Supervised Classification with Short-Term Memory of Sleep Stages using Cardio-respiratory and Body Movement Variables.
CASE(2021)
摘要
In the context of the Internet of Things (IoT) healthcare, biophysical features collected during sleep needs robust analysis methods to be efficiently used to detect sleep disorders. In this paper, analysis methods using a limited number of input variables (cardiac, respiratory, and body movement) have been used to perform the classification of sleep stages. The efficiency of each classification method has been compared to a reference method that combines a large number of biophysical features referred to as PolySomnoGraphy (PSG). Five classical machine learning methods were evaluated by testing their accuracy on the same collected data. Finally, using a neural network with a short memory method, the classification task fitted 91.34% of the PSG classification.
更多查看译文
关键词
Sleep stages,machine learning,supervised classification,sleep architecture,polysomnography
AI 理解论文
溯源树
样例
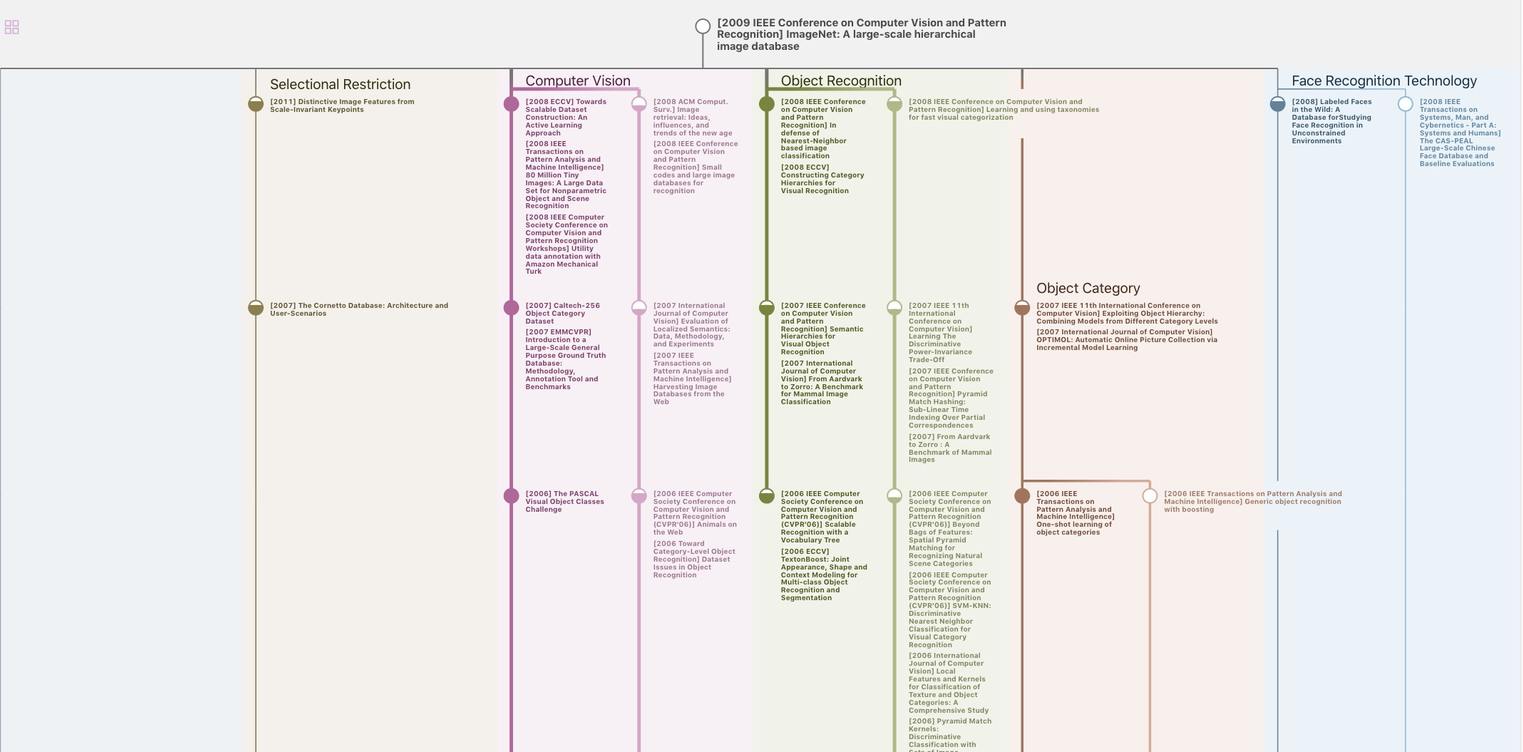
生成溯源树,研究论文发展脉络
Chat Paper
正在生成论文摘要