Multi-Kernel Deformable 3D Convolution for Video Super-Resolution
2021 IEEE INTERNATIONAL SYMPOSIUM ON BROADBAND MULTIMEDIA SYSTEMS AND BROADCASTING (BMSB)(2021)
摘要
Video super-resolution (VSR) methods align and fuse consecutive low-resolution frames to generate high-resolution frames. One of the main difficulties for VSR process is that video contains various motions, and the accuracy of motion estimation greatly affects the quality of video restoration. Diverse receptive fields in temporal and spatial dimensions have the potential to adapt to various motions, which is rarely paid attention in most known VSR methods. In this paper, we propose to provide adaptive receptive fields for VSR model. Firstly, we design a multi-kernel 3D convolution network and integrate it with a multi-kernel deformable convolution network for motion estimation and multiple frames alignment. Secondly, we propose a 2D multi-kernel convolution framework to improve texture restoration quality. Our experimental results show that the proposed framework outperforms the state-of-the-art VSR methods.
更多查看译文
关键词
texture restoration quality,2D multikernel convolution framework,multiple frames alignment,multikernel 3D convolution network,VSR model,adaptive receptive fields,spatial dimensions,temporal dimensions,video restoration,motion estimation,VSR process,high-resolution frames,low-resolution frames,video super-resolution methods,multikernel deformable 3D convolution network
AI 理解论文
溯源树
样例
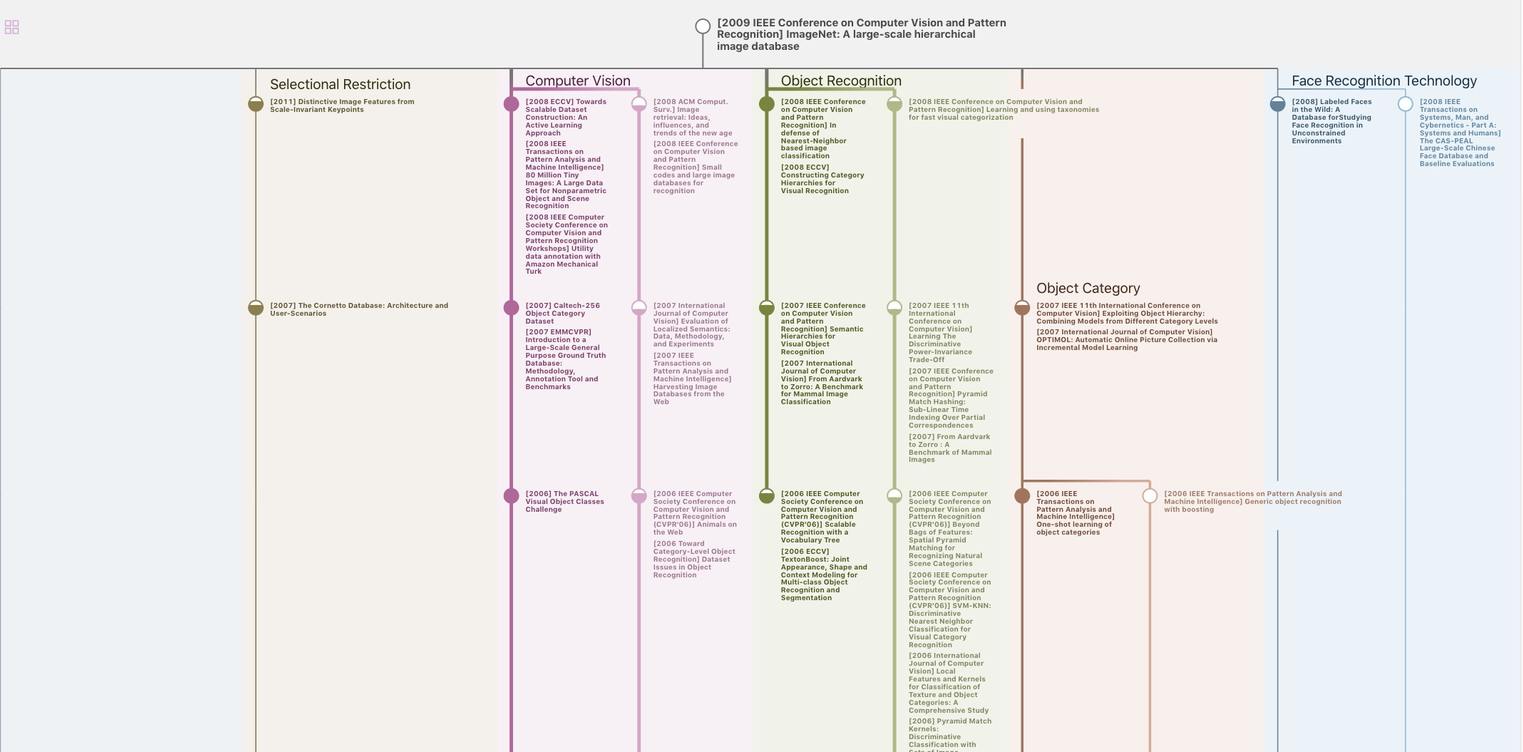
生成溯源树,研究论文发展脉络
Chat Paper
正在生成论文摘要