Product Recommendation Algorithm for Score Prediction Based on Joint Feature Vector Extraction.
DSIT(2021)
摘要
In order to make full use of multiple types of data and improve the accuracy of the recommendation algorithm, a scoring prediction model combining multi-source heterogeneous data such as product attributes, user reviews and friend relationships is proposed for product recommendation. In this algorithm, the product attributes and user comment information are combined, the combination method of LDA and Word2vec is adopted to extract user-commodity joint features, a community division algorithm is used to divide users into communities, features and product ratings are integrated to establish a rating prediction model and the recommended results are obtained by grading forecasting and ranking. The experiment results show that, compared with collaborative filtering and existing recommendation algorithms, the proposed model combining multi-source heterogeneous data can reduce the root mean square error of prediction scores, improve the accuracy of prediction, and increase the accuracy of product recommendation.
更多查看译文
AI 理解论文
溯源树
样例
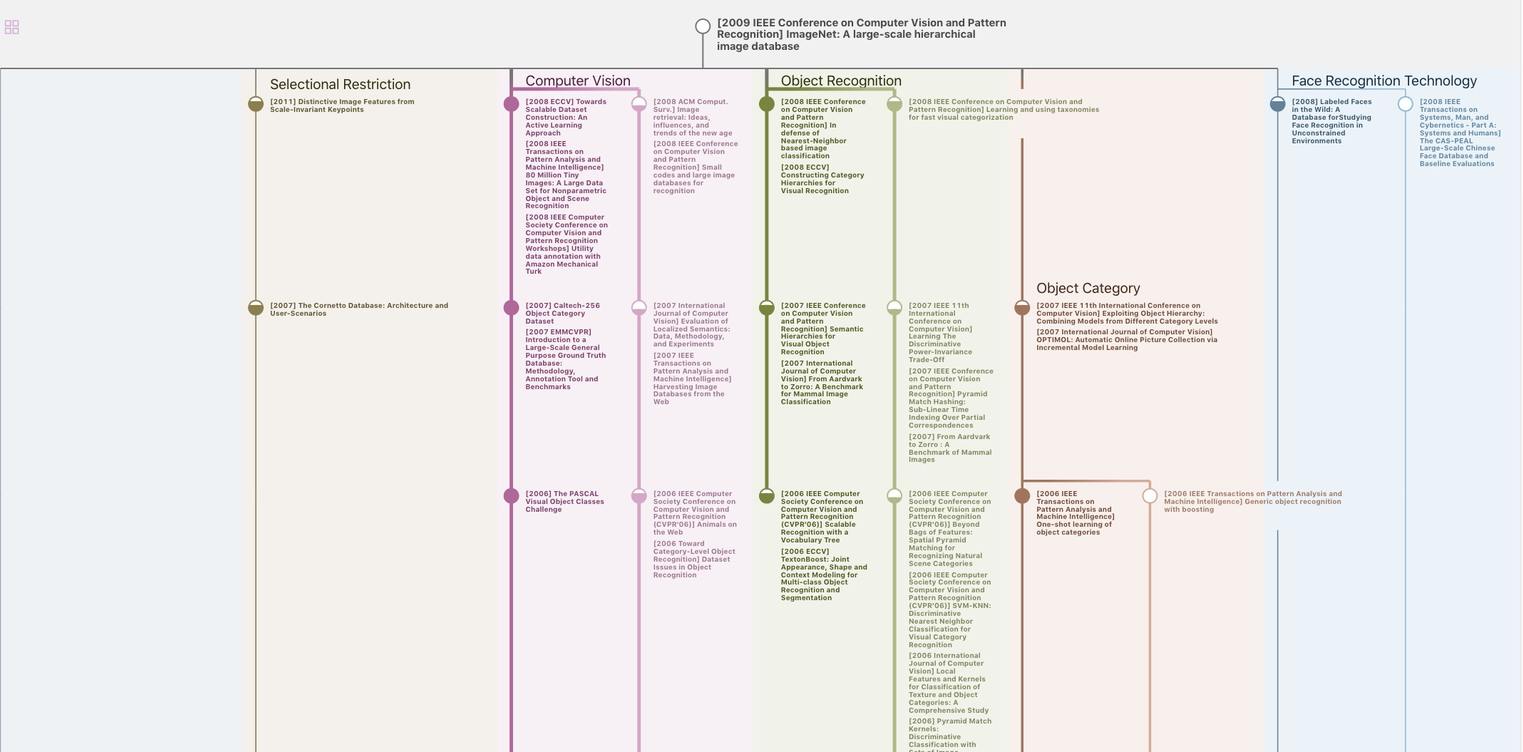
生成溯源树,研究论文发展脉络
Chat Paper
正在生成论文摘要