Task-adaptive Relation Dependent Network for Few-shot Learning
2021 INTERNATIONAL JOINT CONFERENCE ON NEURAL NETWORKS (IJCNN)(2021)
摘要
In solving learning problems with limited training data, few-shot learning is proposed to remember some common knowledge by leveraging a large number of similar few-shot tasks and learning how to adapt a base-learner to a new task for which only a few labeled samples are available. Among different few-shot learning algorithms, metric-based methods, which focus on how to acquire a robust feature embedding space or distance metric for classification, are most studied and believed to be effective for classification tasks. Despite the success of the metric-based methods, the distribution of training data and test data in the same few shot classification task is inconsistent with a distribution bias between them, which affects the quality of feature embeddings. In addition, the way of directly comparing the original features as a whole is deficient. It doesn't emphasize the features with high correlation between samples and thus can't distinguish the differences adequately. This manuscript proposes a novel metric-based few-shot algorithm called Task-adaptive Relation Dependent Network. The method reduces the distribution bias by shifting the dataset and adopting a more detailed comparison of features to capture their intrinsic correspondence, improving the measurements of the similarity between the support set and the query set samples. An experiment is conducted and the results demonstrate that the proposed method has a significant improvement over the baseline on the standard few-shot image classification benchmark datasets.
更多查看译文
关键词
training data,few-shot tasks,base-learner,few-shot learning algorithms,metric-based methods,robust feature embedding space,classification tasks,test data,shot classification task,distribution bias,standard few-shot image classification benchmark datasets,task-adaptive relation dependent network
AI 理解论文
溯源树
样例
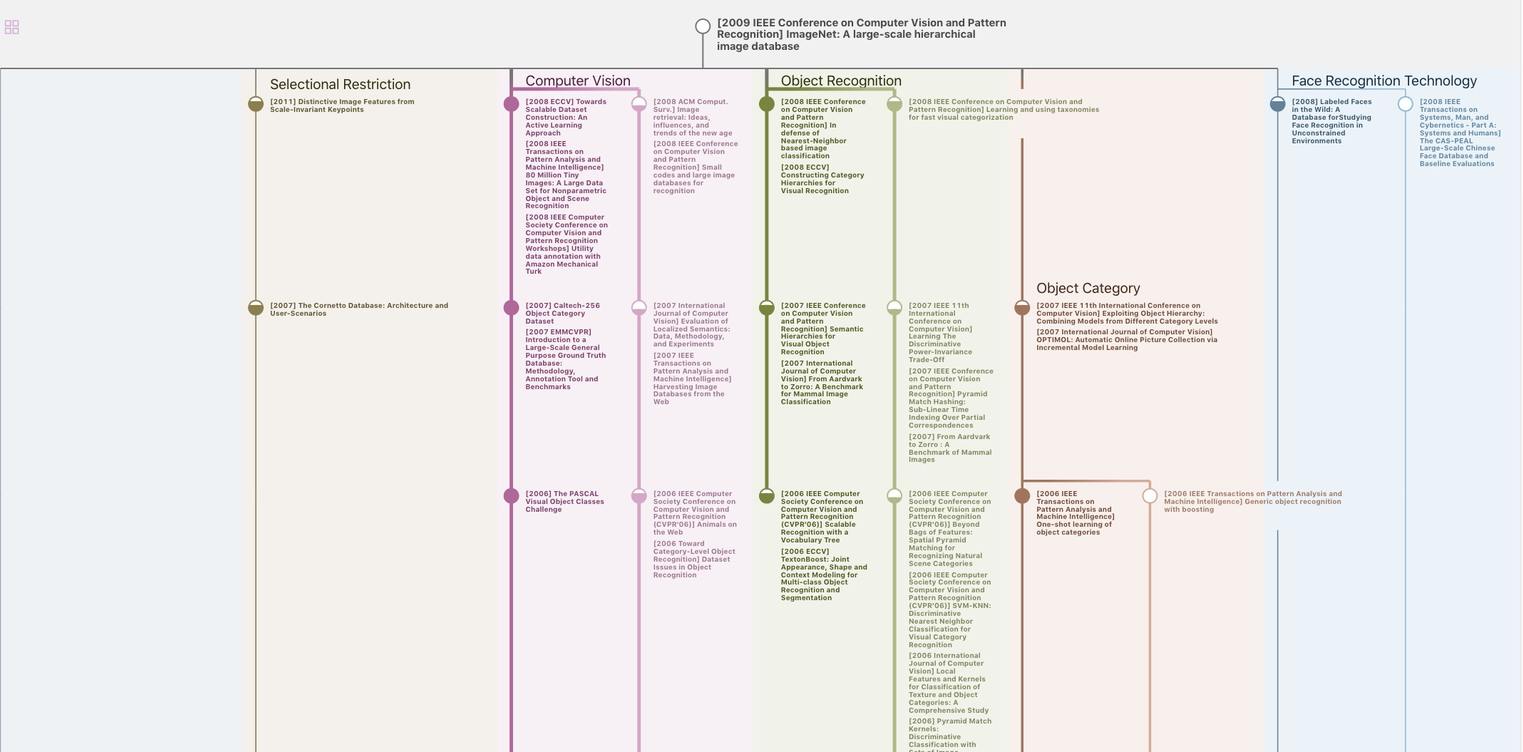
生成溯源树,研究论文发展脉络
Chat Paper
正在生成论文摘要