Robust Automatic Speech Recognition for Call Center Applications
APPLIED COMPUTER SCIENCES IN ENGINEERING, WEA 2021(2021)
摘要
This paper is focused on developing an Automatic Speech Recognition (ASR) system robust against different noisy scenarios. ASR systems are widely used in call centers to convert telephone recordings into text transcriptions which are further used as input to automatically evaluate the Quality of the Service (QoS). Since the evaluation of the QoS and the customer satisfaction is performed by analyzing the text resulting from the ASR system, this process highly depends on the accuracy of the transcription. Given that the calls are usually recorded in non-controlled acoustic conditions, the accuracy of the ASR is typically decreased. To address this problem, we first evaluated four different hybrid architectures: (1) Gaussian Mixture Models (GMM) (baseline), (2) Time Delay Neural Network (TDNN), (3) Long Short-Term Memory (LSTM), and (4) Gated Recurrent Unit (GRU). The evaluation is performed considering a total of 478,6 h of recordings collected in a real call-center. Each recording has its respective transcription and three perceptual labels about the level of noise present during the phone-call: Low level of noise (LN), Medium Level of noise (ML), and High Level of noise (HN). The LSTM-based model achieved the best performance in the MN and HN scenarios with 22, 55% and 27, 99% of word error rate (WER), respectively. Additionally, we implemented a denoiser based on GRUs to enhance the speech signals and the results improved in 1,16% in the HN scenario.
更多查看译文
关键词
ASR, Noise reduction, Speech enhancement, Speech-to-text
AI 理解论文
溯源树
样例
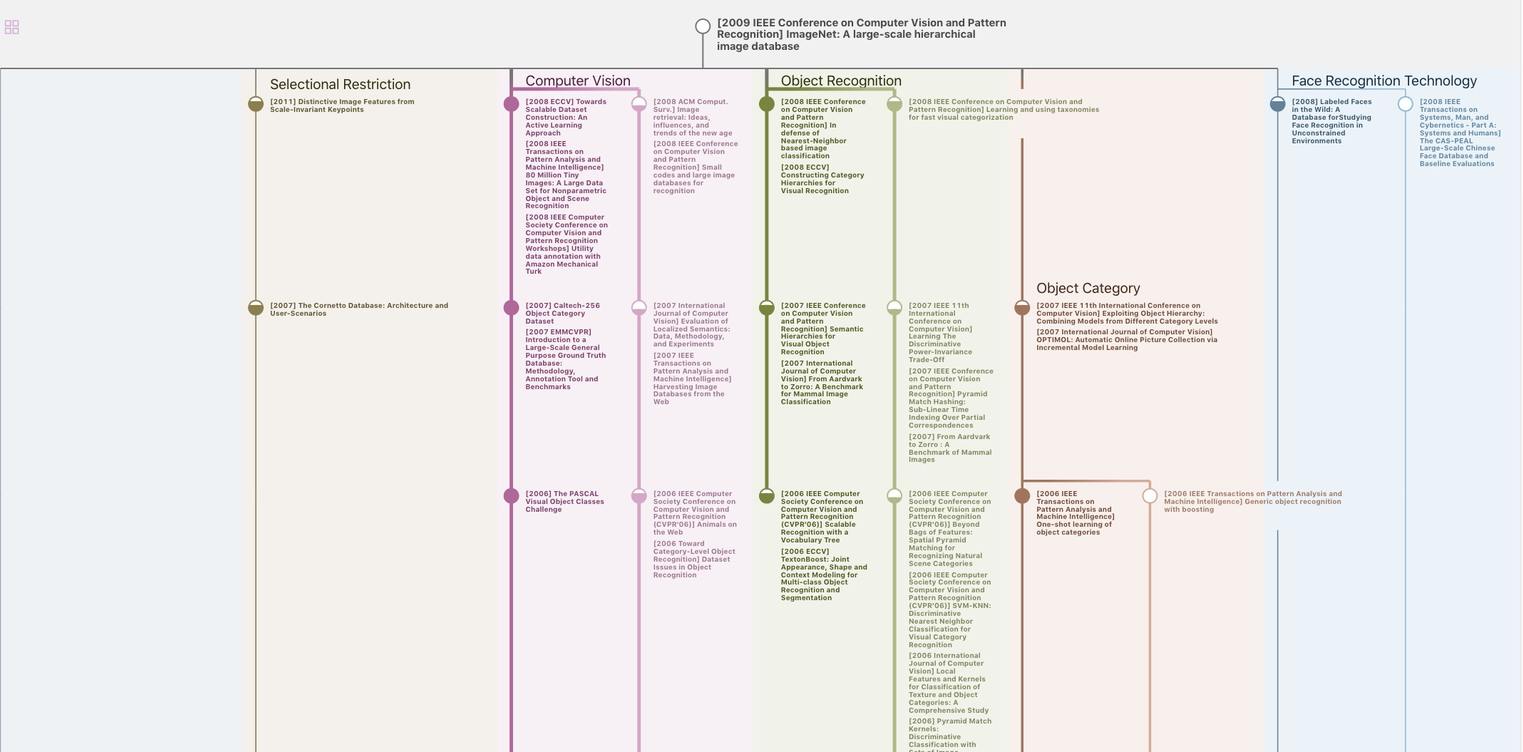
生成溯源树,研究论文发展脉络
Chat Paper
正在生成论文摘要