Spectral Clustering Joint Deep Embedding Learning by Autoencoder
2021 INTERNATIONAL JOINT CONFERENCE ON NEURAL NETWORKS (IJCNN)(2021)
摘要
Spectral clustering has become one of the most popular clustering methods due to its superior performance compared to the traditional clustering methods. However, the performance of spectral clustering would be limited by complex data, such as a huge number of samples and high dimensionality. To address this problem, some existing methods apply deep learning to learn the lower-dimensional representations, spectral clustering is then applied to the representations. Different from the existing methods that separate the two stages of feature representation learning and spectral clustering, in this paper, we propose Spectral Clustering Joint Deep Embedding (SCJDE), a method that simultaneously learns the feature representations and the spectral embedding of spectral clustering via a deep autoencoder. Moreover, a sparsity constraint is imposed to generate better spectral embedding for spectral clustering. Finally, k-means is performed on the spectral embedding to obtain clustering result. The proposed method can learn a good spectral embedding for spectral clustering by deep learning to obtain better clustering results. The experimental results on both synthetic and real-world datasets demonstrate the effectiveness of the proposed method.
更多查看译文
关键词
Spectral Clustering Joint Deep Embedding learning,popular clustering methods,deep learning,spectral embedding,feature representations,deep autoencoder
AI 理解论文
溯源树
样例
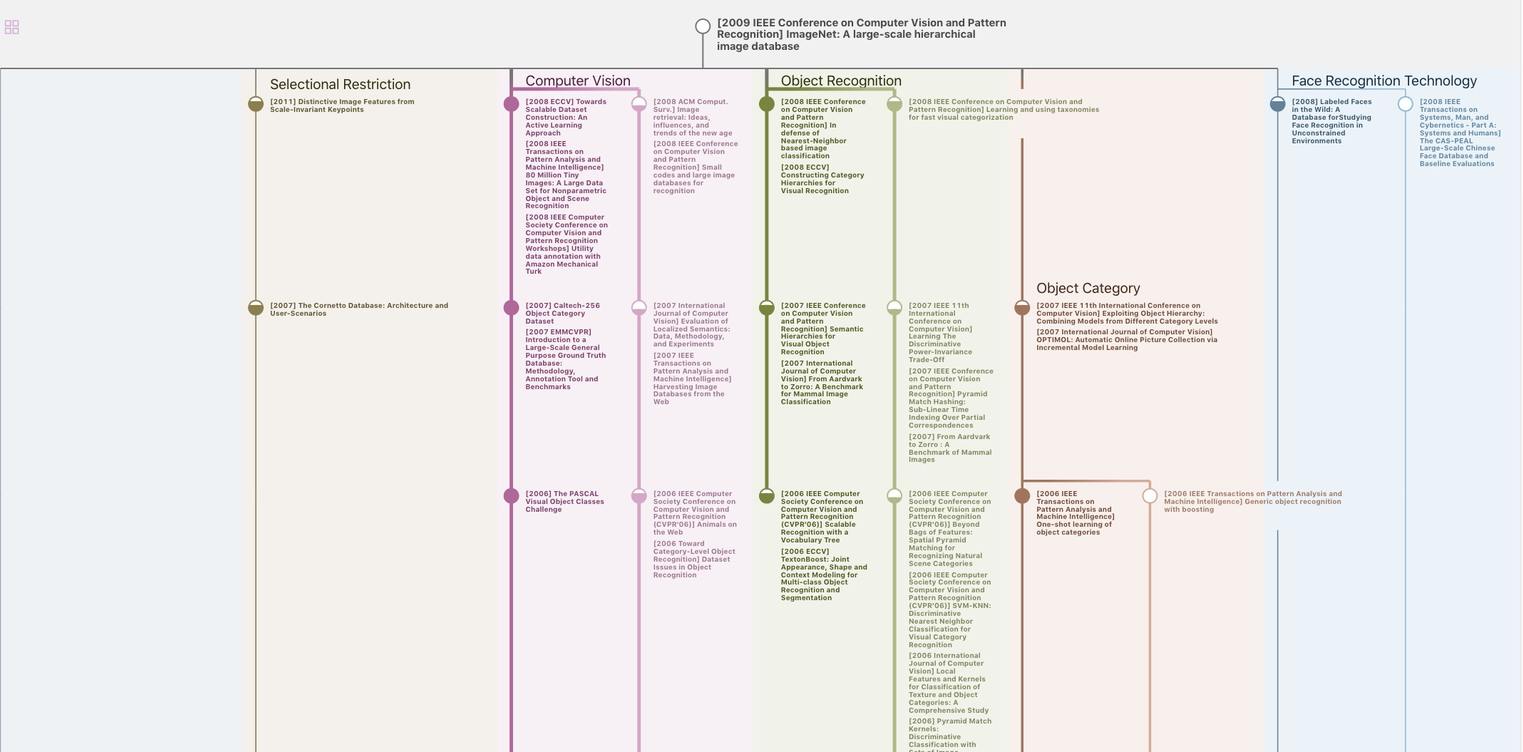
生成溯源树,研究论文发展脉络
Chat Paper
正在生成论文摘要