Ascetic - Enhancing Cross-Iterations Data Efficiency in Out-of-Memory Graph Processing on GPUs.
ICPP(2021)
摘要
Graph analytics are widely used in real-world applications, and GPUs are major accelerators for such applications. However, as graph sizes become significantly larger than the capacity of GPU memory, the performance can degrade significantly due to the heavy overhead required in moving a large amount of graph data between CPU main memory and GPU memory. Some existing approaches have tried to exploit data locality and addressed the issues of memory oversubscription on GPUs. However, these approaches have yet to take advantage of the data reuse cross iterations because of the data sizes in most large-graph analytics. In our studies, we have found that in most graph applications the graph traversals exhibit a roughly sequential scan over the graph data with an extremely large memory footprint. Based on the observation, we propose a novel framework, called Ascetic, to exploit temporal locality with very long reuse distances. In Ascetic, the GPU memory is divided into a Static Region and an On-demand Region. The static region can exploit data reuse across iterations. The on-demand region is designed to load the data requested in the iteration of the graph traversal while not found in the static region. We have implemented a prototype of the Ascetic framework and conducted a series of experiments on performance evaluation. The experimental results show that Ascetic can significantly reduce the data transfer overhead, and allow more overlapped execution between GPU and CPU, which leads to an average of 2.0x speedup over a state-of-the-art approach.
更多查看译文
关键词
Graph Computing, GPU memory oversubscription, Partitionbased method, Data Reuse
AI 理解论文
溯源树
样例
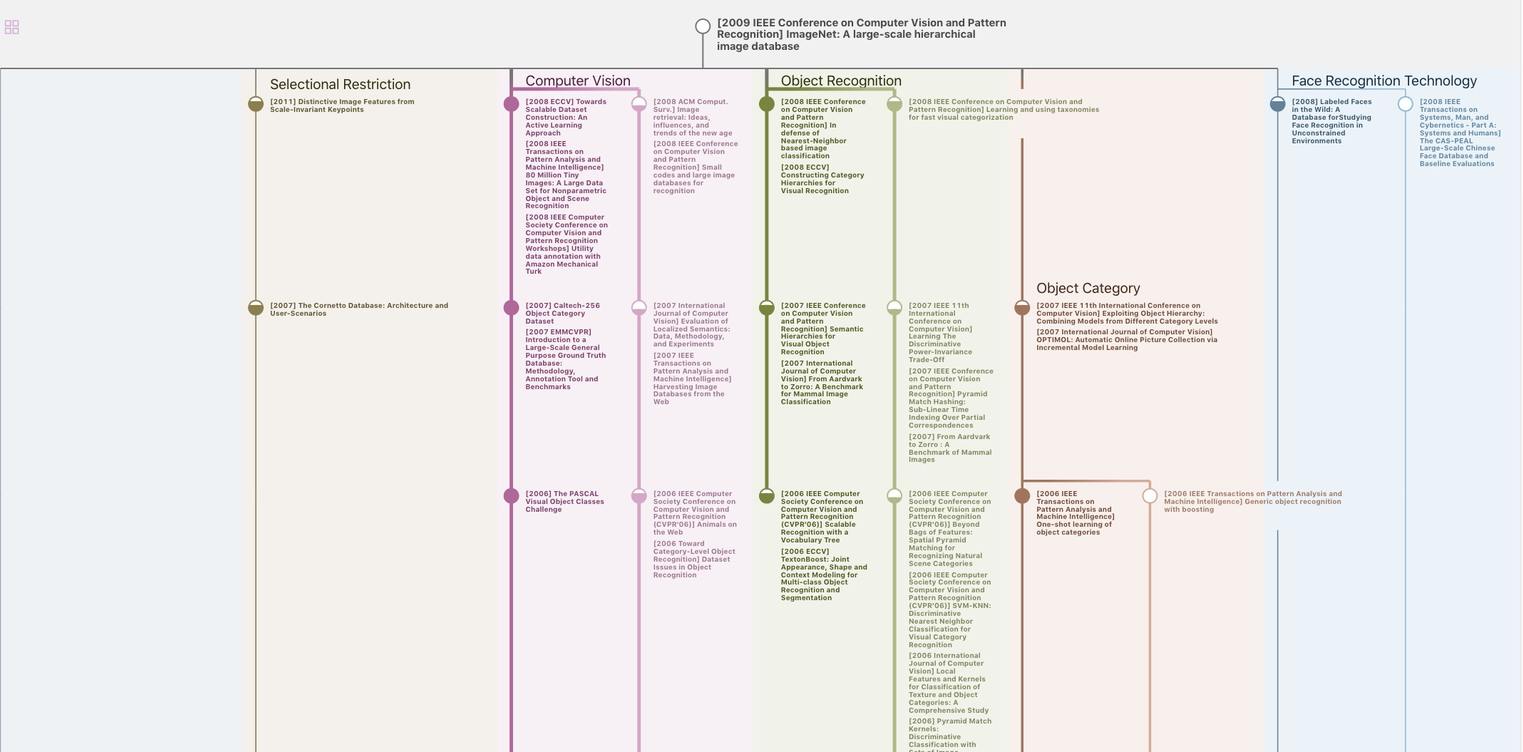
生成溯源树,研究论文发展脉络
Chat Paper
正在生成论文摘要