Semantically Constrained Document-Level Chinese-Mongolian Neural Machine Translation
2021 INTERNATIONAL JOINT CONFERENCE ON NEURAL NETWORKS (IJCNN)(2021)
摘要
By using document-level contextual information, document-level neural machine translation can achieve better results than ordinary machine translation, but traditional document-level machine translation is difficult to focus on the contextual sentence articulation relations and deep positional relations within the discourse while utilizing document-level vocabulary, and the model can concentrate only on relatively shallow inter-sentential relations or positional information. In this paper, we consider that most adjacent sentences are connected in document translation, and such links help improve the quality of translation. We propose a document translation model that focuses more on inter-sentential relations based on the previous work, and propose two methods to strengthen the model's positional information input, and combine these two methods to enhance the traditional Transformer positional information input. This paper also proposes a method for inserting paragraph information to allow inter-sentential relations to be learned by the model, and uses the improved Transformer model for Chinese-Mongolian document translation. Experiments show that in the improved Transformer system, the BLEU scores are enhanced on the Chinese-Mongolian machine translation task after fusing positional information and inter-sentential relation information, and the translation achieves better performance.
更多查看译文
关键词
Machine Translation, Document-level, Sentential Relations, Chinese-Mongolian, Transformer
AI 理解论文
溯源树
样例
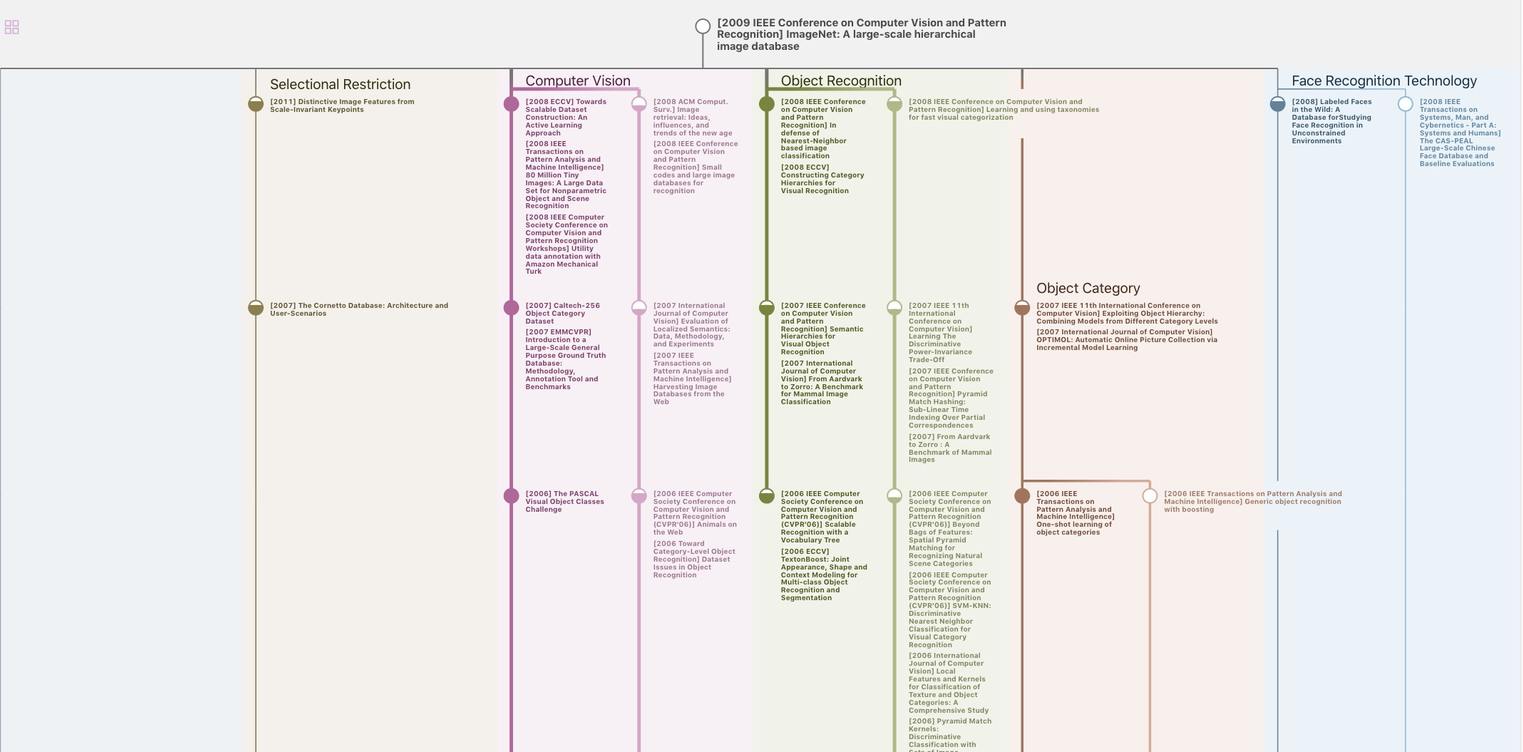
生成溯源树,研究论文发展脉络
Chat Paper
正在生成论文摘要