Development of a proteochemometric-based support vector machine model for predicting bioactive molecules of tubulin receptors
Molecular Diversity(2021)
摘要
Microtubules are receiving enormous interest in drug discovery due to the important roles they play in cellular functions. Targeting tubulin polymerization presents an excellent opportunity for the development of anti-tubulin drugs. Drug resistance and high toxicity of currently used tubulin-binding agents have necessitated the pursuit of novel drug candidates with increased therapeutic potency. The design of novel drug candidates can be achieved using efficient computational techniques to support existing efforts. Proteochemometric (PCM) modeling is a computational technique that can be employed to elucidate the bioactivity relations between related targets and multiple ligands. We have developed a PCM-based Support Vector Machine (SVM) approach for predicting the bioactivity between tubulin receptors and small, drug-like molecules. The bioactivity datasets used for training the SVM algorithm were obtained from the Binding DB database. The SVM-based PCM model yielded a good overall predictive performance with an area under the curve (AUC) of 87%, Matthews correlation coefficient (MCC) of 72%, overall accuracy of 93%, and a classification error of 7%. The algorithm allows the prediction of the likelihood of new interactions based on confidence scores between the query datasets, comprising ligands in SMILES format and protein sequences of tubulin targets. The algorithm has been implemented as a web server known as TubPred, accessible via http://35.167.90.225:5000/ .
更多查看译文
关键词
Proteochemometric, Support vector machine, Tubulin, Bioactivity, Machine learning
AI 理解论文
溯源树
样例
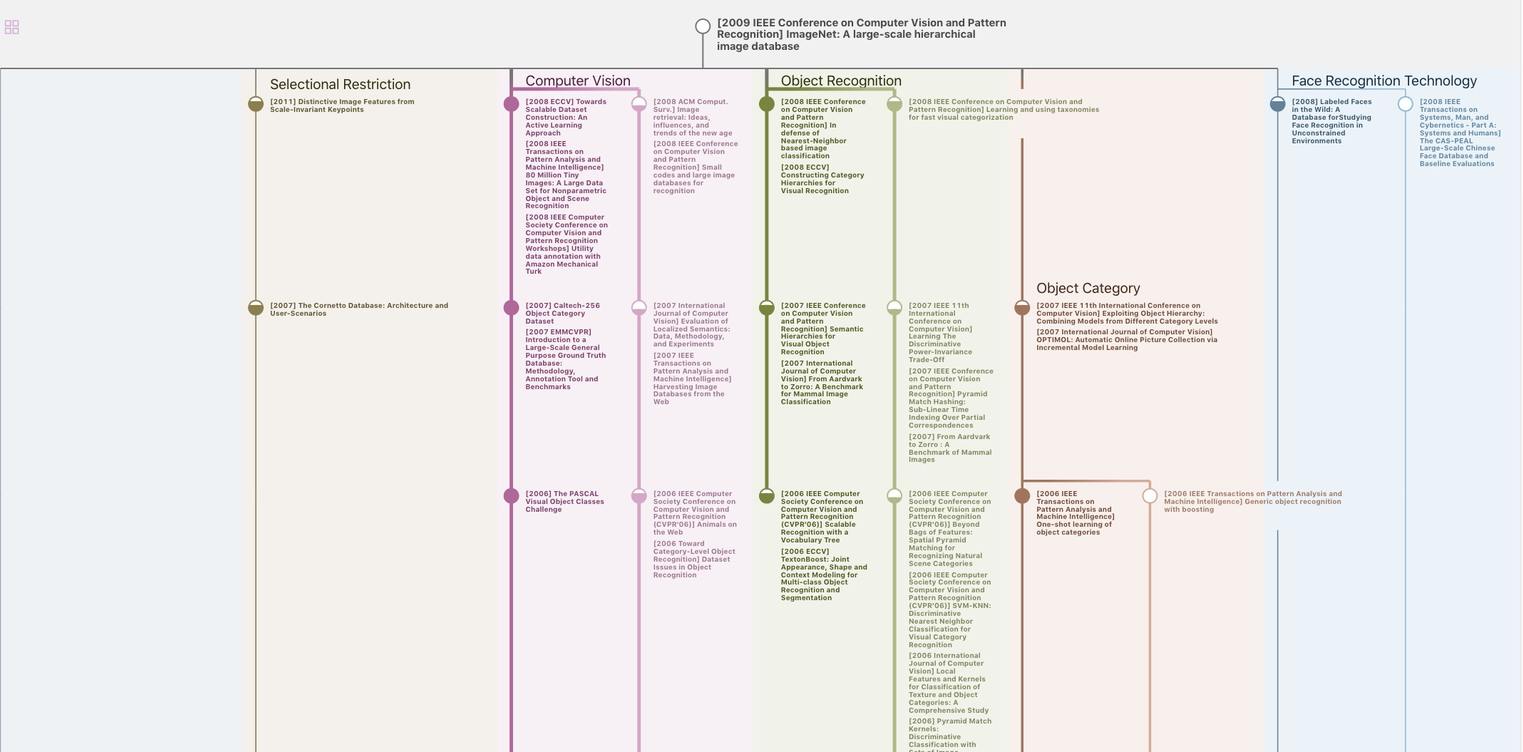
生成溯源树,研究论文发展脉络
Chat Paper
正在生成论文摘要