Multi-Object Tracking with Deep Learning Ensemble for Unmanned Aerial System Applications
ARTIFICIAL INTELLIGENCE AND MACHINE LEARNING IN DEFENSE APPLICATIONS III(2021)
摘要
Multi-object tracking (MOT) is a crucial component of situational awareness in military defense applications. With the growing use of unmanned aerial systems (UASs), MOT methods for aerial surveillance is in high demand. Application of MOT in UAS presents specific challenges such as moving sensor, changing zoom levels, dynamic background, illumination changes, obscurations and small objects. In this work, we present a robust object tracking architecture aimed to accommodate for the noise in real-time situations. Our work is based on the tracking-by-detection paradigm where an independent object detector is first applied to isolate all potential detections and an object tracking model is applied afterwards to link unique objects between frames. Object trajectories are constructed using multiple hypothesis tracking (MHT) framework that produces the best hypothesis based on the kinematic and visual scorings. We propose a kinematic prediction model, called Deep Extended Kalman Filter (DeepEKF), in which a sequence-to-sequence architecture is used to predict entity trajectories in latent space. DeepEKF utilizes a learned image embedding along with an attention mechanism trained to weight the importance of areas in an image to predict future states. For the visual scoring, we experiment with different similarity measures to calculate distance based on entity appearances, including a convolutional neural network (CNN) encoder, pre-trained using Siamese networks. In initial evaluation experiments, we show that our method, combining scoring structure of the kinematic and visual models within a MHT framework, has improved performance especially in edge cases where entity motion is unpredictable, or the data presents frames with significant gaps.
更多查看译文
关键词
multi-object tracking, deep neural network, extended kalman filter, long short-term memory network, multiple hypothesis tracking
AI 理解论文
溯源树
样例
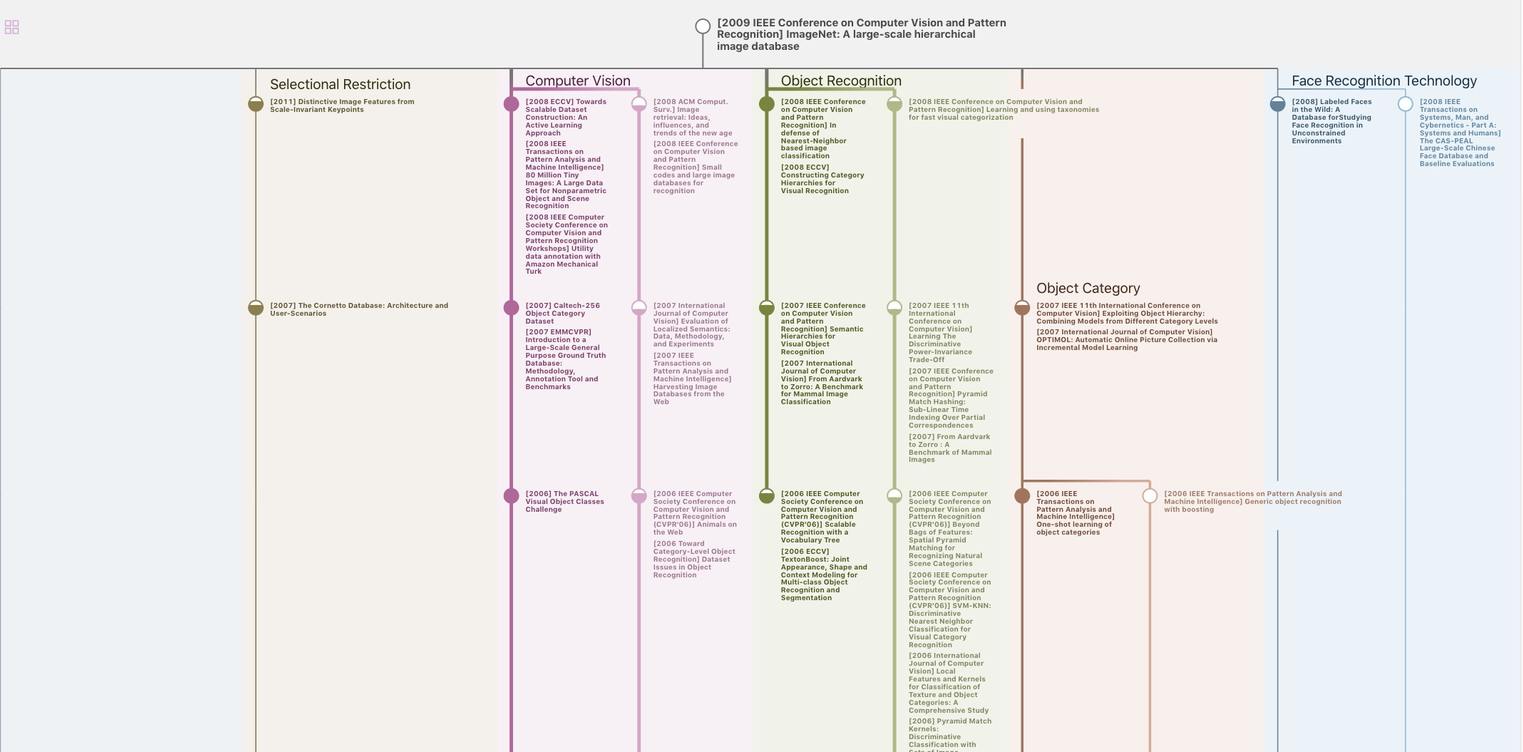
生成溯源树,研究论文发展脉络
Chat Paper
正在生成论文摘要