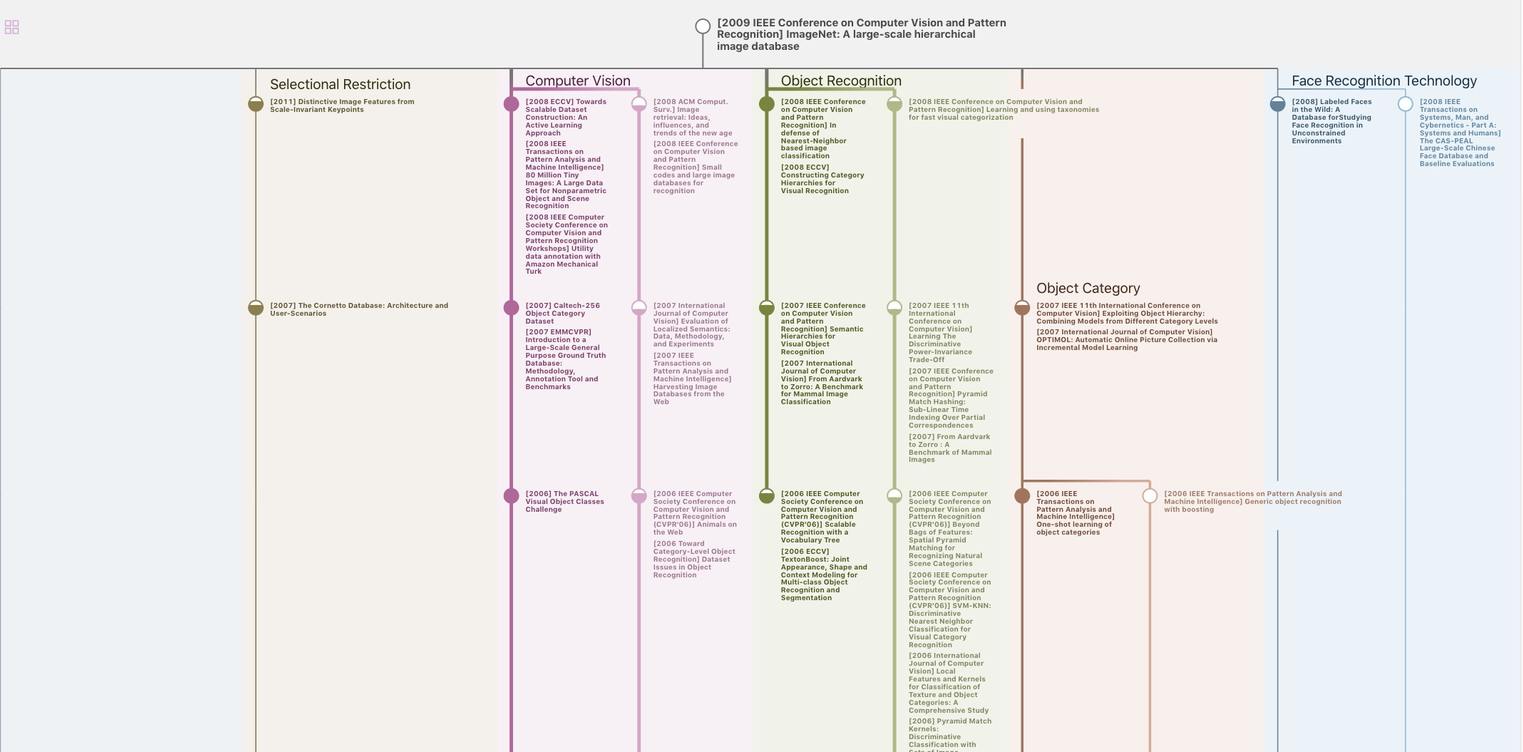
生成溯源树,研究论文发展脉络
Chat Paper
正在生成论文摘要