Learning graph attention-aware knowledge graph embedding
Neurocomputing(2021)
摘要
The knowledge graph, which utilizes graph structure to represent multi-relational data, has been widely used in the reasoning and prediction tasks, attracting considerable research efforts recently. However, most existing works still concentrate on learning knowledge graph embeddings straightforwardly and intuitively without subtly considering the context of knowledge. Specifically, recent models deal with each single triple independently or consider contexts indiscriminately, which is one-sided as each knowledge unit (i.e., triple) can be derived from its partial surrounding triples. In this paper, we propose a graph-attention-based model to encode entities, which formulates a knowledge graph as an irregular graph and explores a number of concrete and interpretable knowledge compositions by integrating the graph-structured information via multiple independent channels. To measure the correlation between entities from different angles (i.e., entity pair, relation, and structure), we respectively develop three attention metrics. By making use of our enhanced entity embeddings, we further introduce several improved factorization functions for updating relation embeddings and evaluating candidate triples. We conduct extensive experiments on downstream tasks including entity classification, entity typing, and link prediction to validate our methods. Empirical results validate the importance of our introduced attention metrics and demonstrate that our proposed method can improve the performance of factorization models on large-scale knowledge graphs.
更多查看译文
关键词
Knowledge graph embedding,Graph attention mechanism,Entity typing,Link prediction
AI 理解论文
溯源树
样例
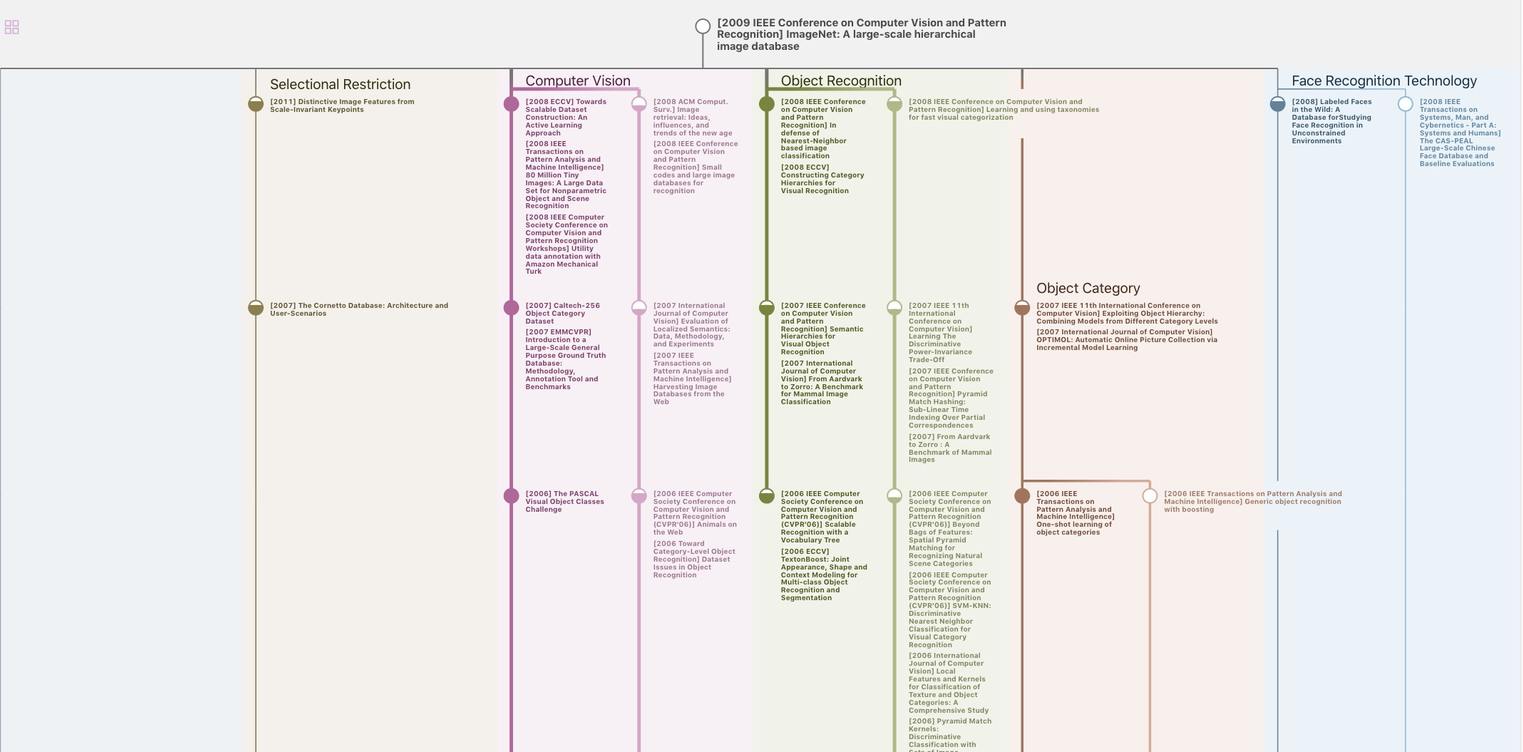
生成溯源树,研究论文发展脉络
Chat Paper
正在生成论文摘要