Long-tailed Distribution Adaptation
PROCEEDINGS OF THE 29TH ACM INTERNATIONAL CONFERENCE ON MULTIMEDIA, MM 2021(2021)
摘要
Recognizing images with long-tailed distributions remains a challenging problem while there lacks an interpretable mechanism to solve this problem. In this study, we formulate Long-tailed recognition as Domain Adaption (LDA), by modeling the long-tailed distribution as an unbalanced domain and the general distribution as a balanced domain. Within the balanced domain, we propose to slack the generalization error bound, which is defined upon the empirical risks of unbalanced and balanced domains and the divergence between them. We propose to jointly optimize empirical risks of the unbalanced and balanced domains and approximate their domain divergence by intra-class and inter-class distances, with the aim to adapt models trained on the long-tailed distribution to general distributions in an interpretable way. Experiments on benchmark datasets for image recognition, object detection, and instance segmentation validate that our LDA approach, beyond its interpretability, achieves state-of-the-art performance.
更多查看译文
关键词
long-tail distribution,domain adaptation,classification,object detection,instance segmentation
AI 理解论文
溯源树
样例
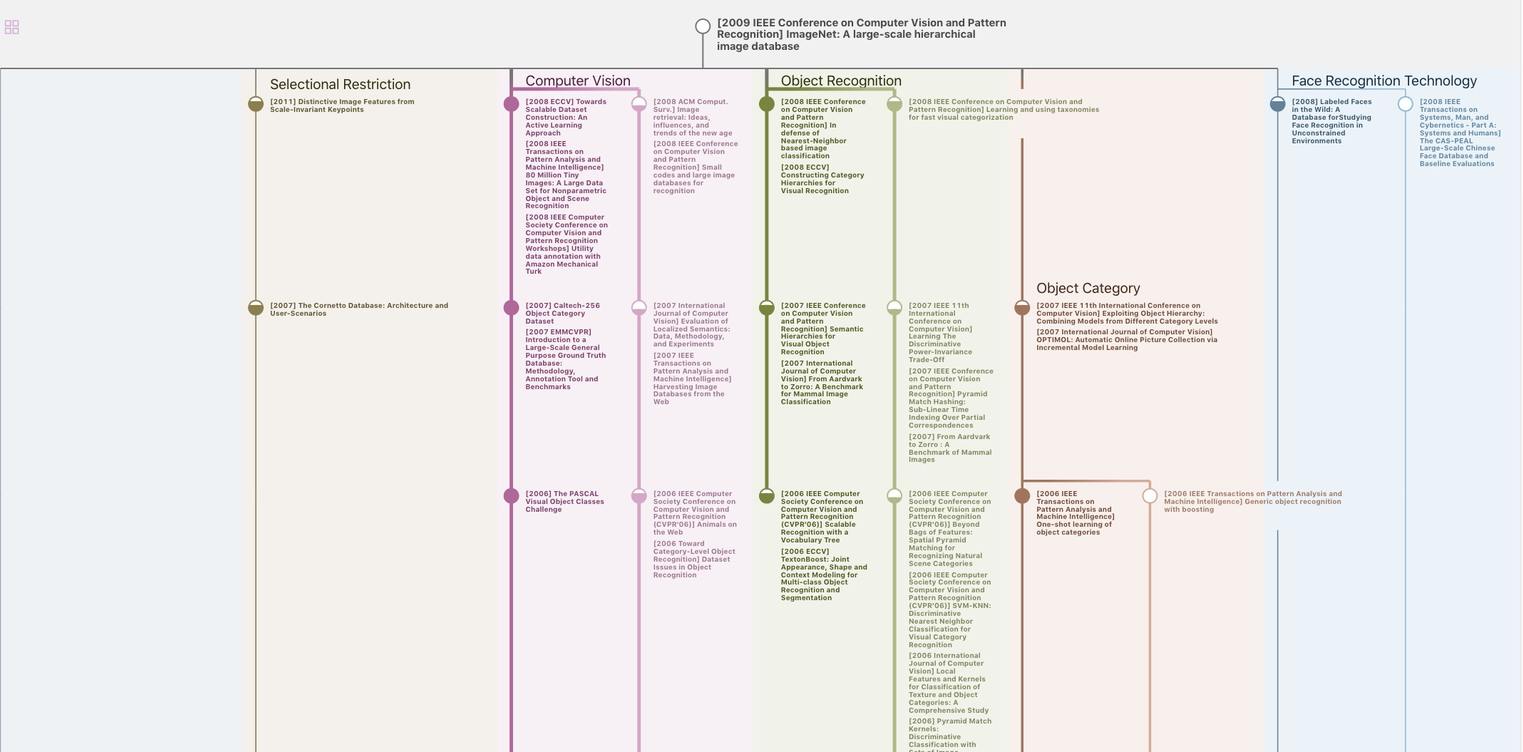
生成溯源树,研究论文发展脉络
Chat Paper
正在生成论文摘要