Attention and Adaptive Bilinear Matching Network for Cross-Domain Few-Shot Defect Classification of Industrial Parts
2021 INTERNATIONAL JOINT CONFERENCE ON NEURAL NETWORKS (IJCNN)(2021)
摘要
In the task of cross-domain few-shot defect classification on industrial part images, it's a challenging research problem that how to effectively reduce the feature distribution between different parts and increase the feature information distance of different defects of the same part. We propose a simple yet effective approach named attention and adaptive bilinear matching network (AABM). Equipped with Residual Attention Module (RAM) to enrich feature information from different semantic layer, the feature extractor could improve the poor performance caused by the issue that dataset covers too many categories. In addition, the adaptive bilinear matching network (ABM) is utilized to learn the feature information and make forecast for solving the problem of fine-grained image analysis in cross-domain few-shot classification. Specifically, we have inserted RAM behind each residual block in a stacked manner based on RESNET-10 as the backbone of feature encoder, which enables linear enhancement of the feature extractor. After the procedure of passing the feature information through an adaptive bilinear matching network whose weights not share between the sub-network, two different posterior probabilities are calculated and the final prediction result is obtained by merging them with the adaptive parameters from the last feature layer through full connection. The results on benchmark and part image datasets demonstrates that our model achieve better performances on cross-domain few-shot classification and our AABM achieves is competitive compared to the state-of-art approaches.
更多查看译文
关键词
Few-shot classification, cross-domain, matching network, defect classification for part image
AI 理解论文
溯源树
样例
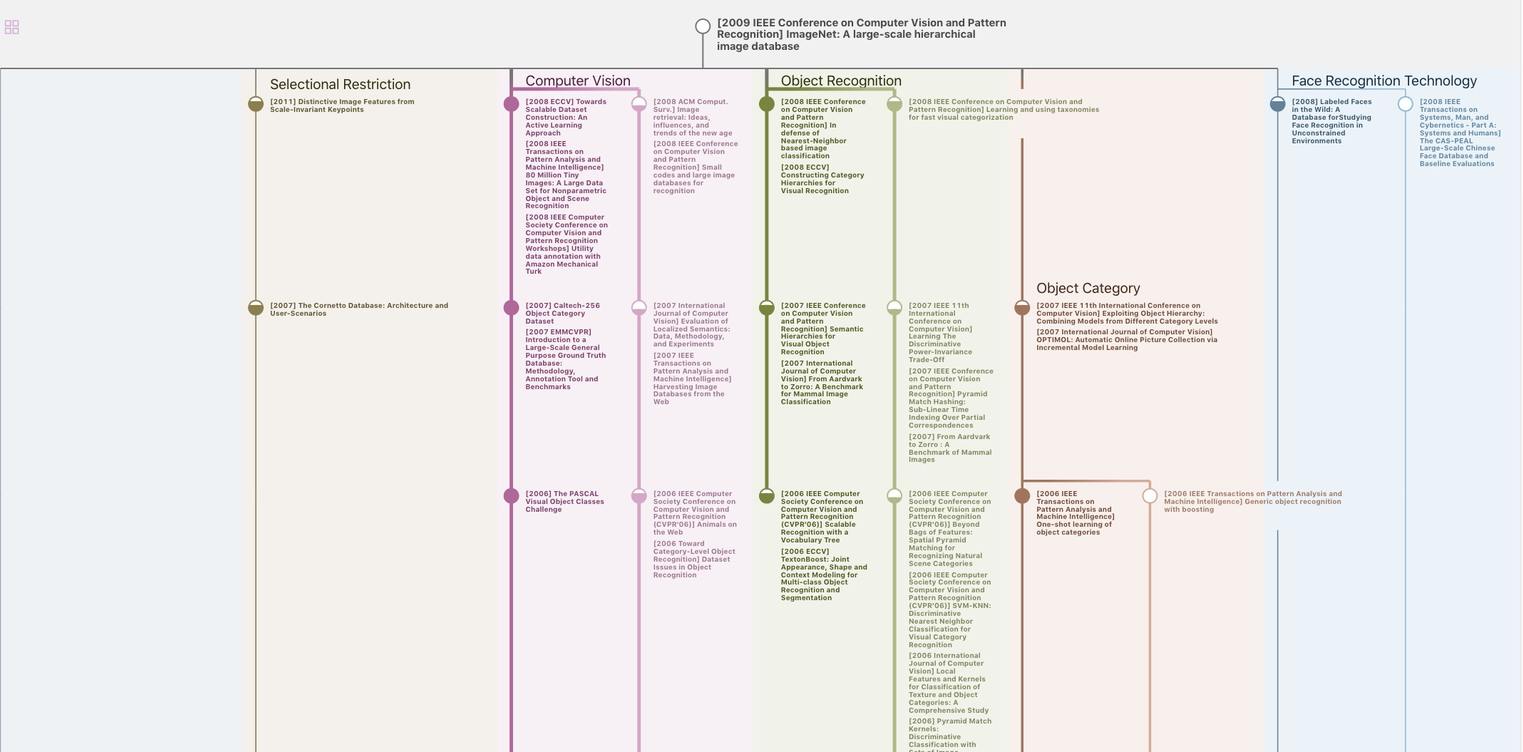
生成溯源树,研究论文发展脉络
Chat Paper
正在生成论文摘要