Multi-label Local-to-Global Feature Selection
2021 INTERNATIONAL JOINT CONFERENCE ON NEURAL NETWORKS (IJCNN)(2021)
摘要
Recent years have witnessed the proliferation of multi-label feature selection, which is an effective data preprocessing step for multi-label learning. And label correlations provide critical information for multi-label feature selection. Existing methods consider global label correlations roughly by assuming that the same label correlations are shared by all samples. Nevertheless, there may exist different local inner label correlations in different local sample sets. Although some methods try to explore them respectively in these local sample sets separated by clustering, they ignore the exterior correlation structure of different local inner correlations, leading to performance degradation. To address this problem, we novelly extract local sample sets for each label through mining Markov blankets of these labels, based on which the proposed multi-label local-to-global feature selection algorithm (ML2G) is employed to select predictive features on these sample subsets. ML2G simultaneously learns different local inner label correlations in each local sample set and the exterior structure of these local inner correlations. Moreover, different from existing methods, ML2G extra considers the asymmetric label correlations, which could describe label correlations more accurately and thus improve the performance of ML2G. Empirical studies validate the superiority of ML2G against state-of-the-art methods on real-world datasets.
更多查看译文
关键词
multi-label learning, feature selection, label correlation
AI 理解论文
溯源树
样例
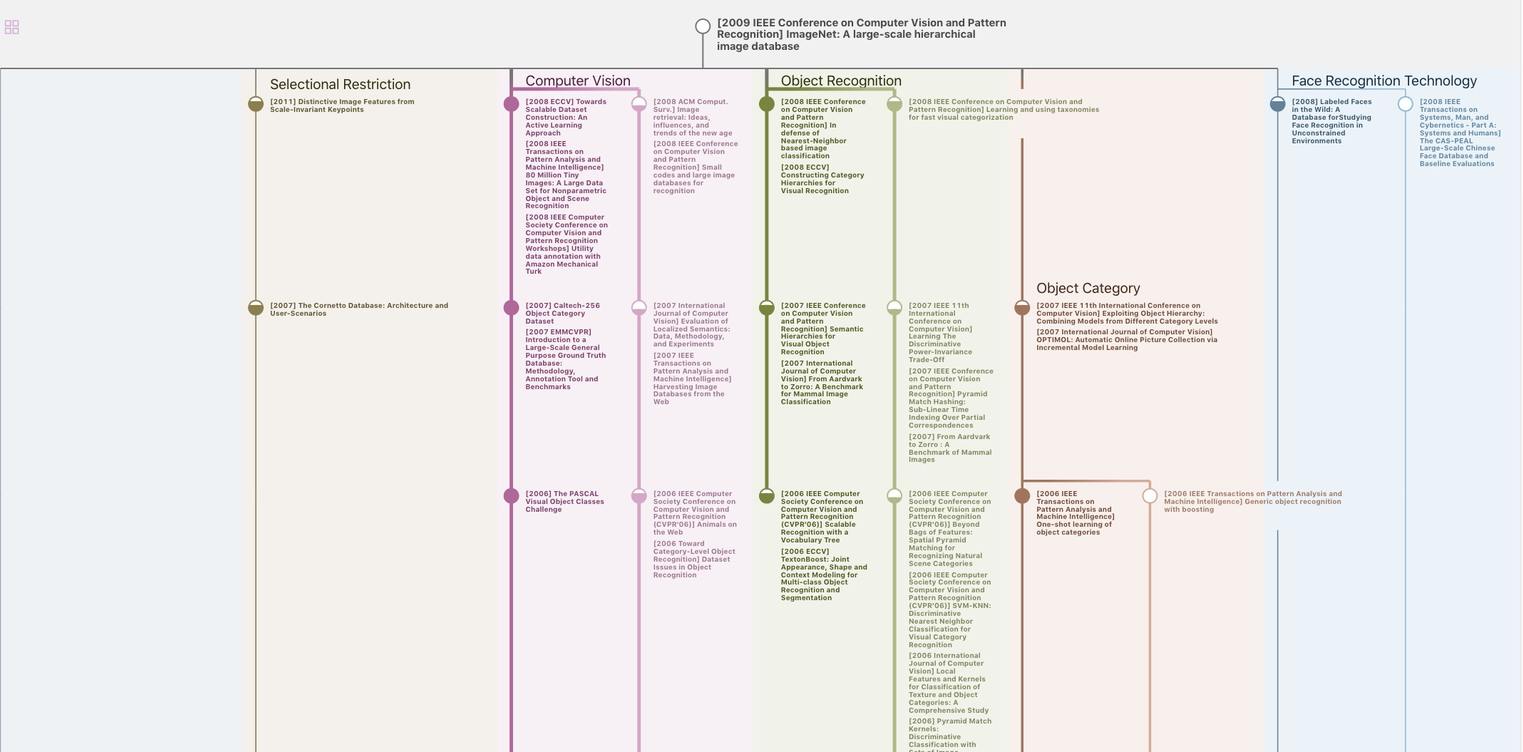
生成溯源树,研究论文发展脉络
Chat Paper
正在生成论文摘要