Online Markov decision processes with non-oblivious strategic adversary
Autonomous Agents and Multi-Agent Systems(2023)
摘要
We study a novel setting in Online Markov Decision Processes (OMDPs) where the loss function is chosen by a non-oblivious strategic adversary who follows a no-external regret algorithm. In this setting, we first demonstrate that MDP-Expert, an existing algorithm that works well with oblivious adversaries can still apply and achieve a policy regret bound of 𝒪(√(T log (L))+τ ^2√( T log (| A | ))) where L is the size of adversary’s pure strategy set and | A | denotes the size of agent’s action space.Considering real-world games where the support size of a NE is small, we further propose a new algorithm: MDP-Online Oracle Expert (MDP-OOE), that achieves a policy regret bound of 𝒪(√(Tlog (L))+τ ^2√( T k log (k))) where k depends only on the support size of the NE. MDP-OOE leverages the key benefit of Double Oracle in game theory and thus can solve games with prohibitively large action space. Finally, to better understand the learning dynamics of no-regret methods, under the same setting of no-external regret adversary in OMDPs, we introduce an algorithm that achieves last-round convergence to a NE result. To our best knowledge, this is the first work leading to the last iteration result in OMDPs.
更多查看译文
关键词
Multi-agent system, Game theory, Online learning, Online Markov decision processes, Non-oblivious adversary, Last round convergence
AI 理解论文
溯源树
样例
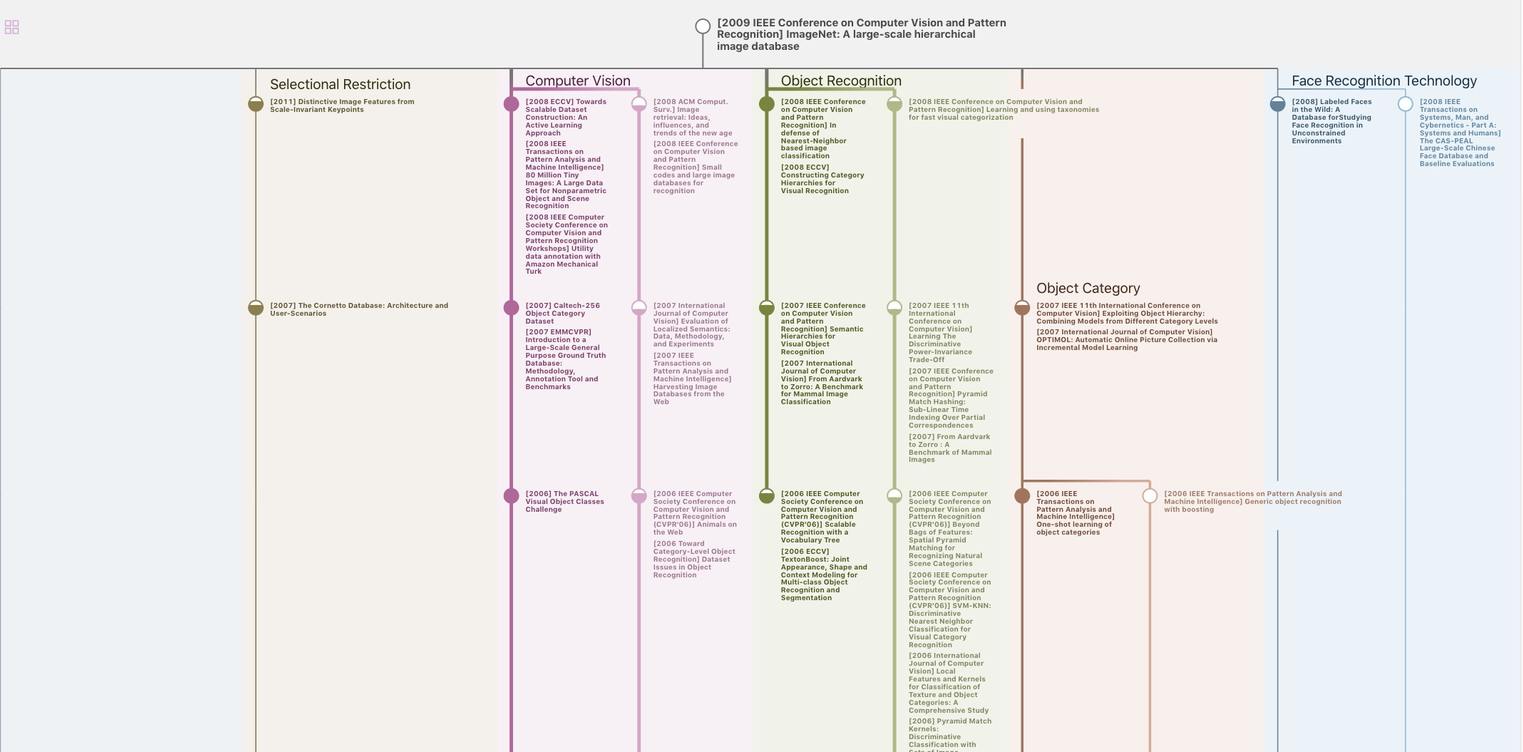
生成溯源树,研究论文发展脉络
Chat Paper
正在生成论文摘要