A Cramer Distance perspective on Quantile Regression based Distributional Reinforcement Learning
arxiv(2022)
摘要
Distributional reinforcement learning (DRL) extends the value-based approach by approximating the full distribution over future returns instead of the mean only, providing a richer signal that leads to improved performances. Quantile Regression (QR) based methods like QR-DQN project arbitrary distributions into a parametric subset of staircase distributions by minimizing the 1-Wasserstein distance. However, due to biases in the gradients, the quantile regression loss is used instead for training, guaranteeing the same minimizer and enjoying unbiased gradients. Non-crossing constraints on the quantiles have been shown to improve the performance of QR-DQN for uncertainty-based exploration strategies. The contribution of this work is in the setting of fixed quantile levels and is twofold. First, we prove that the Cramer distance yields a projection that coincides with the 1-Wasserstein one and that, under noncrossing constraints, the squared Cramer and the quantile regression losses yield collinear gradients, shedding light on the connection between these important elements of DRL. Second, we propose a low complexity algorithm to compute the Cramer distance.
更多查看译文
关键词
quantile regression,cramér distance perspective,learning
AI 理解论文
溯源树
样例
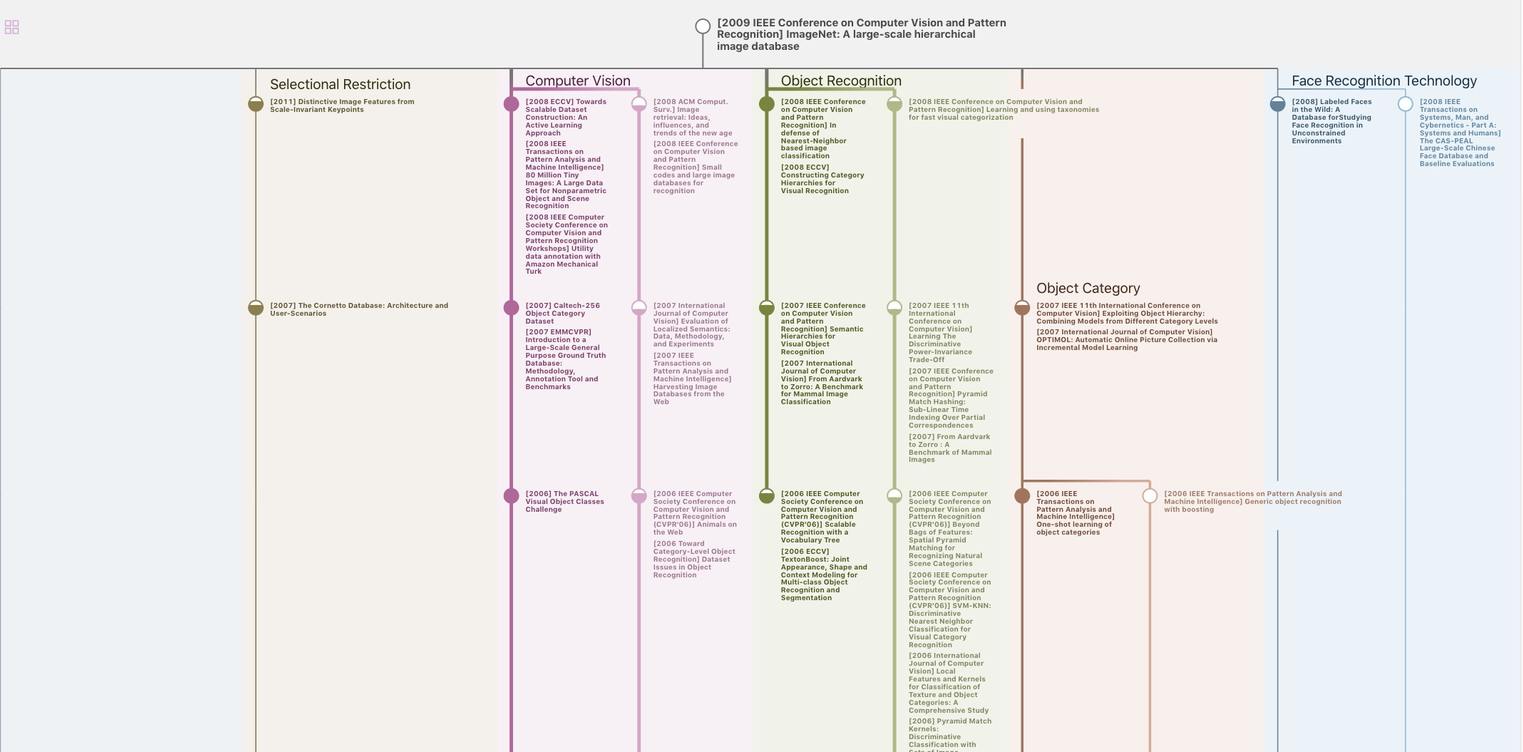
生成溯源树,研究论文发展脉络
Chat Paper
正在生成论文摘要