Recovering SWI-filtered phase data using deep learning
MAGNETIC RESONANCE IN MEDICINE(2022)
摘要
Purpose To develop a deep neural network to recover filtered phase from clinical MR phase images to enable the computation of QSMs. Methods Eighteen deep learning networks were trained to recover combinations of 13 SWI phase-filtering pipelines. SWI-filtered data were computed offline from five multiorientation, multiecho MRI scans yielding 132 3D volumes (118/7/7 training/validation/testing). Two experiments were conducted to show the efficacy of the networks. First, using QSM processing, local fields were computed from the raw phase and subsequently filtered using the SWI-filtering pipelines. The networks were then trained to invert the filtering operation. Second, the trained networks were fine-tuned to recover unfiltered local fields from filtered local fields computed by applying QSM processing to the SWI-filtered phase. Susceptibility maps were computed from the recovered fields and compared with gold standard multiple orientation sampling reconstructions. Results Susceptibility maps computed from the raw phase using standard QSM processing have a normalized root mean square error (NRMSE) of 0.732 +/- 0.095. Susceptibility maps computed from the recovered phase obtained NRMSEs of 0.725 +/- 0.095. The network trained using all 13 processing methods generalized well, obtaining NRMSEs of 0.725 +/- 0.89 on filters it has not seen, while matching the reconstruction accuracy of networks trained to recover a single filter. Conclusion It is feasible to recover SWI-filtered phase using deep learning. QSM can be computed from the recovered phase from SWI acquisition with comparable accuracy to standard QSM processing.
更多查看译文
关键词
deep learning, homodyne, magnetic susceptibility, QSM, recovering phase, SWI
AI 理解论文
溯源树
样例
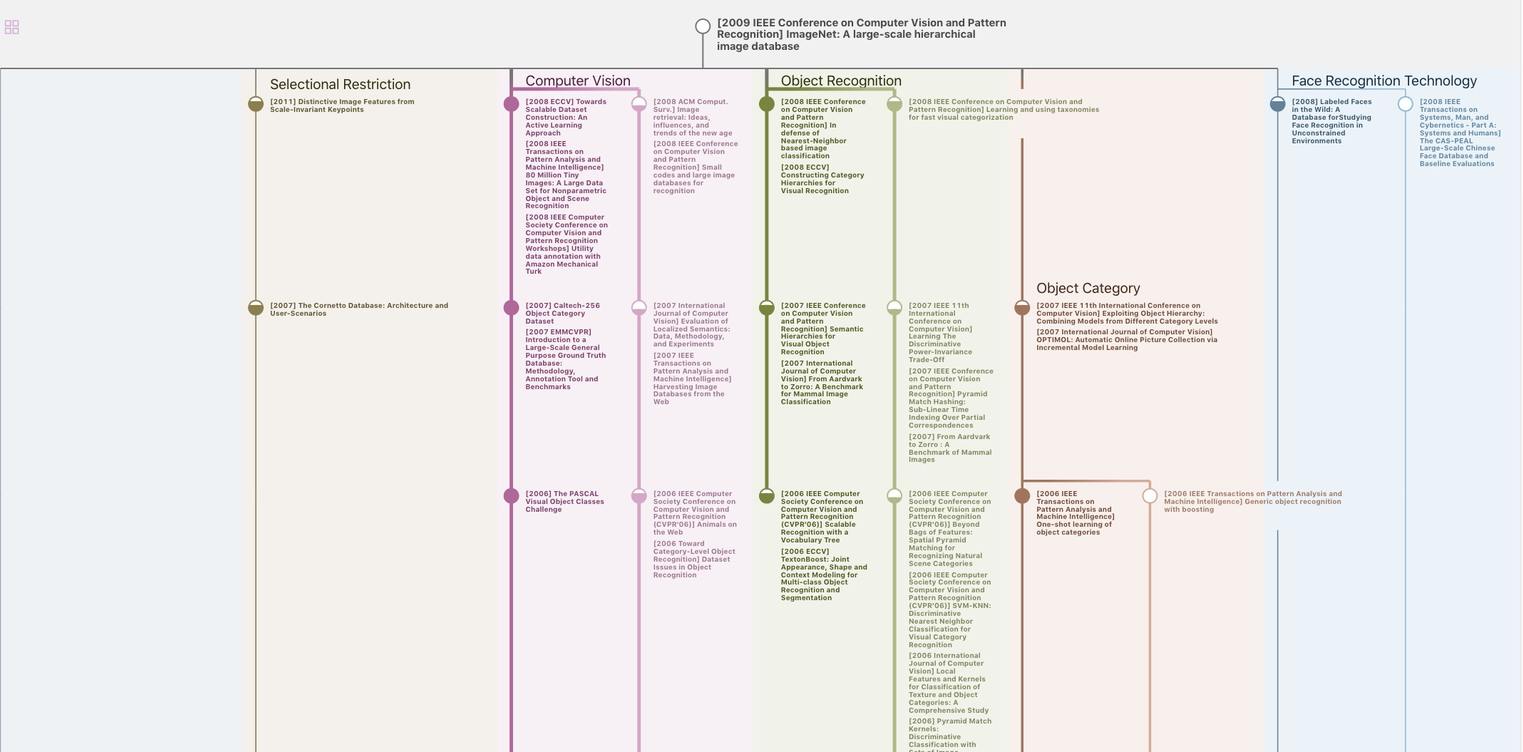
生成溯源树,研究论文发展脉络
Chat Paper
正在生成论文摘要