ComputeCOVID19+ - Accelerating COVID-19 Diagnosis and Monitoring via High-Performance Deep Learning on CT Images.
ICPP(2021)
摘要
The COVID-19 pandemic has highlighted the importance of diagnosis and monitoring as early and accurately as possible. However, the reverse-transcription polymerase chain reaction (RT-PCR) test results in two issues: (1) protracted turnaround time from sample collection to testing result and (2) compromised test accuracy, as low as 67%, due to when and how the samples are collected, packaged, and delivered to the lab to conduct the RT-PCR test. Thus, we present ComputeCOVID19+, our computed tomography-based framework to improve the testing speed and accuracy of COVID-19 (plus its variants) via a deep learning-based network for CT image enhancement called DDnet, short for DenseNet and Deconvolution network. To demonstrate its speed and accuracy, we evaluate ComputeCOVID19+ across several sources of computed tomography (CT) images and on many heterogeneous platforms, including multicore CPU, many-core GPU, and even FPGA. Our results show that ComputeCOVID19+ can significantly shorten the turnaround time from days to minutes and improve the testing accuracy to 91%.
更多查看译文
关键词
AI, biomedical imaging, COVID-19, computed tomography, coronavirus, deep learning, FPGA, GPU, neural network
AI 理解论文
溯源树
样例
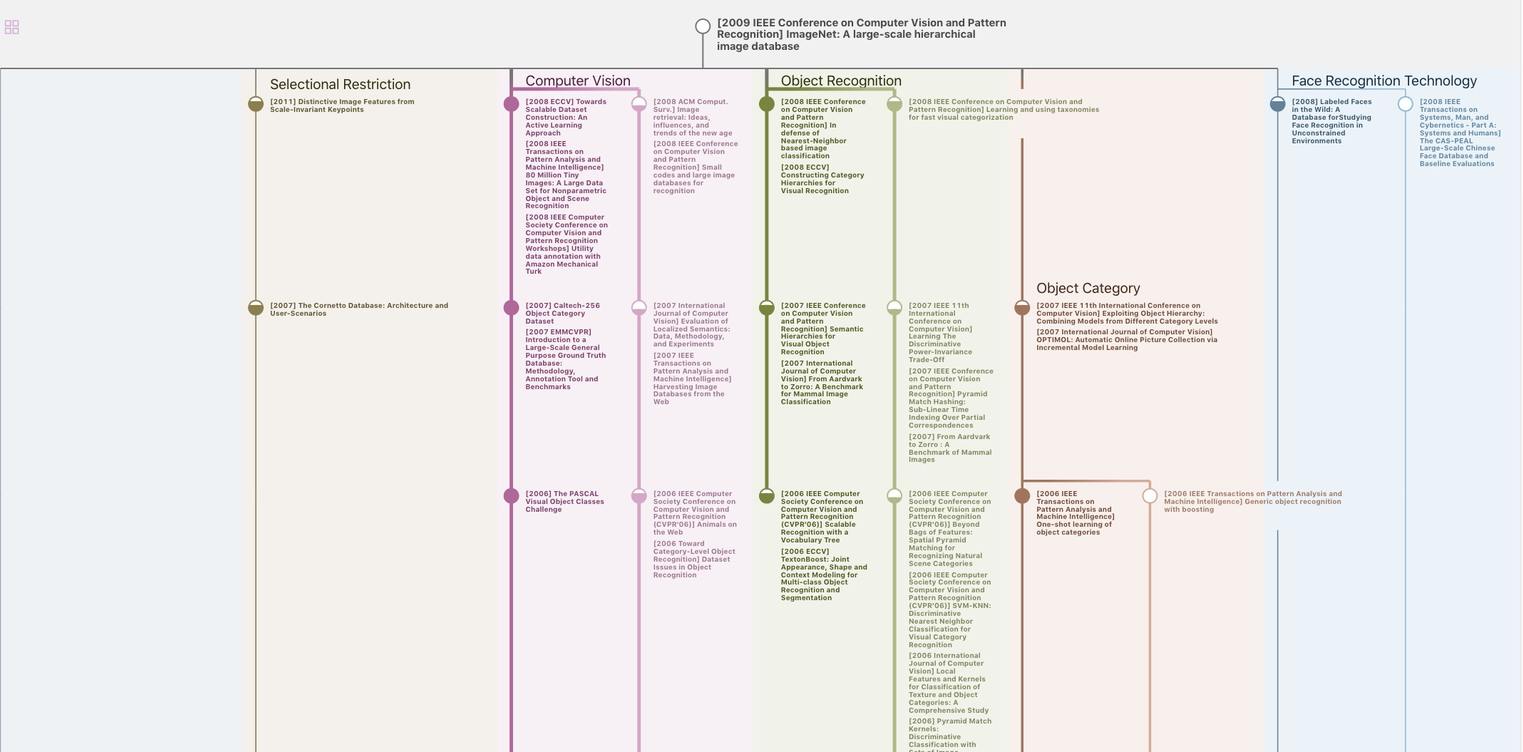
生成溯源树,研究论文发展脉络
Chat Paper
正在生成论文摘要