Volatility prediction comparison via robust volatility proxies: An empirical deviation perspective
JOURNAL OF ECONOMETRICS(2024)
摘要
Volatility forecasting is crucial to risk management and portfolio construction. One particular challenge of assessing volatility forecasts is how to construct a robust proxy for the unknown true volatility. In this work, we show that the empirical loss comparison between two volatility predictors hinges on the deviation of the volatility proxy from the true volatility. We then establish non -asymptotic deviation bounds for three robust volatility proxies, two of which are based on clipped data, and the third of which is based on exponentially weighted Huber loss minimization. In particular, in order for the Huber approach to adapt to non -stationary financial returns, we propose to solve a tuning -free weighted Huber loss minimization problem to jointly estimate the volatility and the optimal robustification parameter at each time point. We then inflate this robustification parameter and use it to update the volatility proxy to achieve optimal balance between the bias and variance of the global empirical loss. We also extend this Huber method to construct volatility predictors. Finally, we exploit the proposed robust volatility proxy to compare different volatility predictors on the Bitcoin market data and calibrated synthetic data. It turns out that when the sample size is limited, applying the robust volatility proxy gives more consistent and stable evaluation of volatility forecasts.
更多查看译文
关键词
Volatility forecasting,Robust loss function,Huber minimization,Risk management,Crypto market
AI 理解论文
溯源树
样例
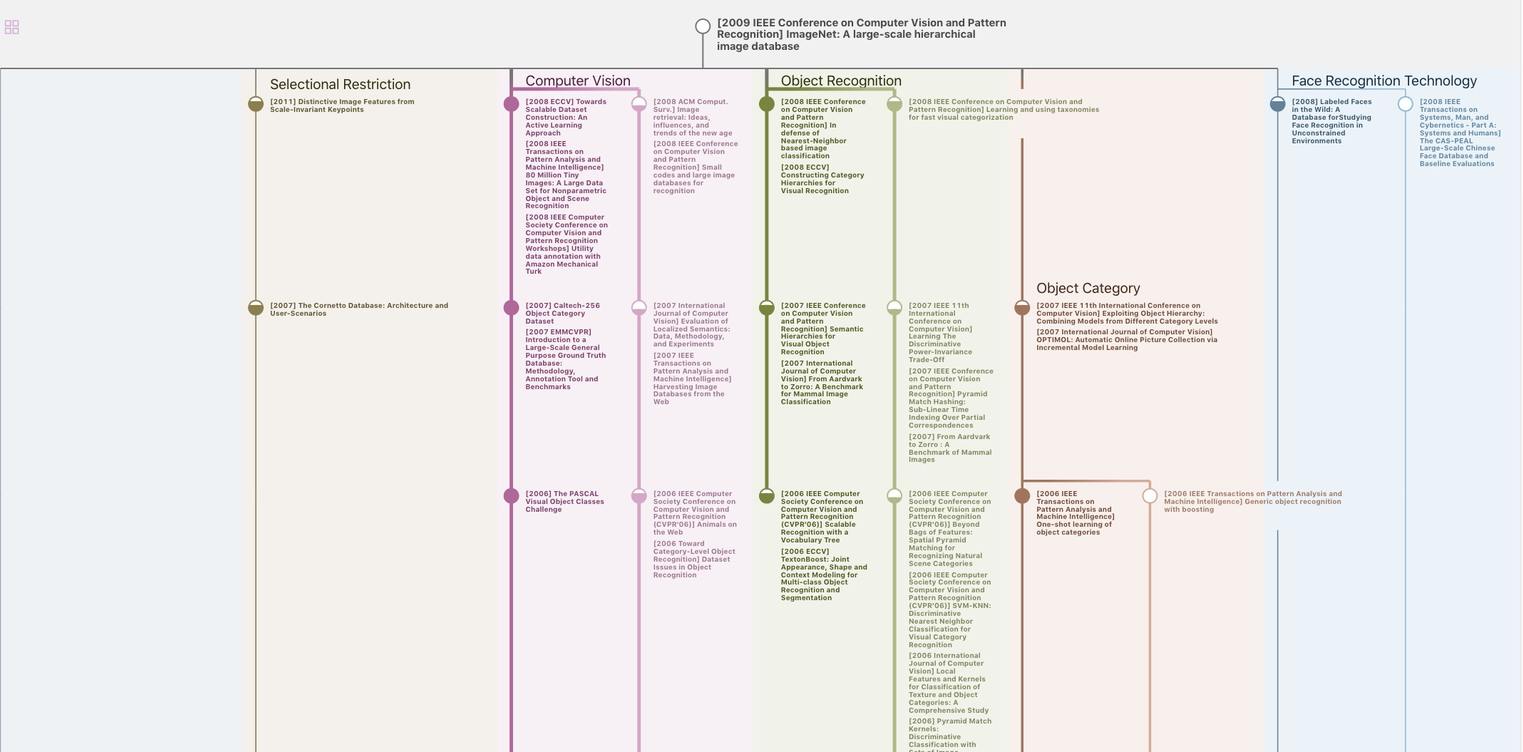
生成溯源树,研究论文发展脉络
Chat Paper
正在生成论文摘要