FLTRL: A Fuzzy-Logic Transfer Learning Powered Reinforcement Learning Method for Intelligent Online Control in Power Systems
EXPLAINABLE AI AND OTHER APPLICATIONS OF FUZZY TECHNIQUES, NAFIPS 2021(2022)
摘要
Automatic control protocols of power systems are of utmost importance in providing reliability and stability to the power delivery, robust demand response, and frequency stability. Due to the advances on computing and sensing, smart data-driven techniques have provided promising solutions in different applications of control systems. However, there still remain challenges for applying data-driven methods to automatic control of power systems. One of the main challenges is that the training procedure of the data-driven methods may need extensive time that is not practical for online control of power systems. To address this issue, in this paper, we employ a fuzzy-logic transfer learning-powered (FLTRL) model to realize an intelligent online control in power systems. The FLTRL model is proposed to ensure power balance and frequency stability in the power system control. To achieve this goal, in our proposed FLTRL model, we exploit fuzzy logic and transfer learning techniques to formulate the correlation between the previous experiences in power system control and the current contingency. The correlation will be seamlessly integrated with deep deterministic policy gradient (DDPG) reinforcement learning (RL) method, which automatically optimizes the decision making on the controlling actions for the power system from the perspective of timely response and effectiveness. In the simulation section, we evaluate the performance of our proposed method by testing it on a customized IEEE 9-bus system.
更多查看译文
关键词
intelligent online control,reinforcement learning,powered systems,fuzzy-logic
AI 理解论文
溯源树
样例
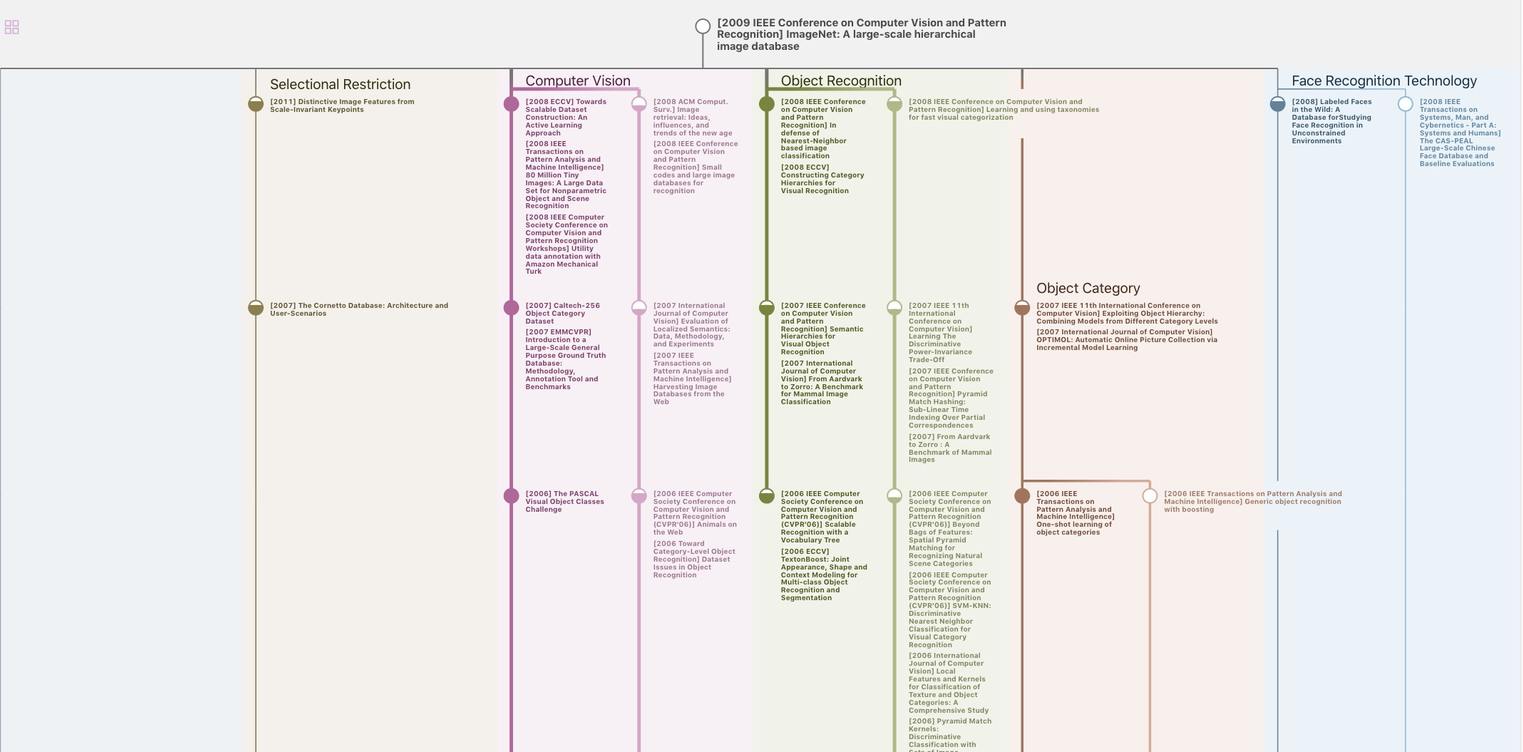
生成溯源树,研究论文发展脉络
Chat Paper
正在生成论文摘要