Distribution-free and Model-Free Multivariate Feature Screening Via Multivariate Rank Distance Correlation
Journal of Multivariate Analysis/Journal of multivariate analysis(2022)
摘要
Feature screening approaches are effective in selecting active features from data with ultrahigh dimensionality and increasing complexity; however, the majority of existing feature screening approaches are either restricted to a univariate response or rely on some distribution or model assumptions. In this article, we propose a novel sure independence screening approach based on the multivariate rank distance correlation (MrDc-SIS). The MrDc-SIS achieves multiple desirable properties such as being distribution-free, completely nonparametric, scale-free, robust for outliers or heavy tails, and sensitive for hidden structures. Moreover, the MrDc-SIS can be used to screen either univariate or multivariate responses and either one dimensional or multi-dimensional predictors. We establish the asymptotic sure screening consistency property of the MrDc-SIS under a mild condition by lifting previous assumptions about the finite moments. Simulation studies demonstrate that MrDc-SIS outperforms three other closely relevant approaches under various settings. We also apply the MrDc-SIS approach to a multi-omics ovarian carcinoma data downloaded from The Cancer Genome Atlas (TCGA).
更多查看译文
关键词
Distance correlation,Feature screening,Multivariate rank,Sure screening property,Ultrahigh dimensional data analysis
AI 理解论文
溯源树
样例
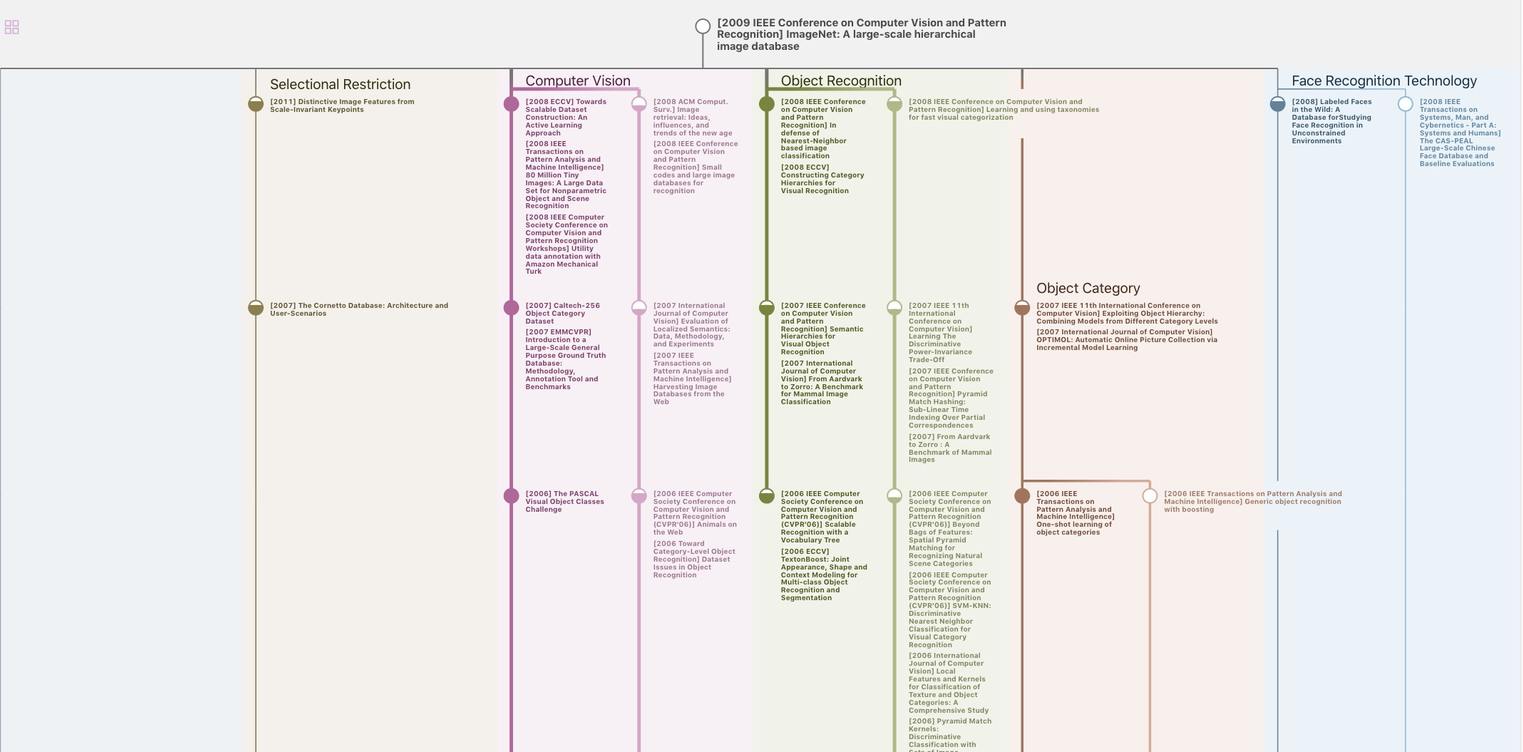
生成溯源树,研究论文发展脉络
Chat Paper
正在生成论文摘要