Adaptive Mechanomyogram Hand Gesture Recognition in Online and Repeatable Environment
CASE(2021)
摘要
We introduce a complete architecture for realtime hand gesture recognition for human-computer interface (HCI) and robotic control. The system addresses ease of use, calibration, and robustness issues which have inhibited gesture recognition wearables in the field. Our system is packaged as a generic (non-customized) arm wearable that integrates: 1) a novel mechanomyogram (MMG) sensing suite; 2) an integrated inertial measurement unit (IMU); 3) accompanying data acquisition and transmission hardware; and 4) real-time signal recognition algorithms to run on the receiving peripheral (e.g. computer, robot, etc.). We implement a rapid training routine capable of grasp pattern identification from small samples (20 per gesture) with less than 5-minute calibration time, which yields immediate real-time accuracies of 84% in amputees (3 gestures) and 89% in non-amputees (5 gestures), with the capacity to scale as users become more comfortable (accurate) with generated gestures. In repeated (5-day) usage with regular donning and doffing of the armband, 89%–91% accuracy is achieved with non-amputees using data over the previous days for reparameterization. Findings demonstrate the capacity to adapt to new able-bodied and amputee subjects with a generic armband and small training datasets, adapt as user proficiency increases, and provide consistent prediction for regular long-term use.
更多查看译文
关键词
hand gesture recognition,mechanomyogram,upper-limb amputee,real-time
AI 理解论文
溯源树
样例
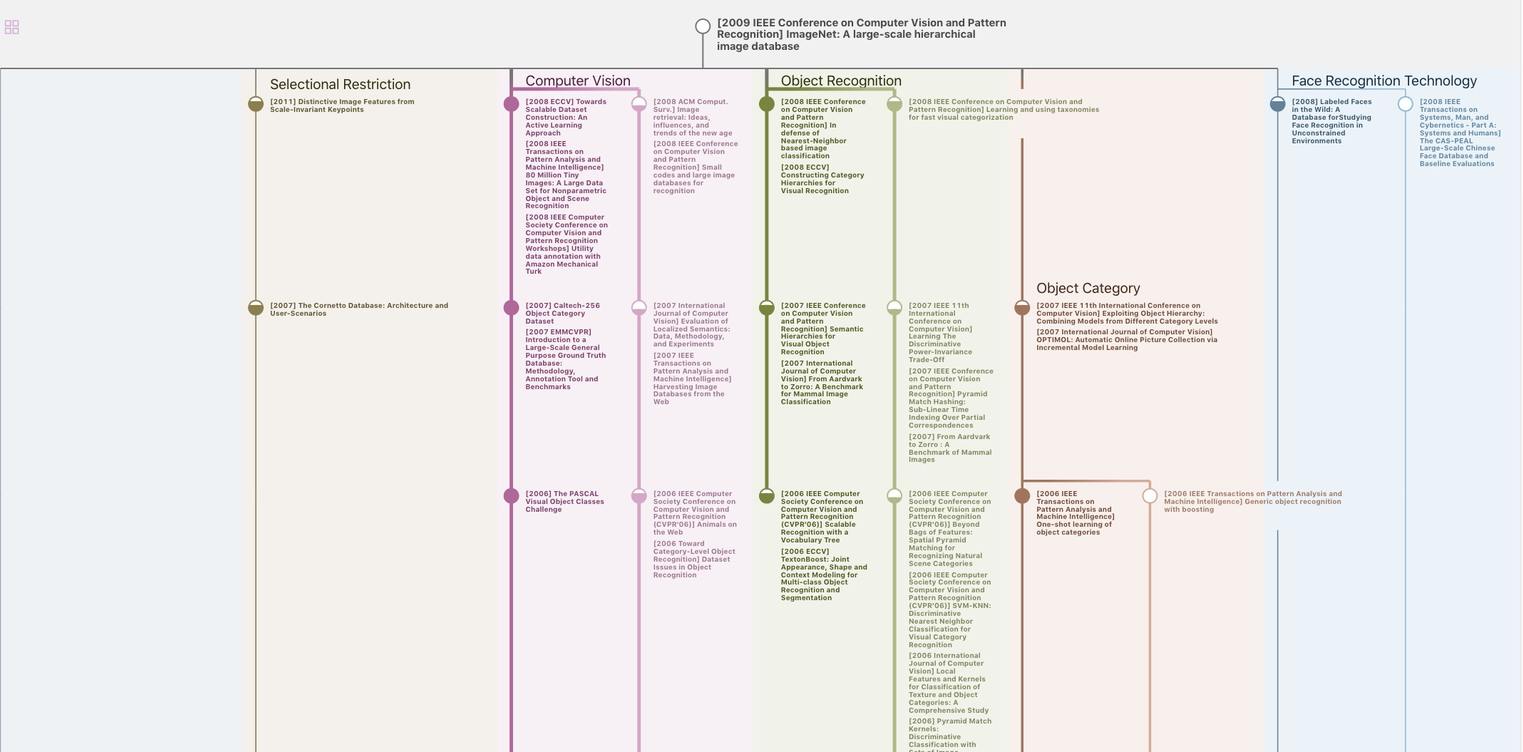
生成溯源树,研究论文发展脉络
Chat Paper
正在生成论文摘要