Robust Deep Multi-task Learning Framework for Cancer Survival Analysis
2021 INTERNATIONAL JOINT CONFERENCE ON NEURAL NETWORKS (IJCNN)(2021)
摘要
Utilizing genomic characterizations and clinical information of patients across different cancer types, Multi-task Learning (MTL) based cancer survival analysis methods achieve good generalization performance and become prevalent. However, due to the high dimensionality and scarcity of the genomic sample set, as well as the inaccuracy of clinical data, the existing MTL-based survival analysis methods are limited to adopt the traditional statistical learning methods. The deep Multi-task Learning schema for cancer survival analysis has been less explored. To fill this gap, this paper proposes a novel robust deep MTL framework for cancer survival analysis. The proposed deep MTL framework is capable of handling low sample sized data with high dimension. Besides, the framework is robust to class-imbalance and task-imbalance of the cancer survival data. Experiments conducted on The Cancer Genome Atlas data sets validate the superiority of our deep MTL framework.
更多查看译文
关键词
cancer survival analysis, multi-task learning, gene pathways
AI 理解论文
溯源树
样例
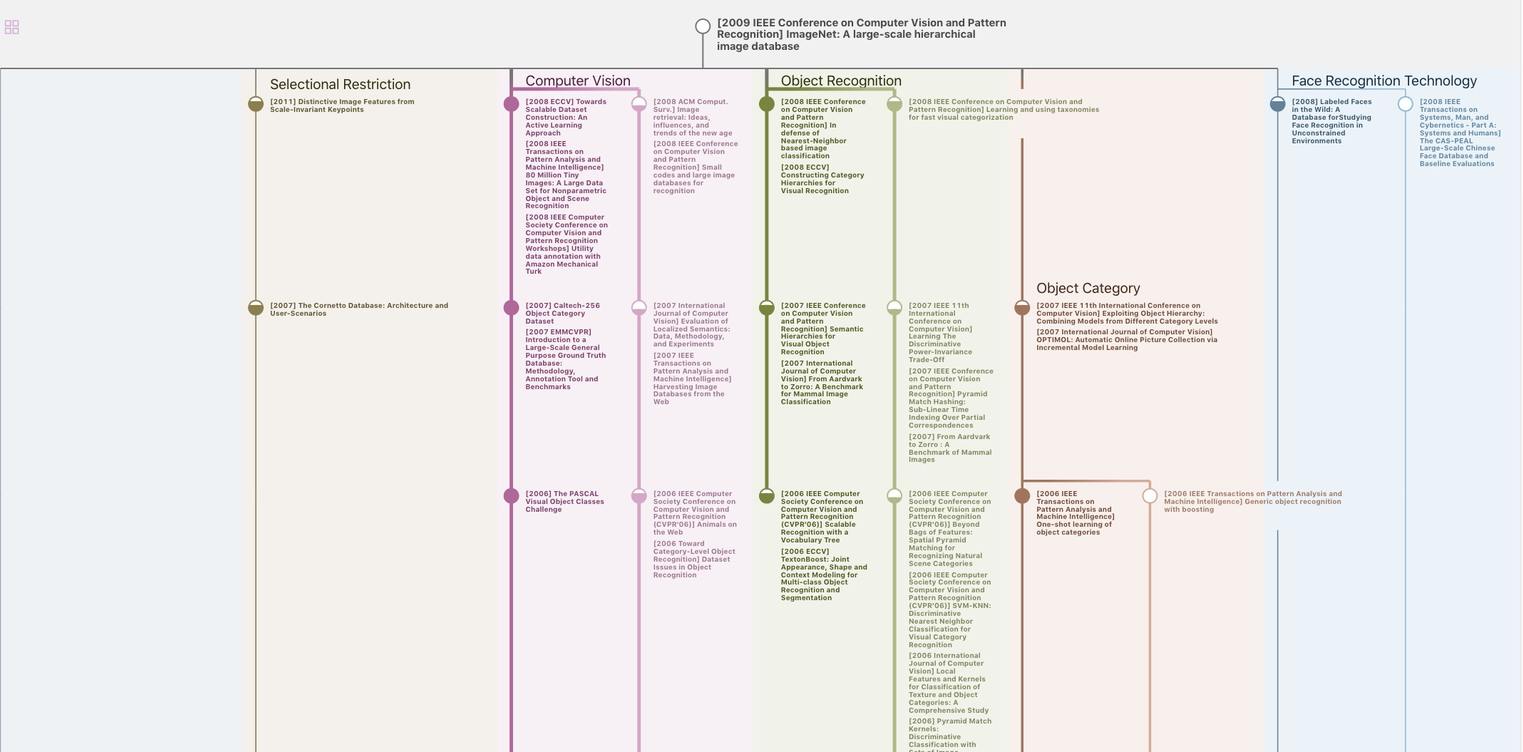
生成溯源树,研究论文发展脉络
Chat Paper
正在生成论文摘要