On Accurate Computation of Trajectory Similarity via Single Image Super-Resolution
2021 INTERNATIONAL JOINT CONFERENCE ON NEURAL NETWORKS (IJCNN)(2021)
摘要
Measuring the similarity between trajectories is fundamental to many location-aware applications. However, the trajectory data collected in the real world suffer from the low-quality problem caused by non-uniform sampling rates and noises, which significantly affects the accuracy of similarity measurement. Traditional pairwise point-matching methods are susceptible to non-uniform sampling rates inherently, since they assume the consistent sampling rate. Although the recurrent neural network (RNN) based methods have addressed this problem by complementing trajectory data, they have the drawback of predicting positions conditioned on the historical data generated by the model itself, which could lead to accumulated bias during the inference stage. In this paper, we propose a novel generative model to address the important issue of low-quality trajectory data based on single image super-resolution. The trajectory similarity is thus computed using trajectory images instead of the trajectory sequential data. By utilizing the images to represent trajectories, we effectively overcome the issues encountered by existing methods. Extensive experimental results using real-world datasets demonstrate that our method outperforms existing methods in terms of accuracy.
更多查看译文
关键词
location-aware applications,nonuniform sampling rates,similarity measurement,recurrent neural network,generative model,low-quality trajectory data,single image super-resolution,trajectory similarity,trajectory images,trajectory sequential data,pairwise point-matching methods,RNN
AI 理解论文
溯源树
样例
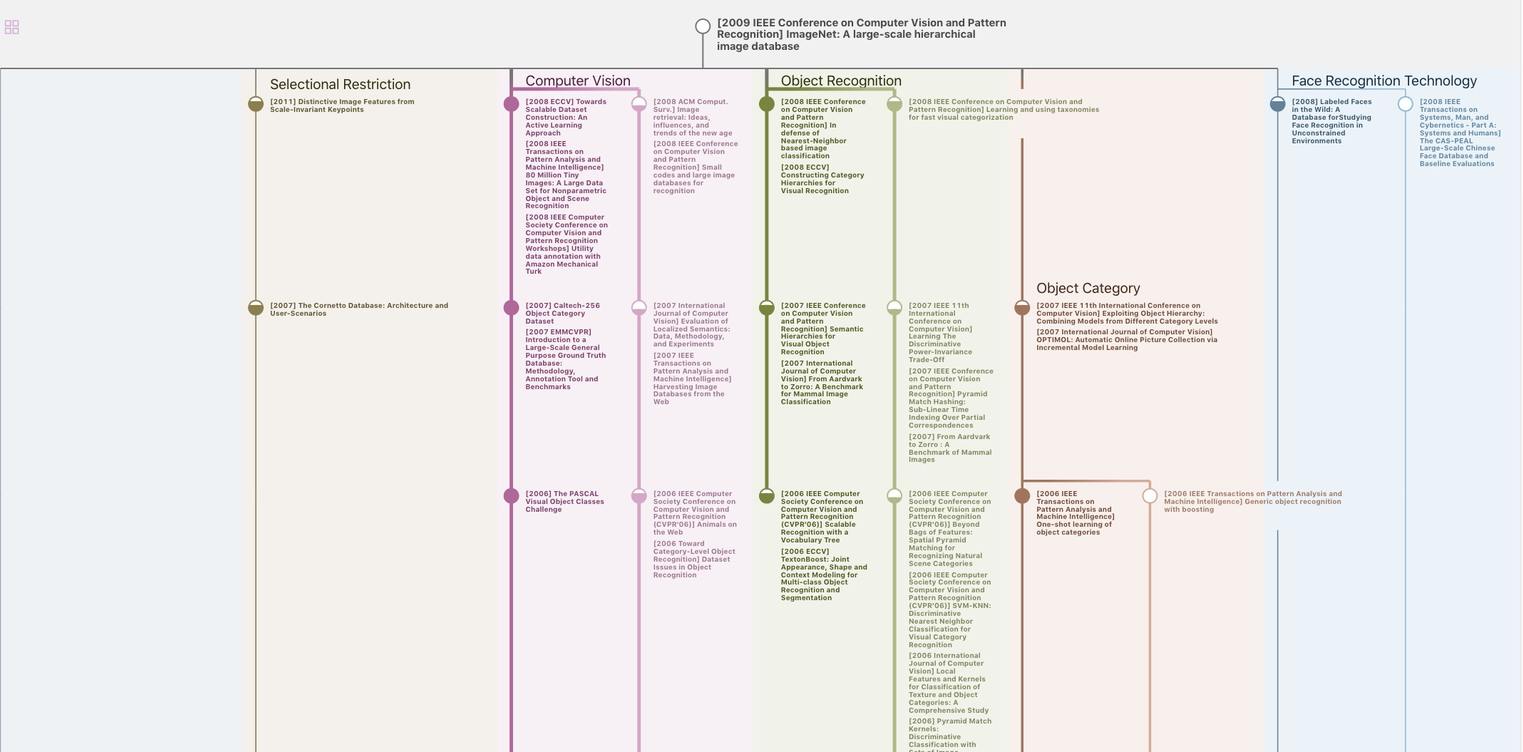
生成溯源树,研究论文发展脉络
Chat Paper
正在生成论文摘要