DRP-VEM: Drug repositioning prediction using voting ensemble
arxiv(2021)
摘要
Traditional drug discovery methods are costly and time-consuming. Drug repositioning (DR) is a common strategy to overcome these issues. Recently, machine learning methods have been used extensively in DR problem. The performance of these methods depends on the features, representations and training dataset. In this problem, feature sets include many redundant features, which have a negative effect on the performance of methods. Moreover, selecting an appropriate training set is influential in the rise of machine learning method accuracy. However, in this problem, we face two obstacles to find the proper training set. First, most methods employ known and unknown drug-disease pairs as positive and negative sets, respectively. While the number of known pairs is much less than unknowns, it leads to machine learning performance error because of biasing to the majority group. Second, the absence of a drug-disease association means this association has not been approved experimentally and may be changed. In this paper, DRP-VEM framework is proposed to overcome the challenges. We assess DRP-VEM based on different parameters: disease and drug feature representations, classification methods, and voting ensemble training approaches. DRP-VEM is evaluated using heterogenous evaluation criteria. Moreover, we compare DRP-VEM using the best combination of parameters with DisDrugPred.
更多查看译文
关键词
prediction,drug,drp-vem
AI 理解论文
溯源树
样例
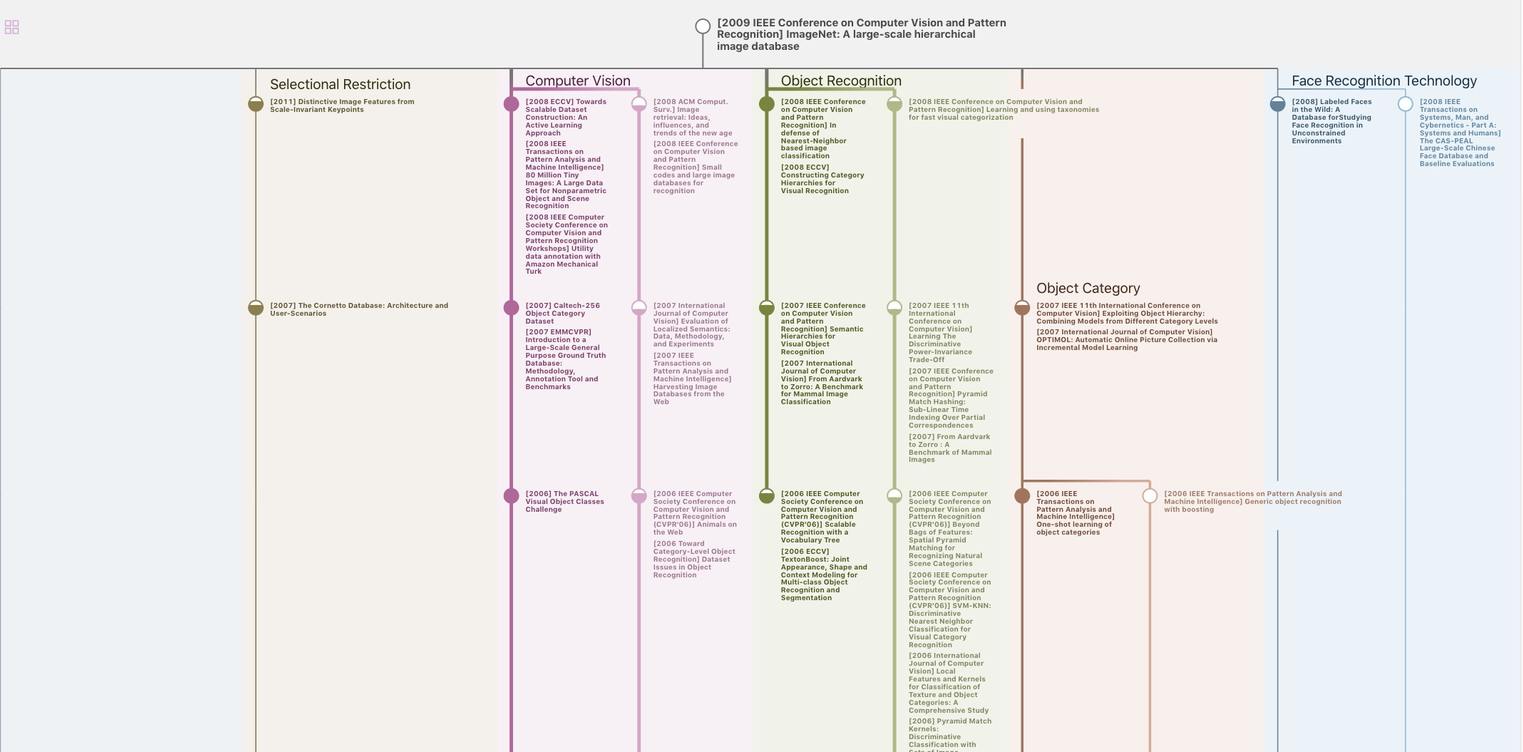
生成溯源树,研究论文发展脉络
Chat Paper
正在生成论文摘要