Weakly Labeled Semi-Supervised Sound Event Detection with Multi-Scale Residual Attention.
IEEE International Joint Conference on Neural Network(2021)
关键词
weakly labeled semisupervised sound event detection,multiscale residual attention,time-frequency scale characteristics,multiscale feature information,multiscale residual convolutional block,selective multiscale attention block,event classification
AI 理解论文
溯源树
样例
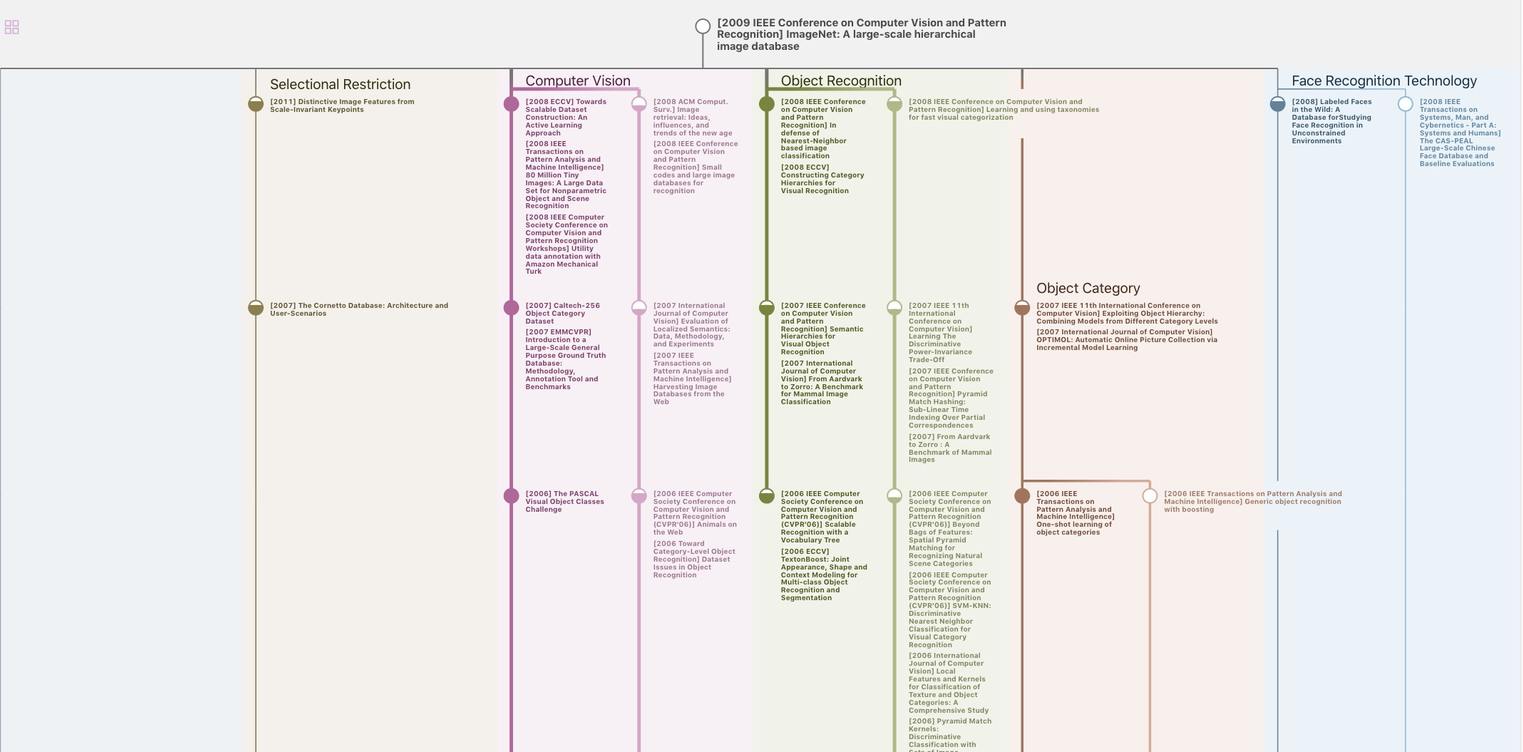
生成溯源树,研究论文发展脉络
Chat Paper
正在生成论文摘要