Group-Based Deep Transfer Learning with Mixed Gate Control for Cross-Domain Recommendation
2021 INTERNATIONAL JOINT CONFERENCE ON NEURAL NETWORKS (IJCNN)(2021)
摘要
For modern recommender systems, the issue of data sparsity still often arises in many cases. To address this challenge, Cross-Domain Recommendation (CDR) has been explored by transferring knowledge learned from the source domain to alleviate the data sparsity in the target domain, and existing methods typically assume intra-domain samples share more commonalities than variabilities. However, it can also cause negative effects when there exists a high diversity among intra-domain samples. In this paper, we propose a novel Mixed Gate Control (MGC) model to fulfill 1) the division of samples into groups according to their commonalities and 2) the transfer of knowledge based on groups. We stack up multiple MGC layers into Multiple Layers MGC (ML-MGC) and apply it to a large-scale bundle retrieval system in a listed internet company. We conduct experiments on its own commercial dataset and two public real-world datasets. Experimental results show the superiority of our model against state-of-the-art methods for the CDR task. Finally, we present a case study to illustrate the groups identified by our model.
更多查看译文
关键词
Recommender Systems, Cross-domain Recommendation, Transfer Learning, Deep Learning
AI 理解论文
溯源树
样例
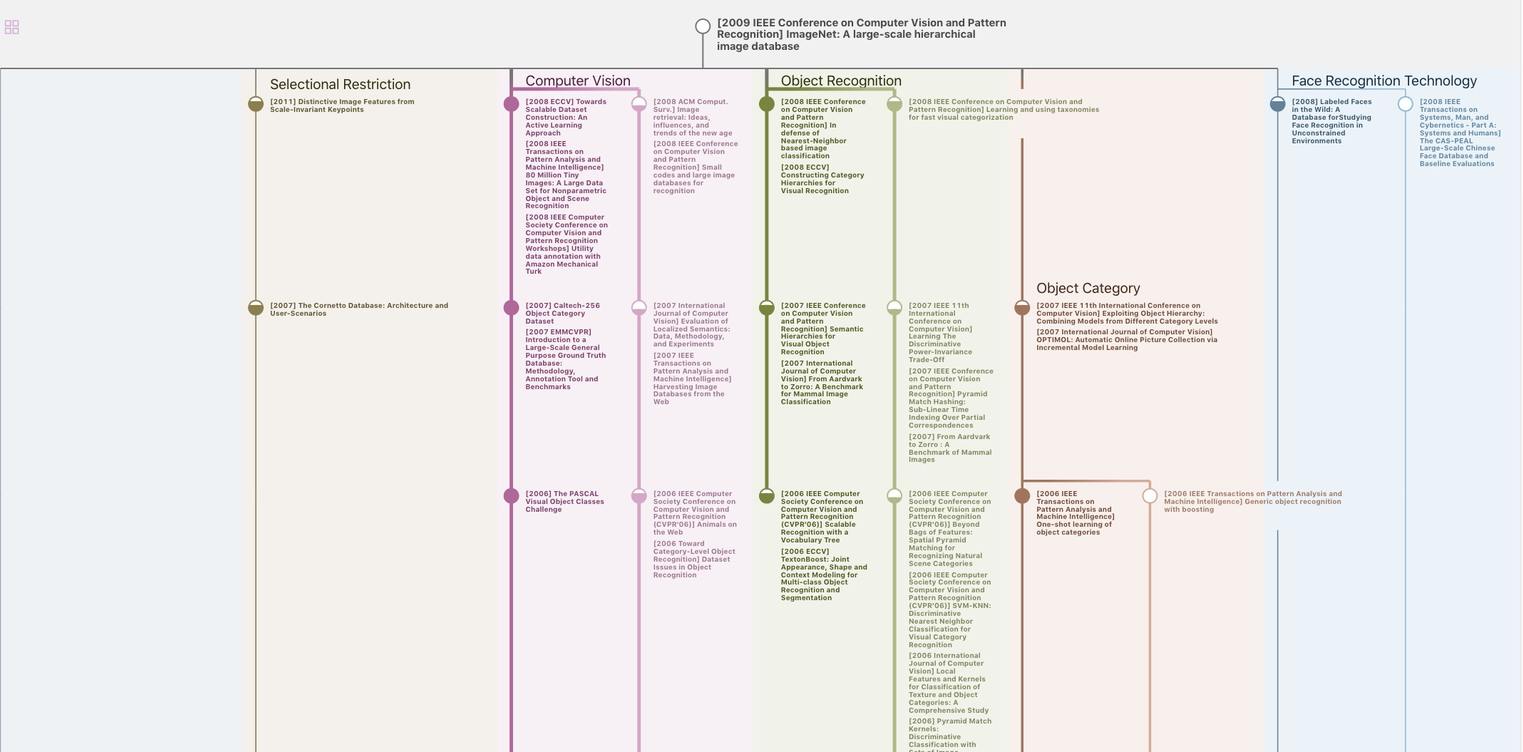
生成溯源树,研究论文发展脉络
Chat Paper
正在生成论文摘要