Soft and Hard Filter Pruning via Dimension Reduction
2021 INTERNATIONAL JOINT CONFERENCE ON NEURAL NETWORKS (IJCNN)(2021)
摘要
Filter pruning is widely used to reduce the computation of deep learning, enabling the deployment of Deep Neural Networks (DNNs) in resource-limited devices. Conventional Hard Filter Pruning (HFP) method zeroizes pruned filters and stops updating them, thus reducing the search space of the model. On the contrary, Soft Filter Pruning (SFP) simply zeroizes pruned filters, keeping updating them in the following training epochs, thus maintaining the capacity of the network. However, SFP, together with its variants, converges much slower than HFP due to its larger search space. Firstly, we generalize SFPbased methods and HFP to analyze their characteristics. Then we propose a Gradually Hard Filter Pruning (GHFP) method to smoothly switch from SFP-based methods to HFP during training and pruning, thus maintaining a large search space at first, gradually reducing the capacity of the model to ensure a moderate convergence speed. Furthermore, we view filter pruning as dimension reduction and propose a novel dimension reduction block integrated into GHFP to significantly outperform other methods by a moderate margin.
更多查看译文
关键词
Filter Pruning, Dimension Reduction, Network Compression, Neural Network, Classification
AI 理解论文
溯源树
样例
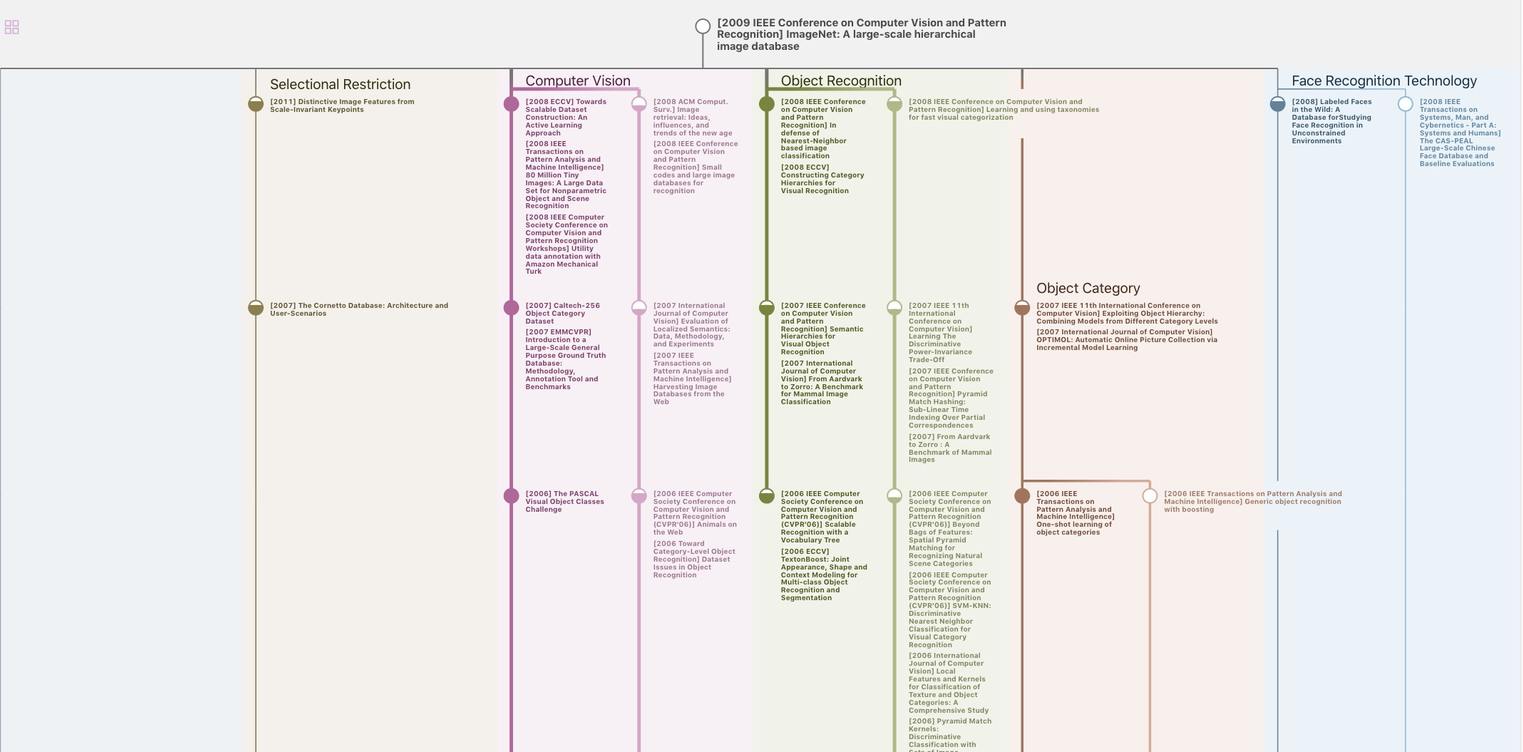
生成溯源树,研究论文发展脉络
Chat Paper
正在生成论文摘要