Selective Adversarial Adaptation Learning via Exclusive Regularization for Partial Domain Adaptation
2021 INTERNATIONAL JOINT CONFERENCE ON NEURAL NETWORKS (IJCNN)(2021)
摘要
In consideration of the suitability for the application scenario, partial domain adaptation is more significant and more valuable than traditional domain adaptation. Most existing partial domain adaptation methods adopt weighting mechanism to avoid negative migration which is caused by outlier classes samples. However, these methods give the equal consideration of each category in the source domain and determine the classes weight by classifier or discriminator, and they do not consider the possible misprediction of the similar samples from classes which are difficult to distinguish in the source domain. This situation may cause the misalignment of the outlier source classes and target classes, and the wrong alignment of the discriminators. In this work, we propose a selective adversarial adaptation learning method via exclusive regularization for partial domain adaptation (ERPDA) to solve these problems. Specifically, we utilize the exclusive regularization to extend the distance between samples of different classes in source domain to learn an inter-class separable discriminant representation to avoid negative transfer. Meanwhile, the positive transfer is performed by Joint Maximum Mean Discrepancy (JMMD) based on selective adaptation adversarial learning via multi-discriminator. Extensive experiments show that ERPDA achieves state-of-the-art results on several partial domain adaptation benchmark datasets.
更多查看译文
关键词
selective adaptation adversarial,partial domain adaptation benchmark datasets,exclusive regularization,traditional domain adaptation,existing partial domain adaptation methods,outlier classes samples,source domain,classes weight,outlier source classes,selective adversarial adaptation learning method,inter-class separable discriminant representation
AI 理解论文
溯源树
样例
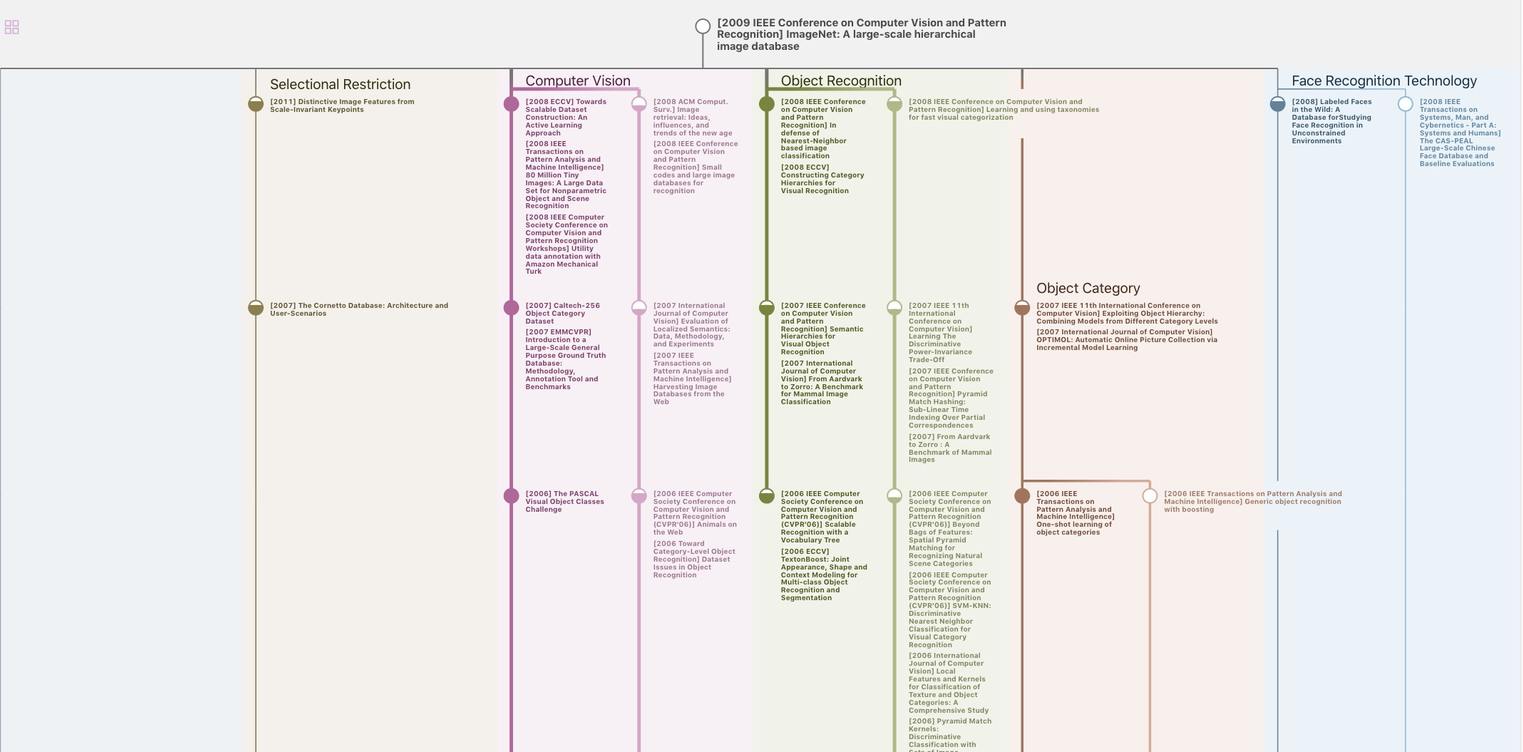
生成溯源树,研究论文发展脉络
Chat Paper
正在生成论文摘要